Automatic Concurrent Arrhythmia Classification Using Deep Residual Neural Networks
2020 COMPUTING IN CARDIOLOGY(2020)
摘要
This paper addresses the PhysioNet/Computing in Cardiology Challenge 2020. The challenge presents a problem to classify 26 types of arrhythmias and normal sinus rhythm using 12-lead electrocardiogram data. We were able to successfully perform the classification task using an eight layer deep residual neural network (ResNet). The skip connections present in the ResNet allowed the model to train faster and produce better challenge score. We also investigated sixteen other models that included convolution and recurrent neural network based models along with interpretability based attention mechanism as all of them are well suited for time series classification problems. The results depicted that the 8 layer ResNet model outperformed other models in terms of challenge score consuming significantly less time during the training phase. We preferred batch wise training to avoid having all the data in memory during training thereby alleviating the problem of memory choking. Our team, deepzx987, obtained a challenge score of 0.305 on validation data, -0.035 on the full test set, and ranked 35th in this year's challenge.
更多查看译文
关键词
automatic concurrent arrhythmia classification,deep residual neural networks,electrocardiogram,skip connections,recurrent neural network,interpretability based attention mechanism,time series classification,ResNet model,batch wise training,PhysioNet-Computing in Cardiology Challenge 2020,arrhythmias,normal sinus rhythm,convolution neural network,memory choking
AI 理解论文
溯源树
样例
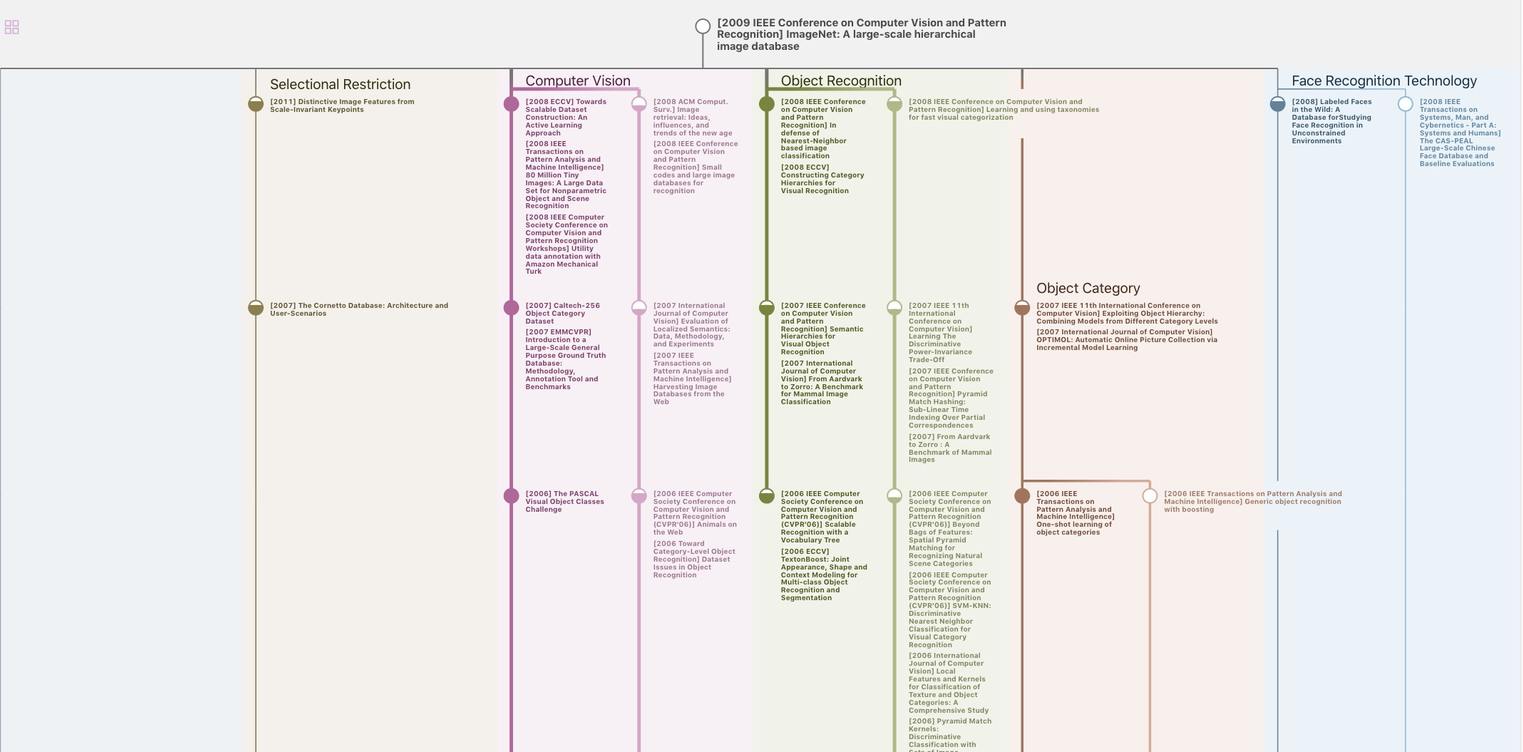
生成溯源树,研究论文发展脉络
Chat Paper
正在生成论文摘要