Treatment effect estimation with Multilevel Regression and Poststratification
arxiv(2021)
摘要
Multilevel regression and poststratification (MRP) is a flexible modeling technique that has been used in a broad range of small-area estimation problems. Traditionally, MRP studies have been focused on non-causal settings, where estimating a single population value using a nonrepresentative sample was of primary interest. In this manuscript, MRP-style estimators will be evaluated in an experimental causal inference setting. We simulate a large-scale randomized control trial with a stratified cluster sampling design, and compare traditional and nonparametric treatment effect estimation methods with MRP methodology. Using MRP-style estimators, treatment effect estimates for areas as small as 1.3$\%$ of the population have lower bias and variance than standard causal inference methods, even in the presence of treatment effect heterogeneity. The design of our simulation studies also requires us to build upon a MRP variant that allows for non-census covariates to be incorporated into poststratification.
更多查看译文
关键词
multilevel regression,poststratification,treatment,effect,estimation
AI 理解论文
溯源树
样例
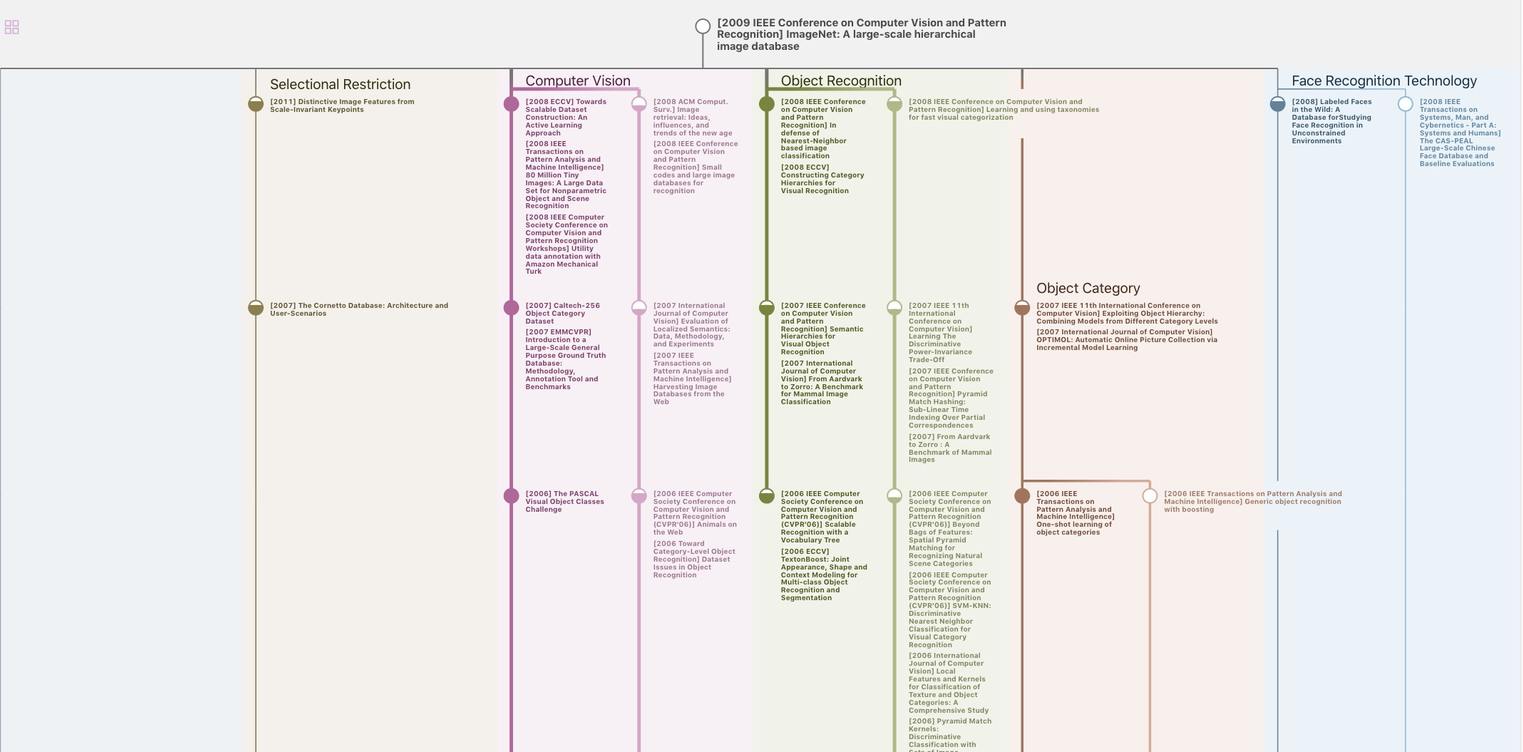
生成溯源树,研究论文发展脉络
Chat Paper
正在生成论文摘要