Separating Stimulus-Induced and Background Components of Dynamic Functional Connectivity in Naturalistic fMRI
IEEE Transactions on Medical Imaging(2022)
摘要
We consider the challenges in extracting stimulus-related neural dynamics from other intrinsic processes and noise in naturalistic functional magnetic resonance imaging (fMRI). Most studies rely on inter-subject correlations (ISC) of low-level regional activity and neglect varying responses in individuals. We propose a novel, data-driven approach based on low-rank plus sparse (
$\text{L}+\text{S}$
) decomposition to isolate stimulus-driven dynamic changes in brain functional connectivity (FC) from the background noise, by exploiting shared network structure among subjects receiving the same naturalistic stimuli. The time-resolved multi-subject FC matrices are modeled as a sum of a low-rank component of correlated FC patterns across subjects, and a sparse component of subject-specific, idiosyncratic background activities. To recover the shared low-rank subspace, we introduce a fused version of principal component pursuit (PCP) by adding a fusion-type penalty on the differences between the columns of the low-rank matrix. The method improves the detection of stimulus-induced group-level homogeneity in the FC profile while capturing inter-subject variability. We develop an efficient algorithm via a linearized alternating direction method of multipliers to solve the fused-PCP. Simulations show accurate recovery by the fused-PCP even when a large fraction of FC edges are severely corrupted. When applied to natural fMRI data, our method reveals FC changes that were time-locked to auditory processing during movie watching, with dynamic engagement of sensorimotor systems for speech-in-noise. It also provides a better mapping to auditory content in the movie than ISC.
更多查看译文
关键词
Algorithms,Brain,Brain Mapping,Humans,Magnetic Resonance Imaging,Motion Pictures
AI 理解论文
溯源树
样例
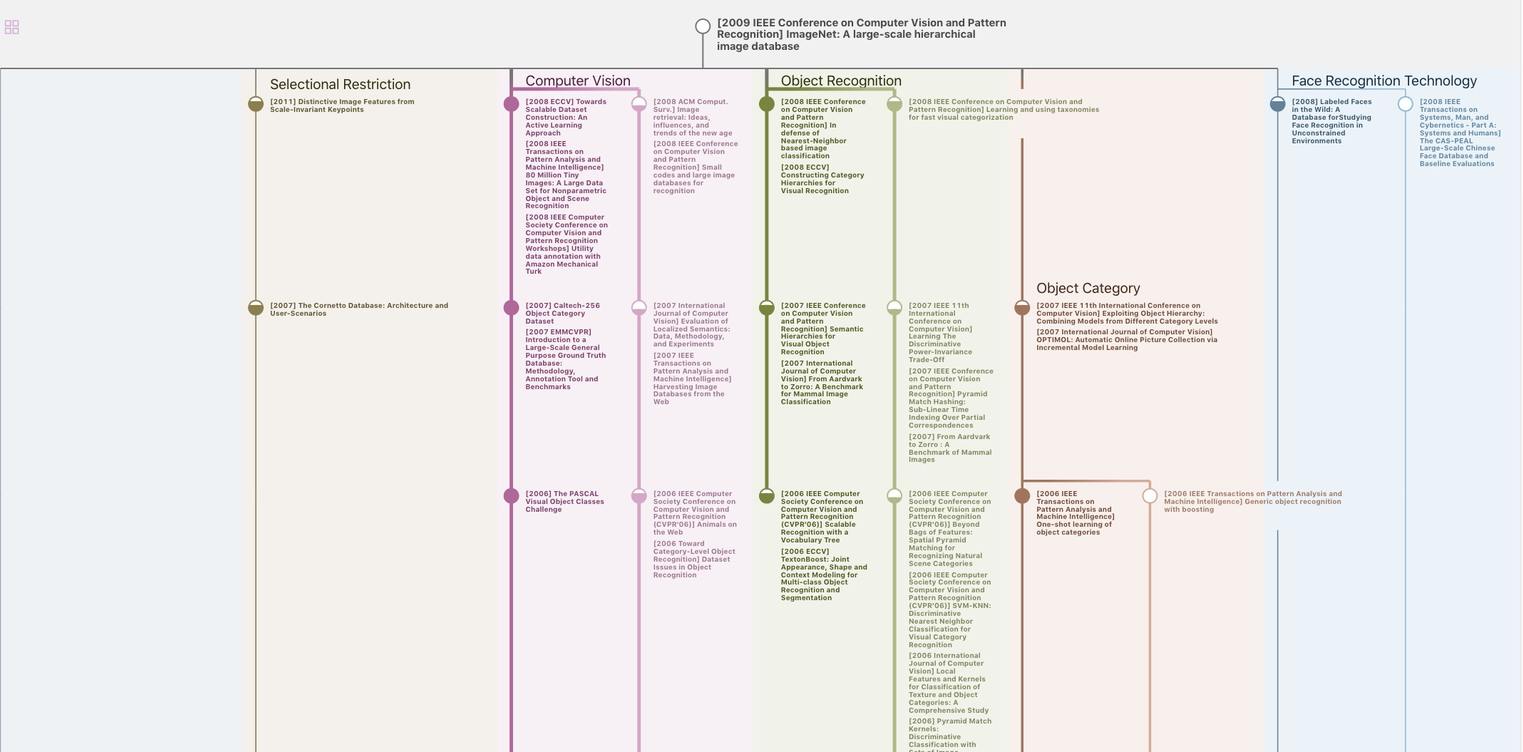
生成溯源树,研究论文发展脉络
Chat Paper
正在生成论文摘要