Heterogeneous Demand Effects of Recommendation Strategies in a Mobile Application: Evidence from Econometric Models and Machine-Learning Instruments.
MIS Quarterly(2022)
摘要
In this paper, we examine the effectiveness of various recommendation strategies in the mobile channel and their impact on consumers' utility and demand levels for individual products. We find significant differences in effectiveness among various recommendation strategies. Interestingly, recommendation strategies that directly embed social proofs for the recommended alternatives outperform other recommendations. Besides, recommendation strategies combining social proofs with higher levels of induced awareness due to the prescribed temporal diversity have an even stronger effect on the mobile channel. In addition, we examine the heterogeneity of the demand effect across items, users, and contextual settings, further verifying empirically the aforementioned information and persuasion mechanisms and generating rich insights. We also facilitate the estimation of causal effects in the presence of endogeneity using machine-learning methods. Specifically, we develop novel econometric instruments that capture product differentiation (isolation) based on deep-learning models of user-generated reviews. Our empirical findings extend the current knowledge regarding the heterogeneous impact of recommender systems, reconcile contradictory prior results in the related literature, and have significant business implications.
更多查看译文
关键词
Recommendations, demand, mobile, econometrics, instrumental variables, deep learning, machine learning
AI 理解论文
溯源树
样例
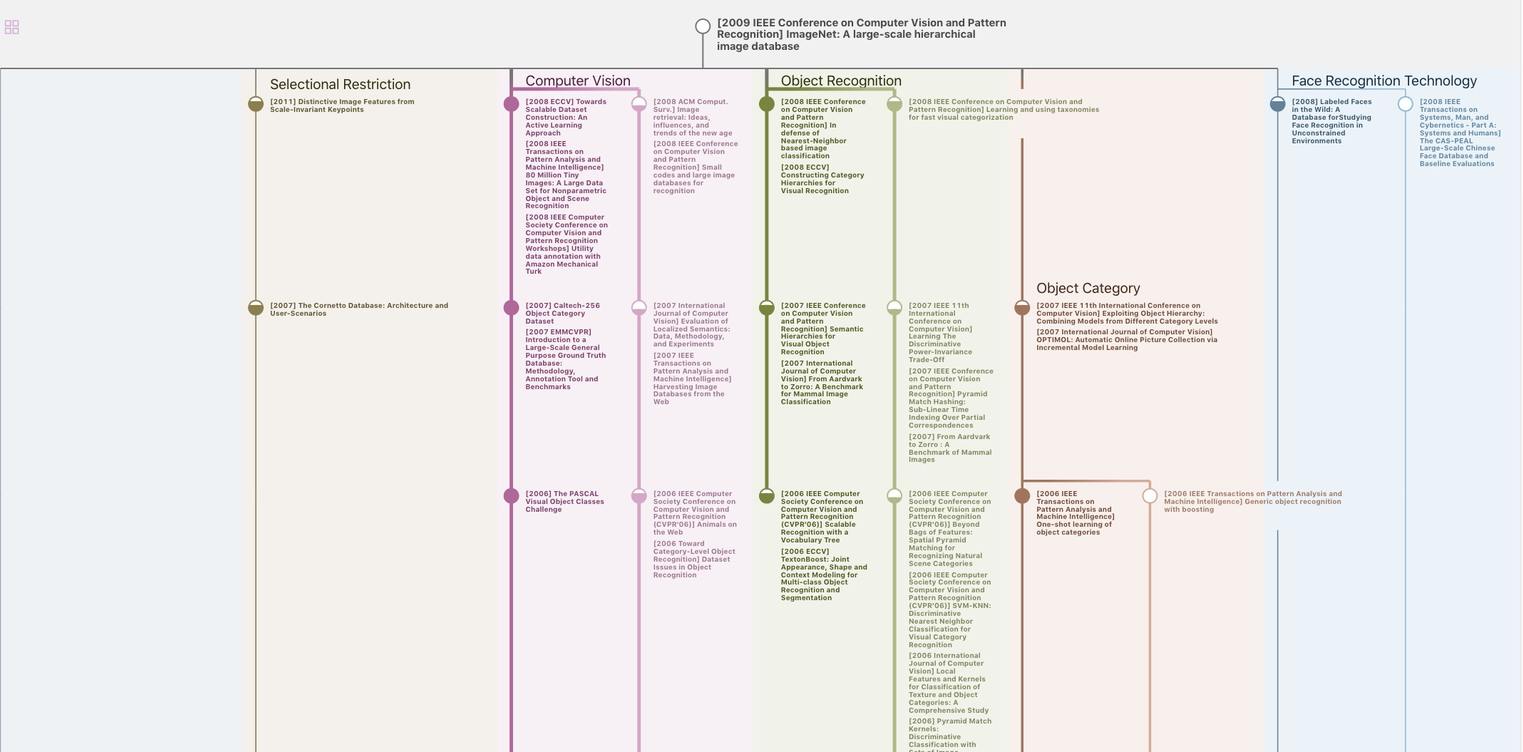
生成溯源树,研究论文发展脉络
Chat Paper
正在生成论文摘要