Multi-Class Classification Of Pathologies Found On Short Ecg Signals
2020 COMPUTING IN CARDIOLOGY(2020)
摘要
The ability to detect several key cardiac pathologies simultaneously, based on ECG signals, is key towards establishing a real-world application of AI models in cardiology. Such a multi-label classification task requires not only well-performing binary classification models, but also a way to combine such models into an overall classification modeling structure. We have approached this task using materials from Classification of 12-lead ECGs for the PhysioNet/Computing in Cardiology Challenge 2020. Duplicate ECG strips have been removed. An annotation tool for labeling ECG wave points and intervals/templates has been created in MATLAB (R), and used for labeling pathological intervals, as well as noisy intervals and inconsistencies between the ECG data and the pre-assigned labels. Several one-vs-rest binary classifiers were built, where morphological features specific to each pathology had been generated from the signals. The binary classifiers were augmented by a multi-class classifier using an Error Correcting Output Codes (ECOC) methodology. Our approach achieved a challenge validation score of 0.616, and full test score of 0.194, placing us 23 (team DSC) out of 41 in the official ranking.
更多查看译文
关键词
multiclass classification,short ECG signals,cardiac pathologies,AI models,multilabel classification task,binary classification models,classification modeling structure,duplicate ECG strips,ECG wave points,pathological intervals,noisy intervals,ECG data,pre-assigned labels,error correcting output codes,ECOC,MATLAB
AI 理解论文
溯源树
样例
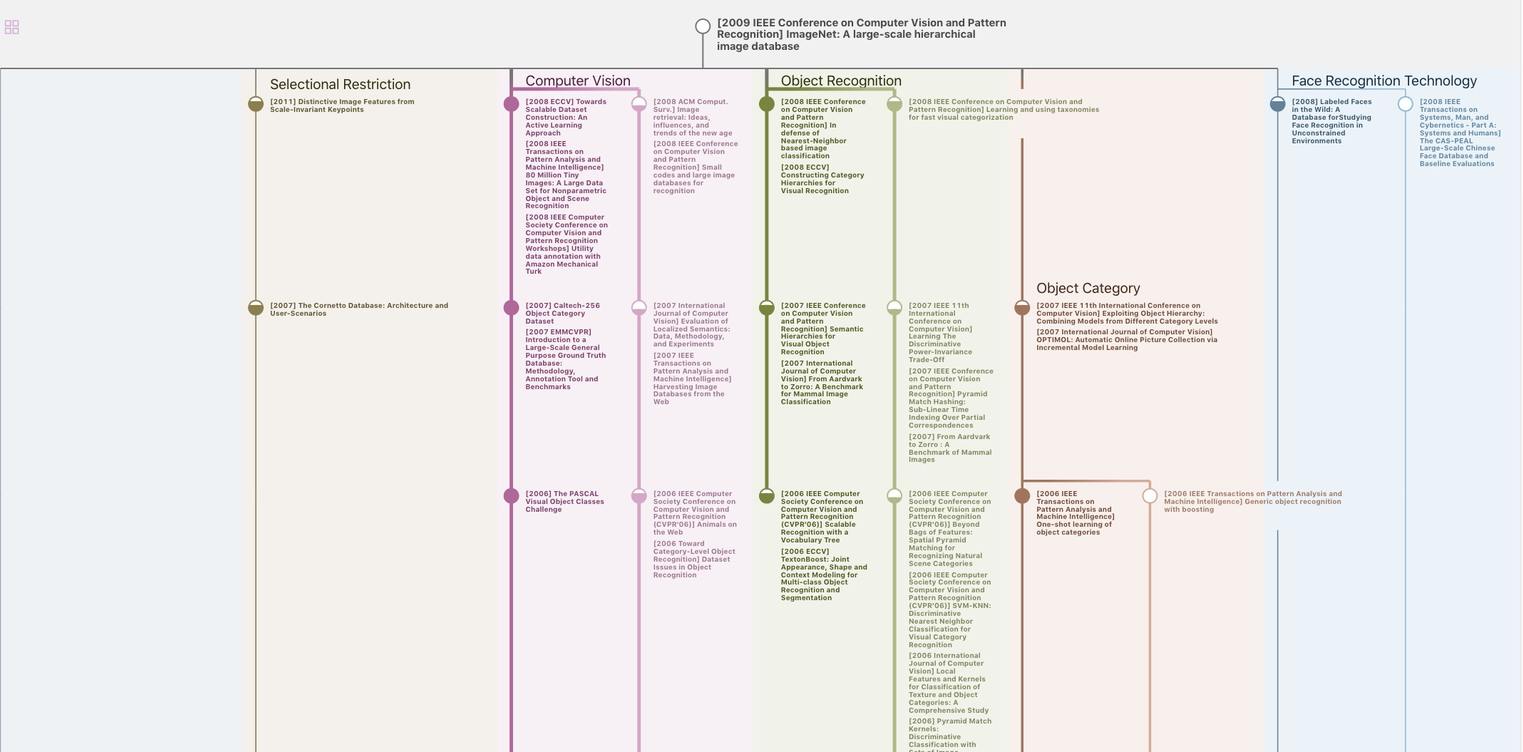
生成溯源树,研究论文发展脉络
Chat Paper
正在生成论文摘要