Robust and Efficient Object Change Detection by Combining Global Semantic Information and Local Geometric Verification.
IROS(2020)
摘要
Identifying new, moved or missing objects is an important capability for robot tasks such as surveillance or maintaining order in homes, offices and industrial settings. However, current approaches do not distinguish between novel objects or simple scene readjustments nor do they sufficiently deal with localization error and sensor noise. To overcome these limitations, we combine the strengths of global and local methods for efficient detection of novel objects in 3D reconstructions of indoor environments. Global structure, determined from 3D semantic information, is exploited to establish object candidates. These are then locally verified by comparing isolated geometry to a reference reconstruction provided by the task. We evaluate our approach on a novel dataset containing different types of rooms with 31 scenes and 260 annotated objects. Experiments show that our proposed approach significantly outperforms baseline methods.
更多查看译文
关键词
global semantic information,local geometric verification,important capability,robot tasks,surveillance,industrial settings,simple scene readjustments,localization error,sensor noise,global methods,local methods,indoor environments,global structure,3D semantic information,object candidates,reference reconstruction,260 annotated objects
AI 理解论文
溯源树
样例
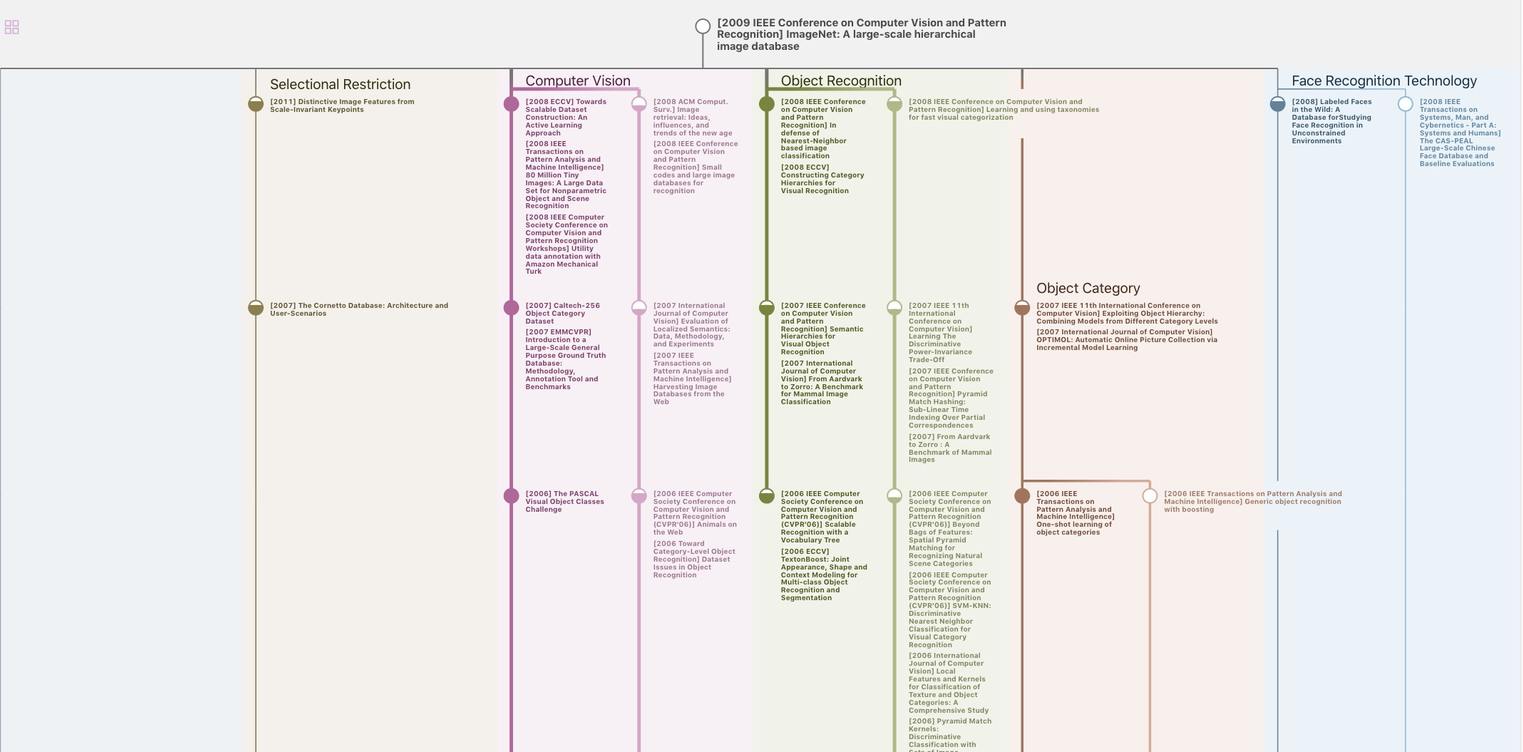
生成溯源树,研究论文发展脉络
Chat Paper
正在生成论文摘要