Learning Periods From Incomplete Multivariate Time Series
20TH IEEE INTERNATIONAL CONFERENCE ON DATA MINING (ICDM 2020)(2020)
摘要
Modeling and detection of seasonality in time series is essential for accurate analysis, prediction and anomaly detection. Examples of seasonal effects at different scales abound: the increase in consumer product sales during the holiday season recurs yearly, and similarly household electricity usage has daily, weekly and yearly cycles. The period in real-world time series, however, may be obfuscated by noise and missing values arising in data acquisition. How can one learn the natural periodicity from incomplete multivariate time series?We propose a robust framework for multivariate period detection, called LAPIS. It encodes incomplete and noisy data as a sparse summary via a Ramanujan periodic dictionary. LAPIS can accurately detect a mixture of multiple periods in the same time series even when 70% of the observations are missing. A key innovation of our framework is that it exploits shared periods across individual time series even when they are not correlated or in-phase. Beyond detecting periods, LAPIS enables improvements in downstream applications such as forecasting, missing value imputation and clustering. At the same time our approach scales to large real-world data executing within seconds on datasets of length up to half a million time points.
更多查看译文
关键词
Period learning, Multivariate time series, Missing data imputation, Alternating Optimization
AI 理解论文
溯源树
样例
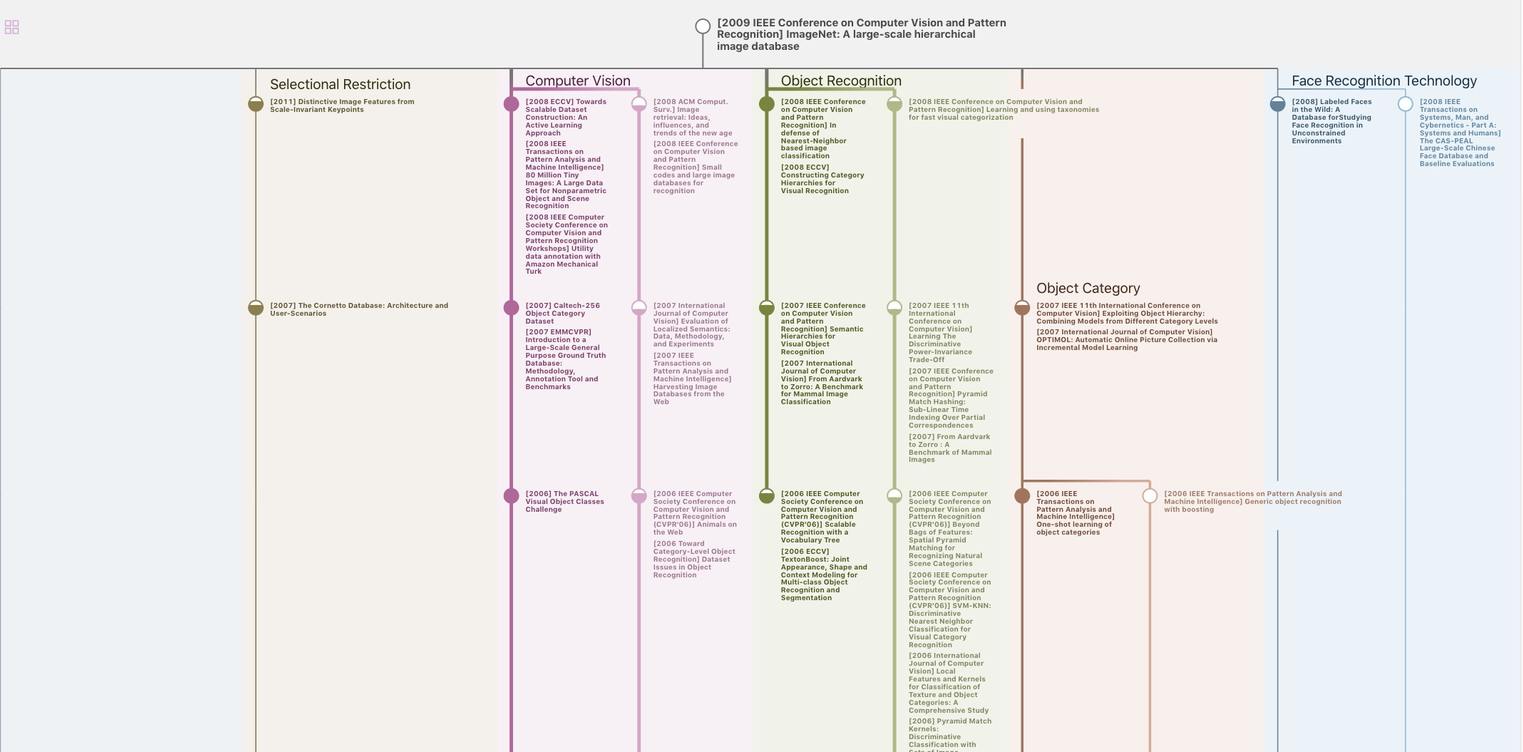
生成溯源树,研究论文发展脉络
Chat Paper
正在生成论文摘要