Nf-Vga: Incorporating Normalizing Flows Into Graph Variational Autoencoder For Embedding Attribute Networks
20TH IEEE INTERNATIONAL CONFERENCE ON DATA MINING (ICDM 2020)(2020)
摘要
Network embedding (NE), aiming to embed a network into a low dimensional latent representation while preserving the inherent structural properties of the network, has attracted considerable attention recently. Variational Autoencoder (VAE) has been widely studied for NE. Existing VAE based methods let the network follow a unimodal distribution, that is, they typically use some fixed distribution as the prior, e.g. Gaussian distribution. However, in reality networks often contain many complicated structural properties [5], [6] (such as the first/second order proximity, the motif or community structures, power-law, etc). The latent representation from unimodal and fixed distribution is not capable of describing such multi-modal characteristic of networks. To address this issue, we develop a new VAE method for NE, named Normalizing Flow Variational Graph Autoencoder (NF-VGA). We design a prior-generative module based on normalizing flows to generate flexible, multi-modal distribution as the prior of the latent representation. To make the generated prior better describe the coupling relationship between nodes, we further utilize network local structures to guide the prior generation. Extensive experiments on some real-world networks show a superior performance of the new approach over some state-of-the-art methods on some popular network embedding tasks.
更多查看译文
关键词
deep learning, network embedding
AI 理解论文
溯源树
样例
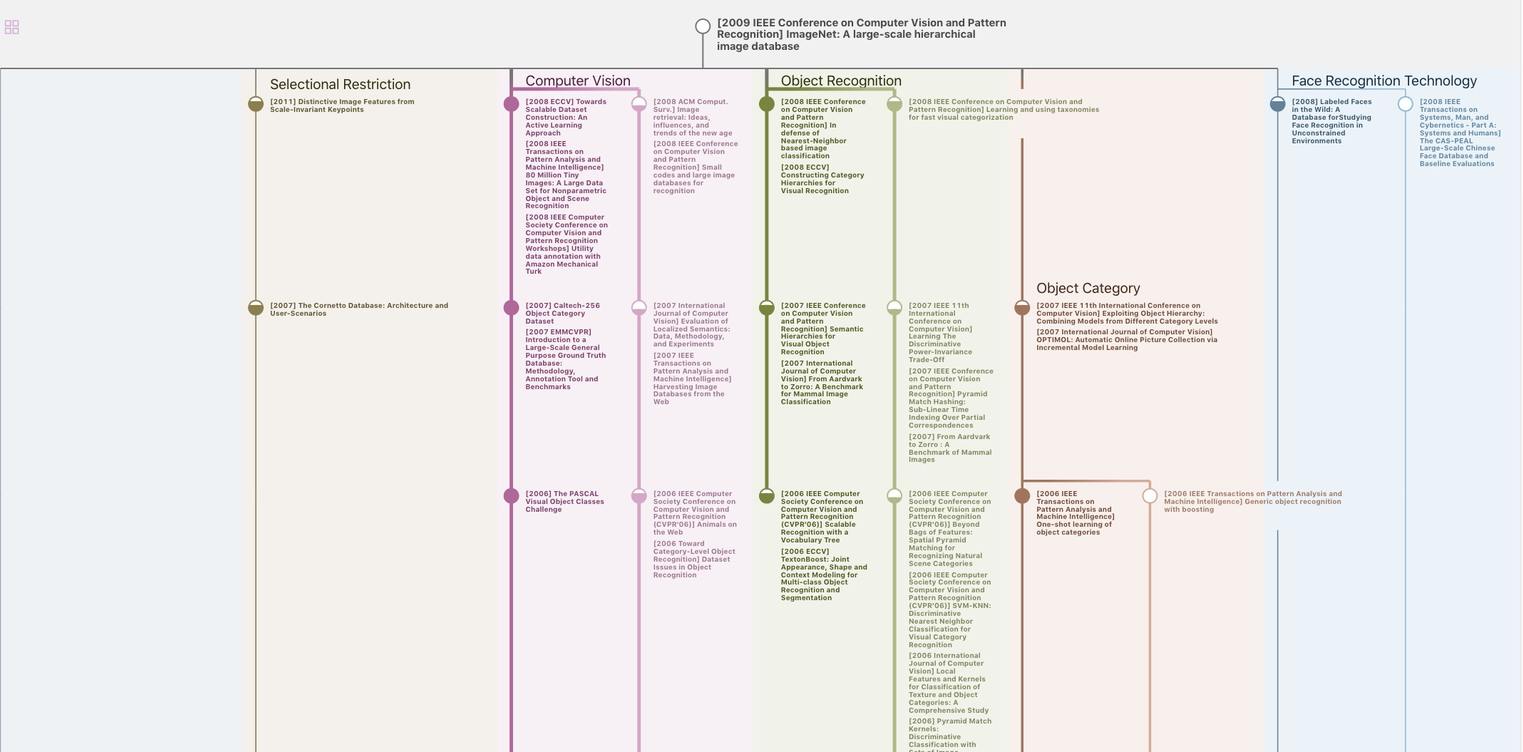
生成溯源树,研究论文发展脉络
Chat Paper
正在生成论文摘要