Concentration estimates for functions of finite high-dimensional random arrays
arxiv(2021)
摘要
Let $\boldsymbol{X}$ be a $d$-dimensional random array on $[n]$ whose entries take values in a finite set $\mathcal{X}$, that is, $\boldsymbol{X}=\langle X_s:s\in {[n]\choose d}\rangle$ is an $\mathcal{X}$-valued stochastic process indexed by the set ${[n]\choose d}$ of all $d$-element subsets of $[n]:=\{1,\dots,n\}$. We give sufficient -- and easily checked -- conditions on the random array $\boldsymbol{X}$ which ensure that for every function $f\colon \mathcal{X}^{{[n]\choose d}}\to\mathbb{R}$ which satisfies $\mathbb{E}[f(\boldsymbol{X})]=0$ and $\|f(\boldsymbol{X})\|_{L_p}=1$ for some $p>1$, the random variable $f(\boldsymbol{X})$ becomes concentrated after conditioning it on a large subarray of $\boldsymbol{X}$; these conditions cover wide classes of random arrays with not necessarily independent entries. Examples are also given which show the optimality of various aspects of the results. The proof is based on analytic and probabilistic tools -- in particular, estimates for martingale difference sequences in $L_p$ spaces -- and reduces the conditional concentration of the random variable $f(\boldsymbol{X})$ to (an approximate form of) the dissociativity of the random array $\boldsymbol{X}$. The latter is then obtained using combinatorial arguments.
更多查看译文
关键词
concentration inequalities, density polynomial Hales-Jewett conjecture, exchangeable random arrays, martingale difference sequences, quasirandomness, spreadable random arrays
AI 理解论文
溯源树
样例
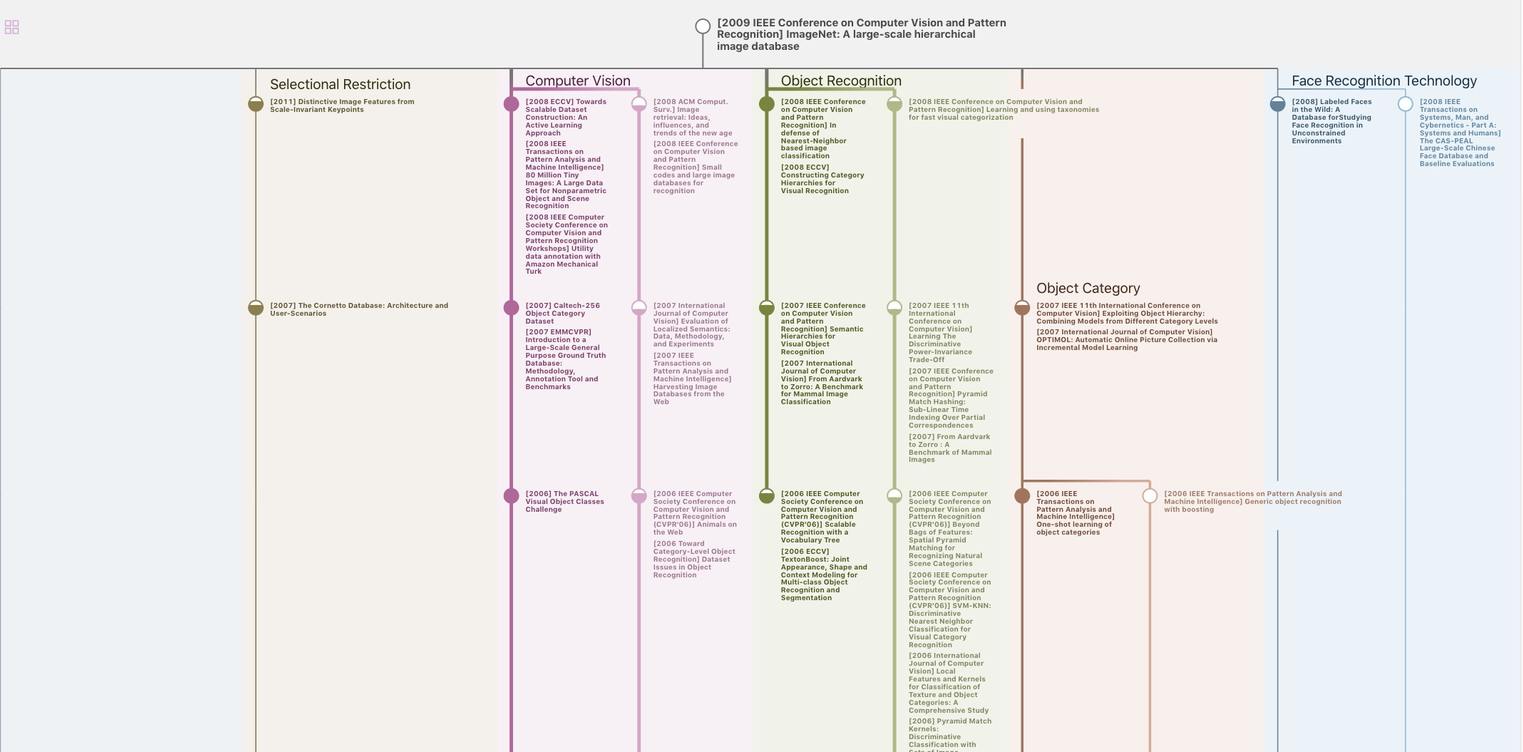
生成溯源树,研究论文发展脉络
Chat Paper
正在生成论文摘要