On the Fundamental Limits of Exact Inference in Structured Prediction
2022 IEEE International Symposium on Information Theory (ISIT)(2022)
摘要
Inference in structured prediction is naturally modeled with a graph, where the goal is to recover the unknown true label for each node given noisy observations corresponding to nodes and edges. The focus of this paper is on the fundamental limits of exact recovery irrespective of computational efficiency, assuming the generative process proposed by [1]. Analyzing the fundamental limits is crucial for algorithm evaluation and development. In this regard, we establish the information-theoretic limit bounds and show that there exists a gap between the limits and the performance of the existent tractable method [2], implying the need for further development of algorithms for exact inference. The fundamental limit we suggest applies to general connected graphs and involves graphical metrics such as the Cheeger constant and the maximum degree. Finally, we reveal that the sufficient and necessary conditions derived from the limit bounds are tight up to a logarithmic factor for a wide range of graphs.
更多查看译文
关键词
structured prediction,exact inference,fundamental limits
AI 理解论文
溯源树
样例
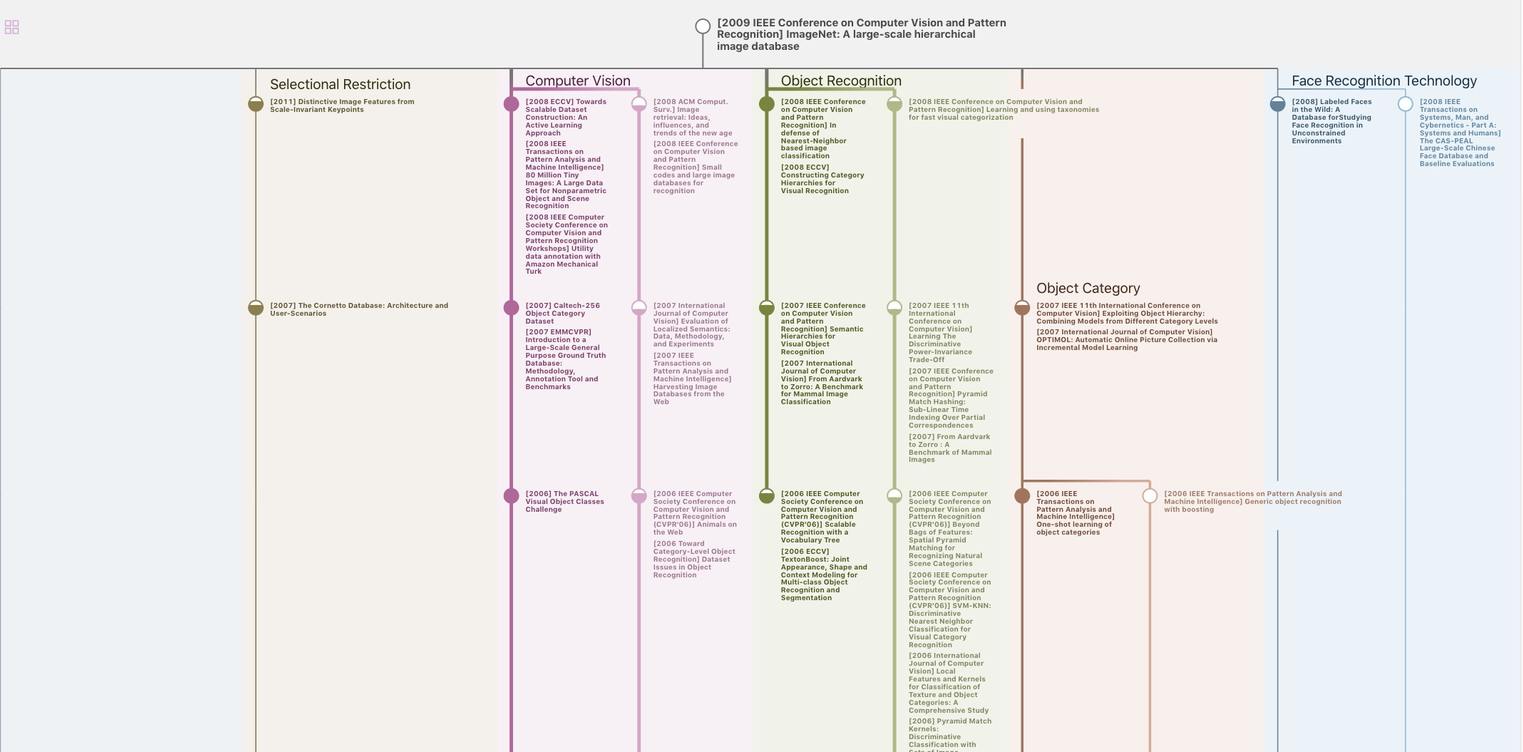
生成溯源树,研究论文发展脉络
Chat Paper
正在生成论文摘要