Deep Semantic Network Representation
20TH IEEE INTERNATIONAL CONFERENCE ON DATA MINING (ICDM 2020)(2020)
摘要
Network representation aims to learn low-dimensional vector representations of network nodes while preserving the inherent properties of the network. For all its popularity, majority of the existing methods focus on exploitation of diverse information, including network topology and semantic information on nodes of network, and ignore their implicit semantics. For example, we all know the saying that birds of a feather flock together. More concretely, semantic information of one node can be influenced by its neighbors' semantic information. Furthermore, even two nodes are not directly connected, they may have similar implicit semantic information (i.e., high-order semantic proximity). Thus, they should be close in the represented vector space.To this end, we propose a Deep Semantic Network Representation approach (DSNR) in the self-translation framework from sequence to sequence. To excavate the implicit semantic information of nodes and capture the high-order semantic proximity, three key components make our approach effective, i.e., aggregation of nodes neighbors' semantic information and enhancement to the semantic feature representations of nodes by a deep autoencoder, integration of nodes semantic information in node identity sequence to generate node semantic sequence, and translation from node semantic sequence to node identity sequence to capture the high-order semantic proximity in an attention-enhanced seq2seq framework. Extensive experiments based on three real-world datasets have verified the effectiveness of our proposed approach.
更多查看译文
关键词
Semantic networks, Network representation, Node semantics, Self-translation, High-order proximity
AI 理解论文
溯源树
样例
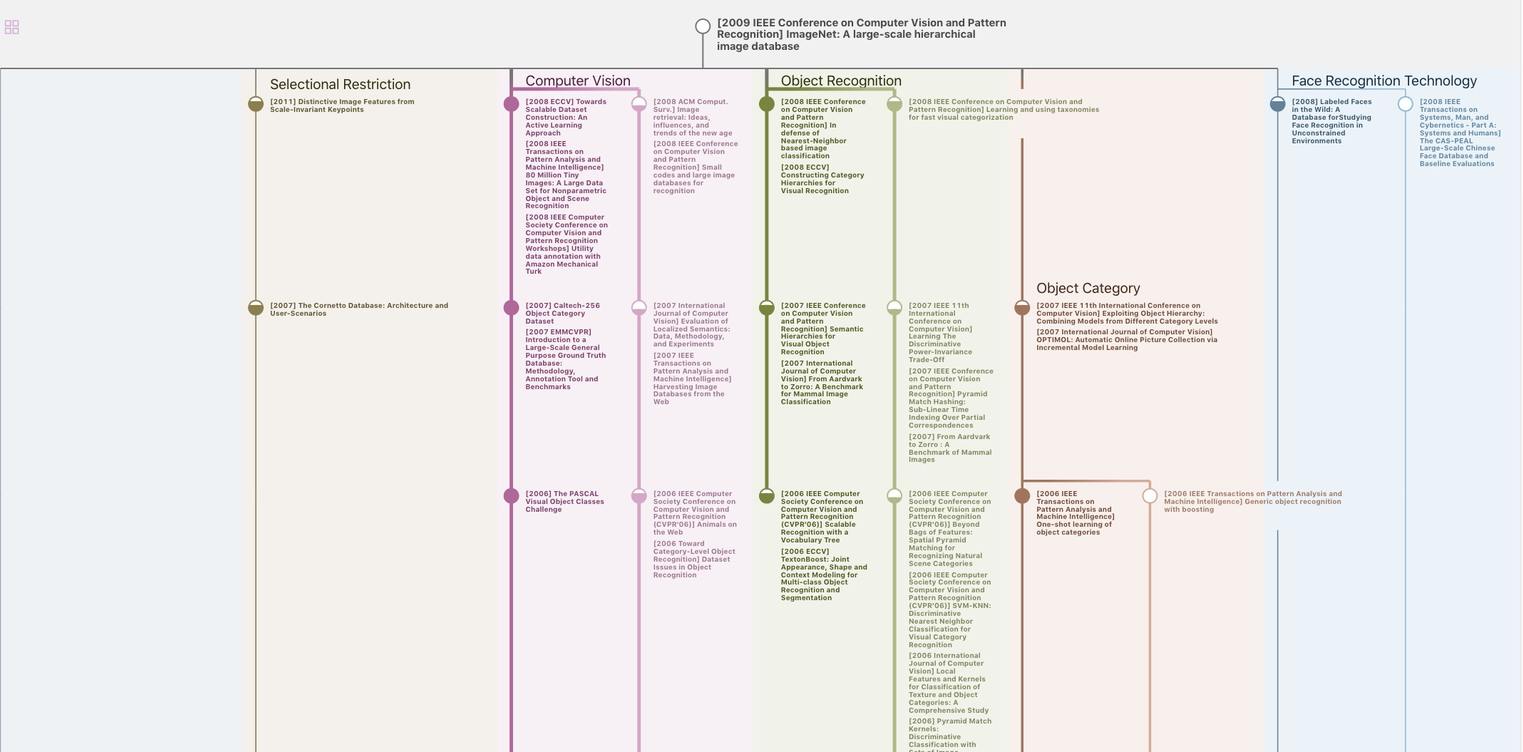
生成溯源树,研究论文发展脉络
Chat Paper
正在生成论文摘要