Tensor Action Spaces for Multi-agent Robot Transfer Learning
IROS(2020)
摘要
We explore using reinforcement learning on single and multi-agent systems such that after learning is finished we can apply a policy zero-shot to new environment sizes, as well as different number of agents and entities. Building off previous work, we show how to map back and forth between the state and action space of a standard Markov Decision Process (MDP) and multi-dimensional tensors such that zero-shot transfer in these cases is possible. Like in previous work, we use a special network architecture designed to work well with the tensor representation, known as the Fully Convolutional Q-Network (FCQN). We show simulation results that this tensor state and action space combined with the FCQN architecture can learn faster than traditional representations in our environments. We also show that the performance of a transferred policy is comparable to the performance of policy trained from scratch in the modified environment sizes and with modified number of agents and entities. We also show that the zero- shot transfer performance across team sizes and environment sizes remains comparable to the performance of training from scratch specific policies in the transferred environments. Finally, we demonstrate that our simulation trained policies can be applied to real robots and real sensor data with comparable performance to our simulation results. Using such policies we can run variable sized teams of robots in a variable sized operating environment with no changes to the policy and no additional learning necessary.
更多查看译文
关键词
tensor action spaces,multiagent robot transfer learning,reinforcement learning,multiagent systems,policy zero-shot,standard Markov decision process,multidimensional tensors,network architecture,tensor representation,tensor state,FCQN architecture,transferred policy,modified environment sizes,team sizes,scratch specific policies,transferred environments,simulation trained policies,variable sized teams,variable sized operating environment,zero-shot transfer performance,fully convolutional Q-network,standard MDP
AI 理解论文
溯源树
样例
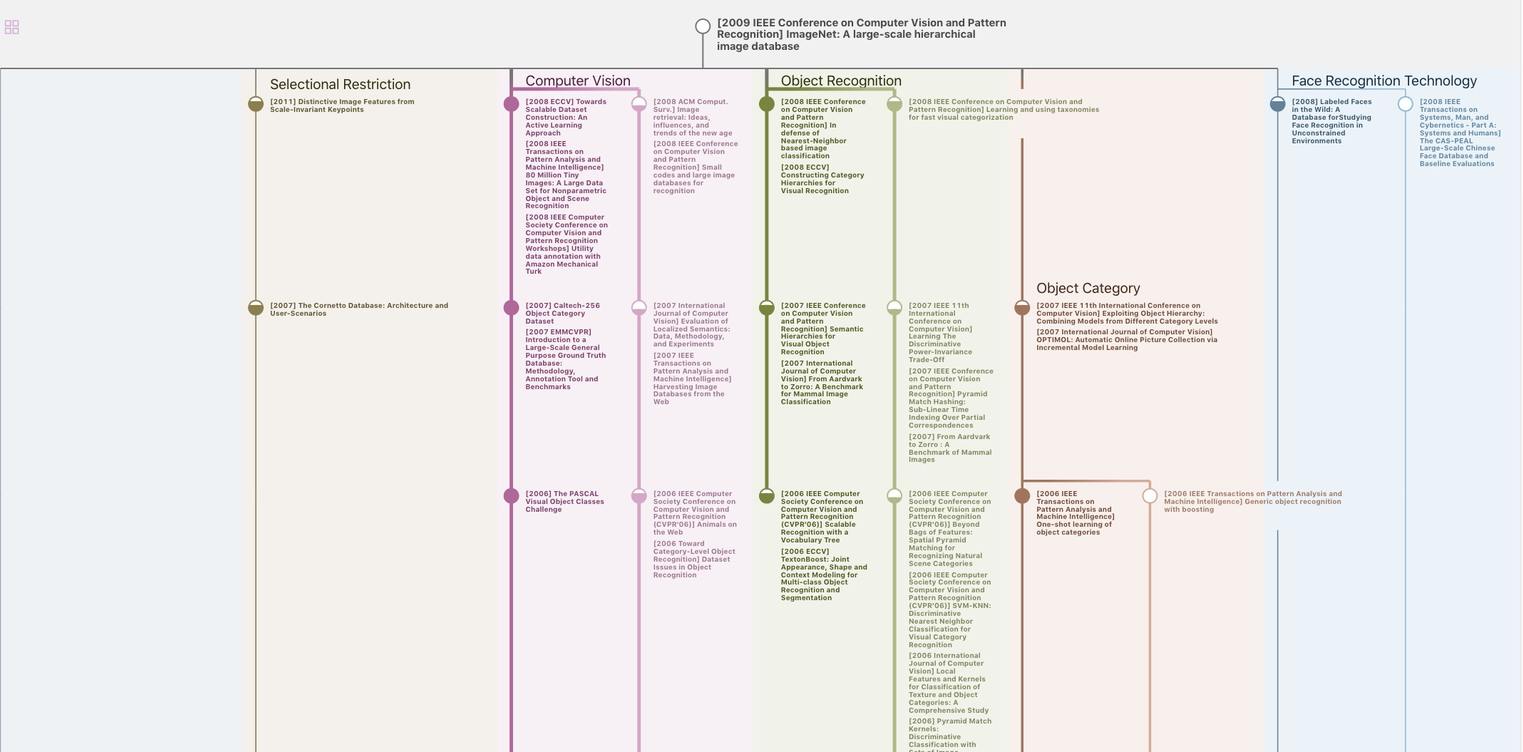
生成溯源树,研究论文发展脉络
Chat Paper
正在生成论文摘要