Sequential and Parallel Constrained Max-value Entropy Search via Information Lower Bound.
International Conference on Machine Learning(2022)
摘要
Max-value entropy search (MES) is one of the state-of-the-art approaches in Bayesian optimization (BO). In this paper, we propose a novel variant of MES for constrained problems, called Constrained MES via Information lower BOund (CMES-IBO), that is based on a Monte Carlo (MC) estimator of a lower bound of a mutual information (MI). Unlike existing studies, our MI is defined so that uncertainty with respect to feasibility can be incorporated. We derive a lower bound of the MI that guarantees non-negativity, while a constrained counterpart of conventional MES can be negative. We further provide theoretical analysis that assures the low-variability of our estimator which has never been investigated for any existing information-theoretic BO. Moreover, using the conditional MI, we extend CMES-IBO to the parallel setting while maintaining the desirable properties. We demonstrate the effectiveness of CMES-IBO by several benchmark functions and real-world problems.
更多查看译文
关键词
entropy,search,max-value
AI 理解论文
溯源树
样例
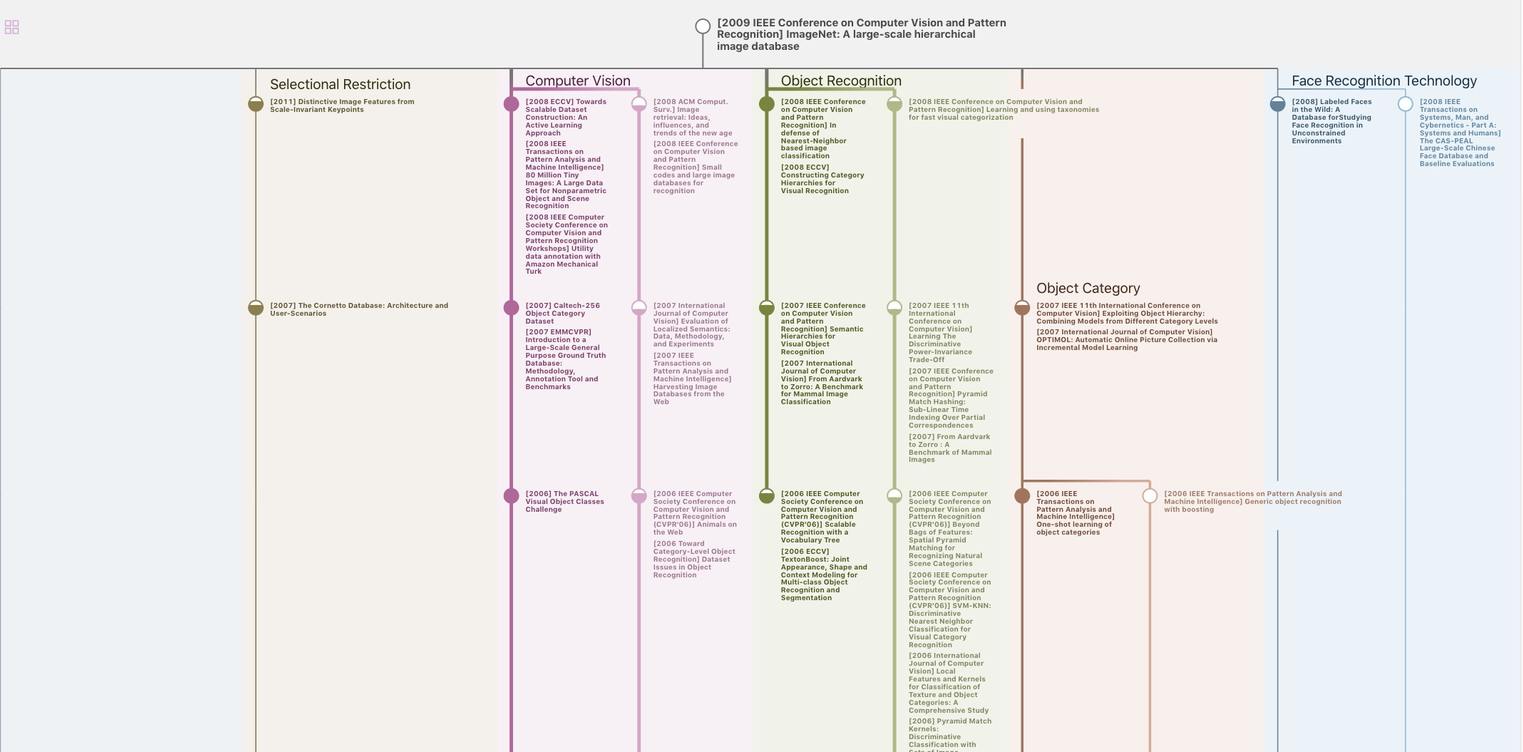
生成溯源树,研究论文发展脉络
Chat Paper
正在生成论文摘要