Automatic Classification of Arrhythmias by Residual Network and BiGRU with Attention Mechanism.
Computing in cardiology(2020)
摘要
Aims: Over the last decade, many attempts have been implemented for automatic detection of cardiac arrhythmias, however, their performances are still not ideal due to unreliable extracted features of designed models or limited small public datasets. In this study, we investigate automatic detection of arrhythmias from 12-lead electrocardiogram (ECG) recordings using an attention-based Res-BiGRU model. Methods: We train a deep neural networks (DNNs) to identify eight types of arrhythmias. The constructed model contains residual convolutional modules and bidirectional Gated Recurrent Unit (BiGRU) layers to extract features from preprocessed ECG signals. The mechanism of attention is applied to learn an attention distribution on the list of extracted features, and sum the weighted features by the attention into a single feature vector, that is responsible for the final classification. We train our model on the training set of the PhysioNet/Computing in Cardiology Challenge 2020 with time length ranging from 6 s to 60 s. The proposed model can handle signals with variable lengths. Results: The overall score with five-cross validation of training set is 0.543 for our team (DeepHeart), which demonstrates a good efficacy of our model, demonstrating that it may have potential practical applications.
更多查看译文
关键词
residual network,attention mechanism,cardiac arrhythmias,attention-based Res-BiGRU model,deep neural networks,residual convolutional modules,bidirectional gated recurrent unit layers,ECG signals,single feature vector,twelve-lead electrocardiogram recordings,automatic classification,feature extraction,five-cross validation,time 6.0 s to 60.0 s
AI 理解论文
溯源树
样例
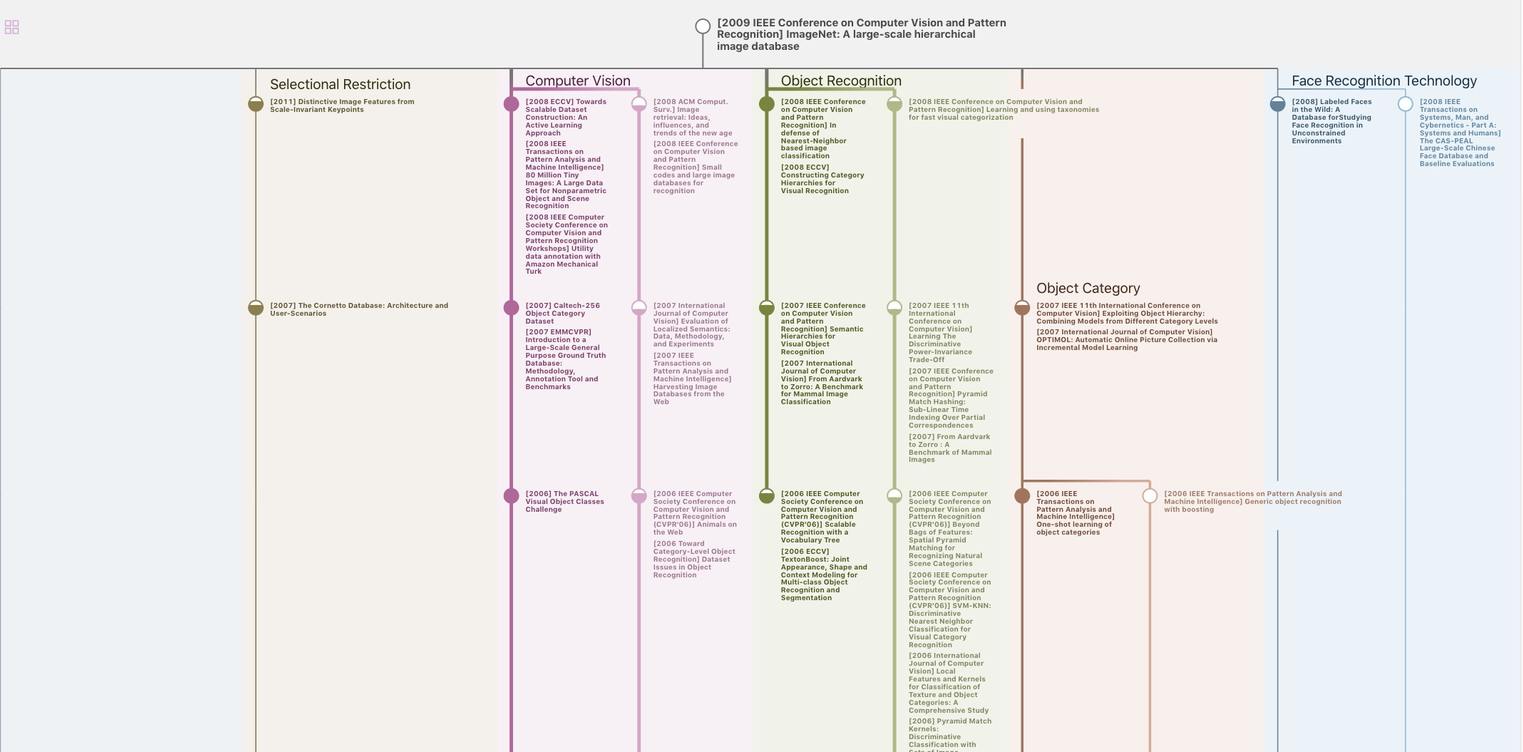
生成溯源树,研究论文发展脉络
Chat Paper
正在生成论文摘要