Interpret-able feedback for AutoML systems
arxiv(2021)
摘要
Automated machine learning (AutoML) systems aim to enable training machine learning (ML) models for non-ML experts. A shortcoming of these systems is that when they fail to produce a model with high accuracy, the user has no path to improve the model other than hiring a data scientist or learning ML -- this defeats the purpose of AutoML and limits its adoption. We introduce an interpretable data feedback solution for AutoML. Our solution suggests new data points for the user to label (without requiring a pool of unlabeled data) to improve the model's accuracy. Our solution analyzes how features influence the prediction among all ML models in an AutoML ensemble, and we suggest more data samples from feature ranges that have high variance in such analysis. Our evaluation shows that our solution can improve the accuracy of AutoML by 7-8% and significantly outperforms popular active learning solutions in data efficiency, all the while providing the added benefit of being interpretable.
更多查看译文
关键词
automl systems,feedback,interpret-able
AI 理解论文
溯源树
样例
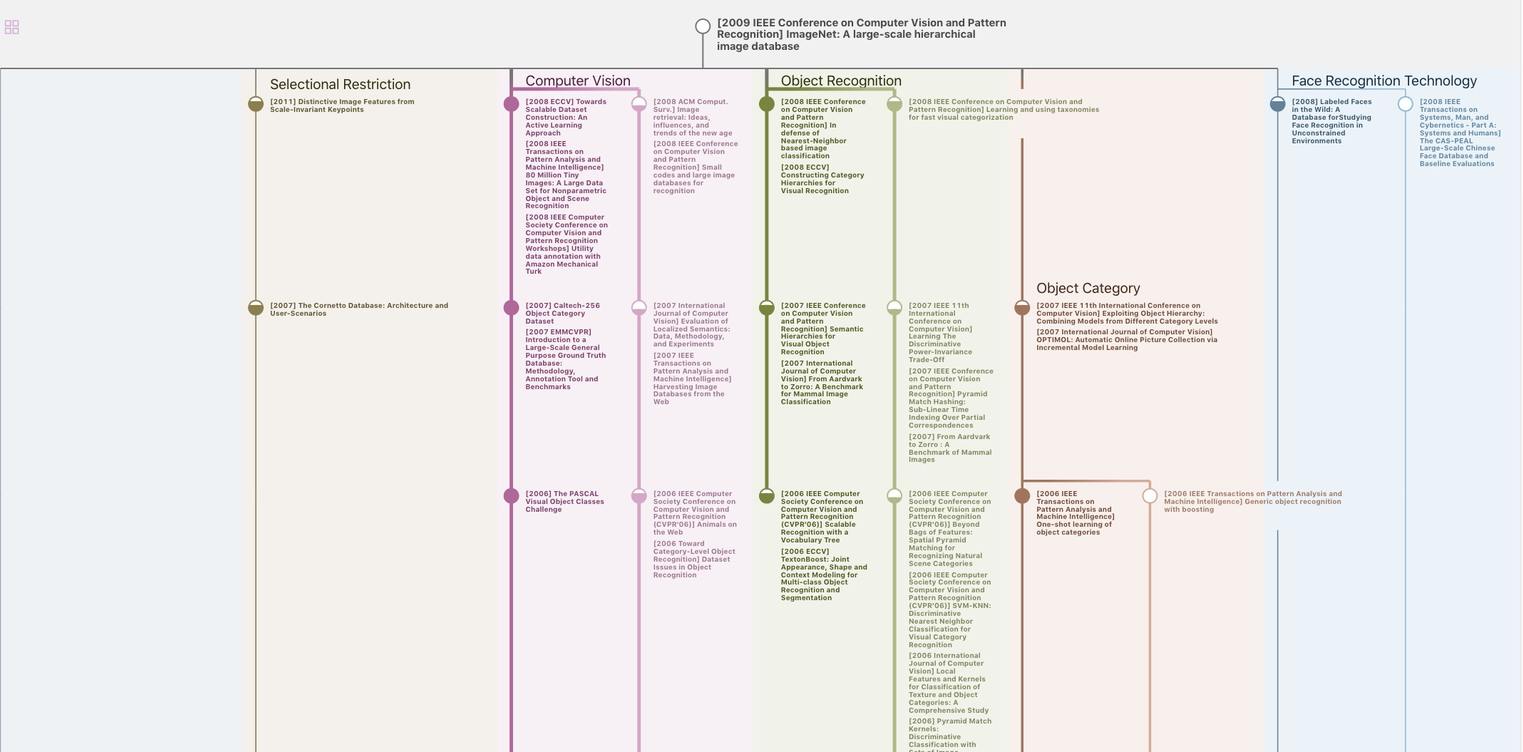
生成溯源树,研究论文发展脉络
Chat Paper
正在生成论文摘要