Federated Depression Detection from Multi-SourceMobile Health Data
arXiv (Cornell University)(2021)
摘要
Depression is one of the most common mental illness problems, and the symptoms shown by patients are not consistent, making it difficult to diagnose in the process of clinical practice and pathological research.Although researchers hope that artificial intelligence can contribute to the diagnosis and treatment of depression, the traditional centralized machine learning needs to aggregate patient data, and the data privacy of patients with mental illness needs to be strictly confidential, which hinders machine learning algorithms clinical application.To solve the problem of privacy of the medical history of patients with depression, we implement federated learning to analyze and diagnose depression. First, we propose a general multi-view federated learning framework using multi-source data,which can extend any traditional machine learning model to support federated learning across different institutions or parties.Secondly, we adopt late fusion methods to solve the problem of inconsistent time series of multi-view data.Finally, we compare the federated framework with other cooperative learning frameworks in performance and discuss the related results.
更多查看译文
关键词
depression,health,data,multi-sourcemobile
AI 理解论文
溯源树
样例
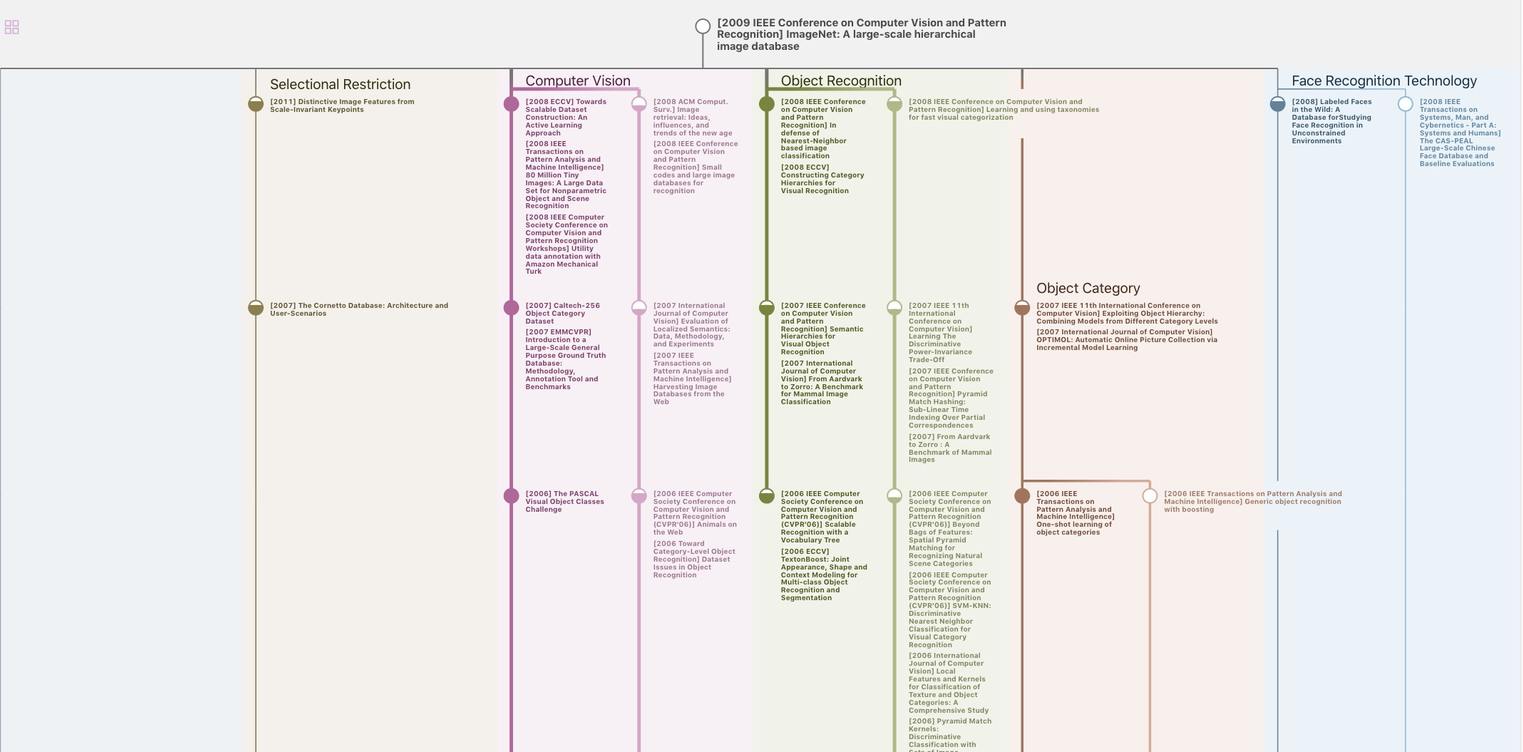
生成溯源树,研究论文发展脉络
Chat Paper
正在生成论文摘要