Toward a mathematical theory of trajectory inference
ANNALS OF APPLIED PROBABILITY(2024)
摘要
We devise a theoretical framework and a numerical method to infer trajectories of a stochastic process from samples of its temporal marginals. This problem arises in the analysis of single -cell RNA-sequencing data, which provide high-dimensional measurements of cell states but cannot track the trajectories of the cells over time. We prove that for a class of stochastic processes it is possible to recover the ground truth trajectories from limited samples of the temporal marginals at each time-point, and provide an efficient algorithm to do so in practice. The method we develop, Global Waddington-OT (gWOT), boils down to a smooth convex optimization problem posed globally over all time-points involving entropy-regularized optimal transport. We demonstrate that this problem can be solved efficiently in practice and yields good reconstructions, as we show on several synthetic and real data sets.
更多查看译文
关键词
Trajectory inference,stochastic processes,convex optimization,optimal transport,developmental biology,single-cell RNA-sequencing
AI 理解论文
溯源树
样例
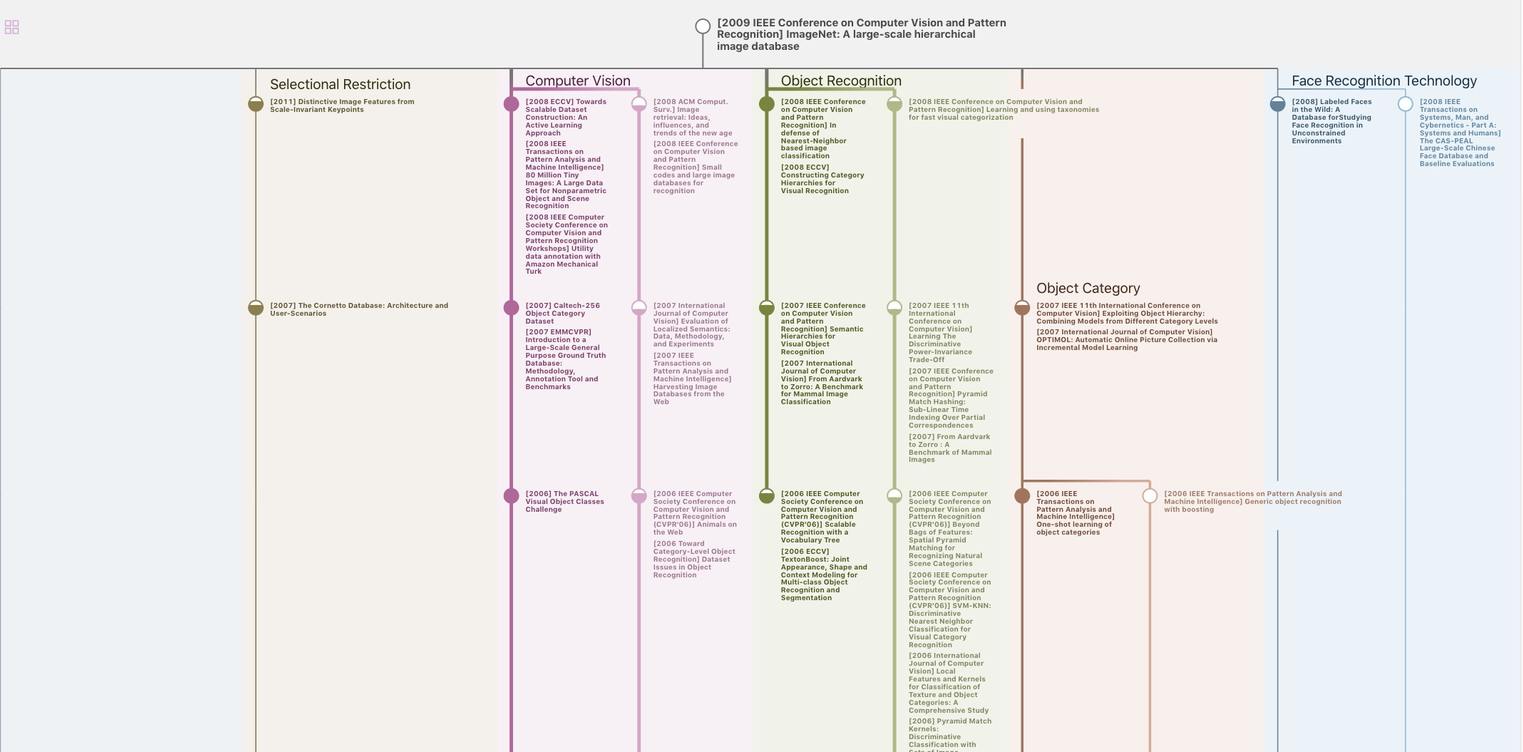
生成溯源树,研究论文发展脉络
Chat Paper
正在生成论文摘要