Learning an Overlap-based Observation Model for 3D LiDAR Localization
IROS(2020)
摘要
Localization is a crucial capability for mobile robots and autonomous cars. In this paper, we address learning an observation model for Monte-Carlo localization using 3D LiDAR data. We propose a novel, neural network-based observation model that computes the expected overlap of two 3D LiDAR scans. The model predicts the overlap and yaw angle offset between the current sensor reading and virtual frames generated from a pre-built map. We integrate this observation model into a Monte-Carlo localization framework and tested it on urban datasets collected with a car in different seasons. The experiments presented in this paper illustrate that our method can reliably localize a vehicle in typical urban environments. We furthermore provide comparisons to a beam-endpoint and a histogram-based method indicating a superior global localization performance of our method with fewer particles.
更多查看译文
关键词
3D LiDAR localization,autonomous cars,3D LiDAR data,neural network-based observation model,expected overlap,3D LiDAR scans,overlap angle,yaw angle,Monte-Carlo localization framework,histogram-based method,superior global localization performance
AI 理解论文
溯源树
样例
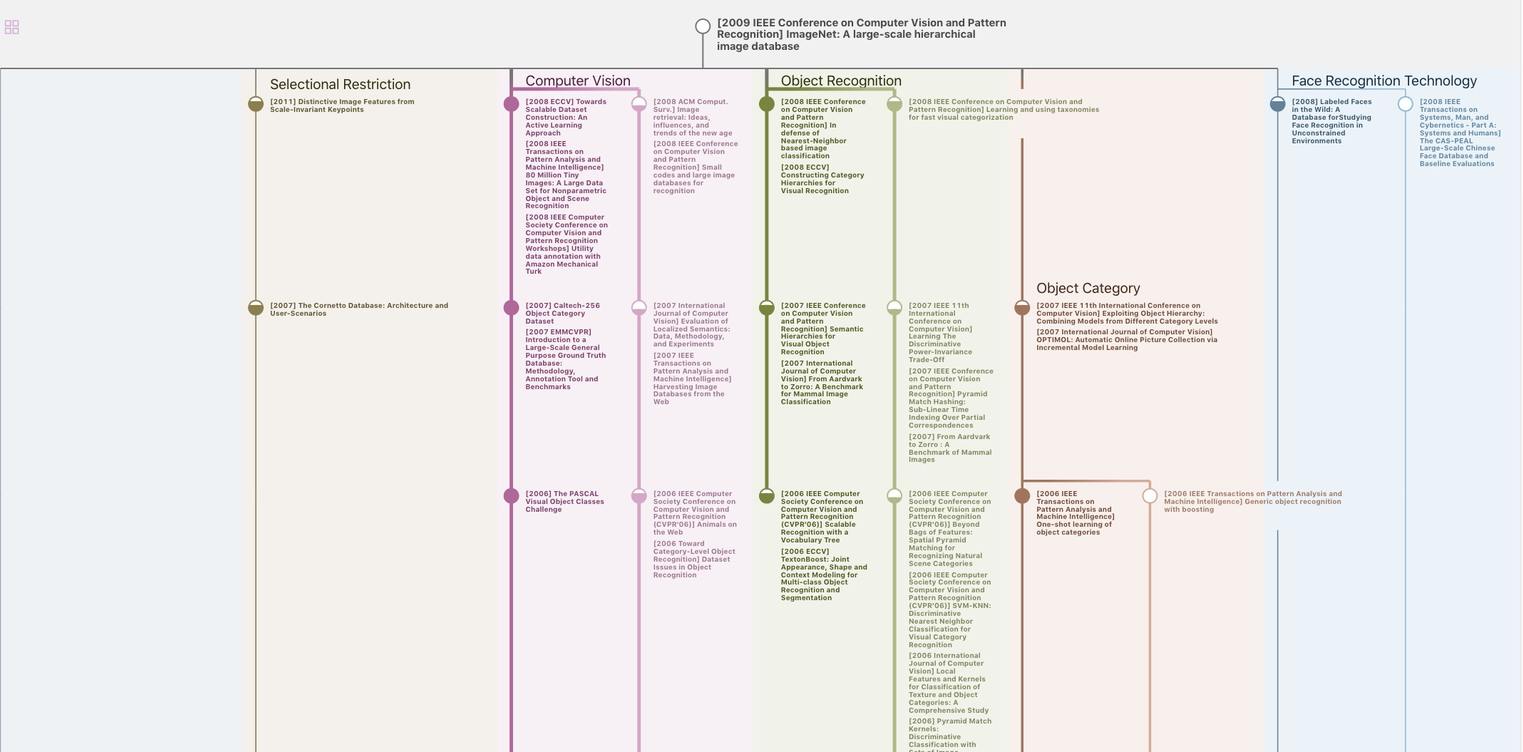
生成溯源树,研究论文发展脉络
Chat Paper
正在生成论文摘要