Exploiting Inconsistency Problem In Multi-Label Classification Via Metric Learning
20TH IEEE INTERNATIONAL CONFERENCE ON DATA MINING (ICDM 2020)(2020)
摘要
Multi-label classification problem has fined growing attention in recent years due to its diverse applications to real-world problems such as image annotation and query suggestions. However, traditional multi-label classification methods tend to fail due to the inconsistency between input and output space, where similar instances in the feature space may have distinct semantic labels in the output space. To eliminate the inconsistency problem, in this paper, we propose a supervised metric learning approach for multi-label classification, called MLMLI, which attempts to learn a similarity metric for multi-label data. The basic idea is to incorporate label similarity in output space as weak supervision to assign higher similarity to the pairs of instances with more similar labels. To this end, a weighted triple loss, and a step-specified coordinate descent method are employed. Different from traditional dimensionality reduction approaches, MLMLI is independent of any prior information of data, and thus enjoys a high capacity of generalization. Moreover, the metric learned by MLMLI offers a new venue for feature learning. Experiments on real-world datasets have further demonstrated the effectiveness of MLMLI and show its superiority over many state-of-the-art algorithms.
更多查看译文
关键词
Multi-label learning, Metric learning, Classification, Feature learning
AI 理解论文
溯源树
样例
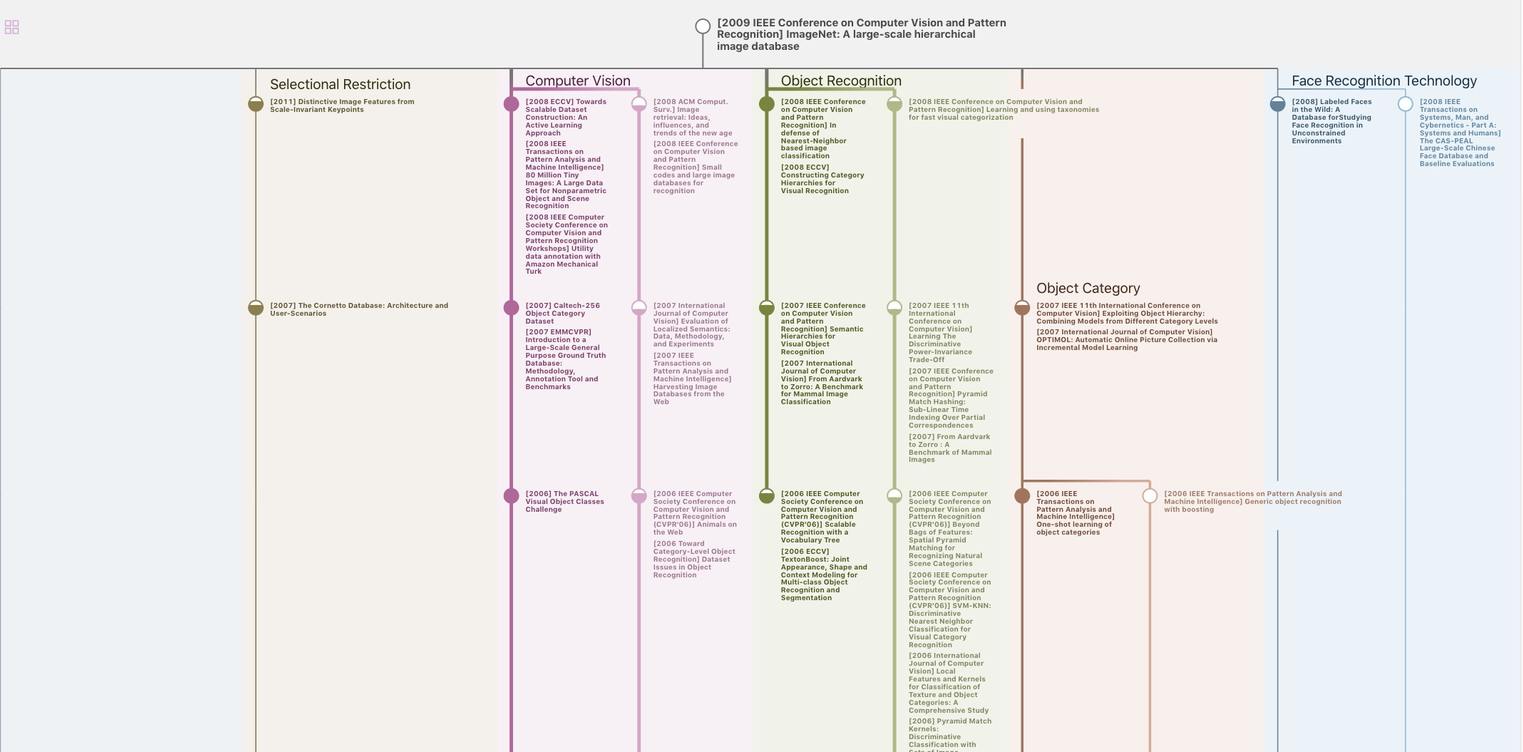
生成溯源树,研究论文发展脉络
Chat Paper
正在生成论文摘要