Direct-Search For A Class Of Stochastic Min-Max Problems
24TH INTERNATIONAL CONFERENCE ON ARTIFICIAL INTELLIGENCE AND STATISTICS (AISTATS)(2021)
摘要
Recent applications in machine learning have renewed the interest of the community in min-max optimization problems. While gradient-based optimization methods are widely used to solve such problems, there are however many scenarios where these techniques are not well-suited, or even not applicable when the gradient is not accessible. We investigate the use of direct-search methods that belong to a class of derivativefree techniques that only access the objective function through an oracle. In this work, we design a novel algorithm in the context of min-max saddle point games where one sequentially updates the min and the max player. We prove convergence of this algorithm under mild assumptions, where the objective of the max-player satisfies the Polyak-Lojasiewicz (PL) condition, while the minplayer is characterized by a nonconvex objective. Our method only assumes dynamically adjusted accurate estimates of the oracle with a fixed probability. To the best of our knowledge, our analysis is the first one to address the convergence of a direct-search method for min-max objectives in a stochastic setting.
更多查看译文
关键词
stochastic,direct-search,min-max
AI 理解论文
溯源树
样例
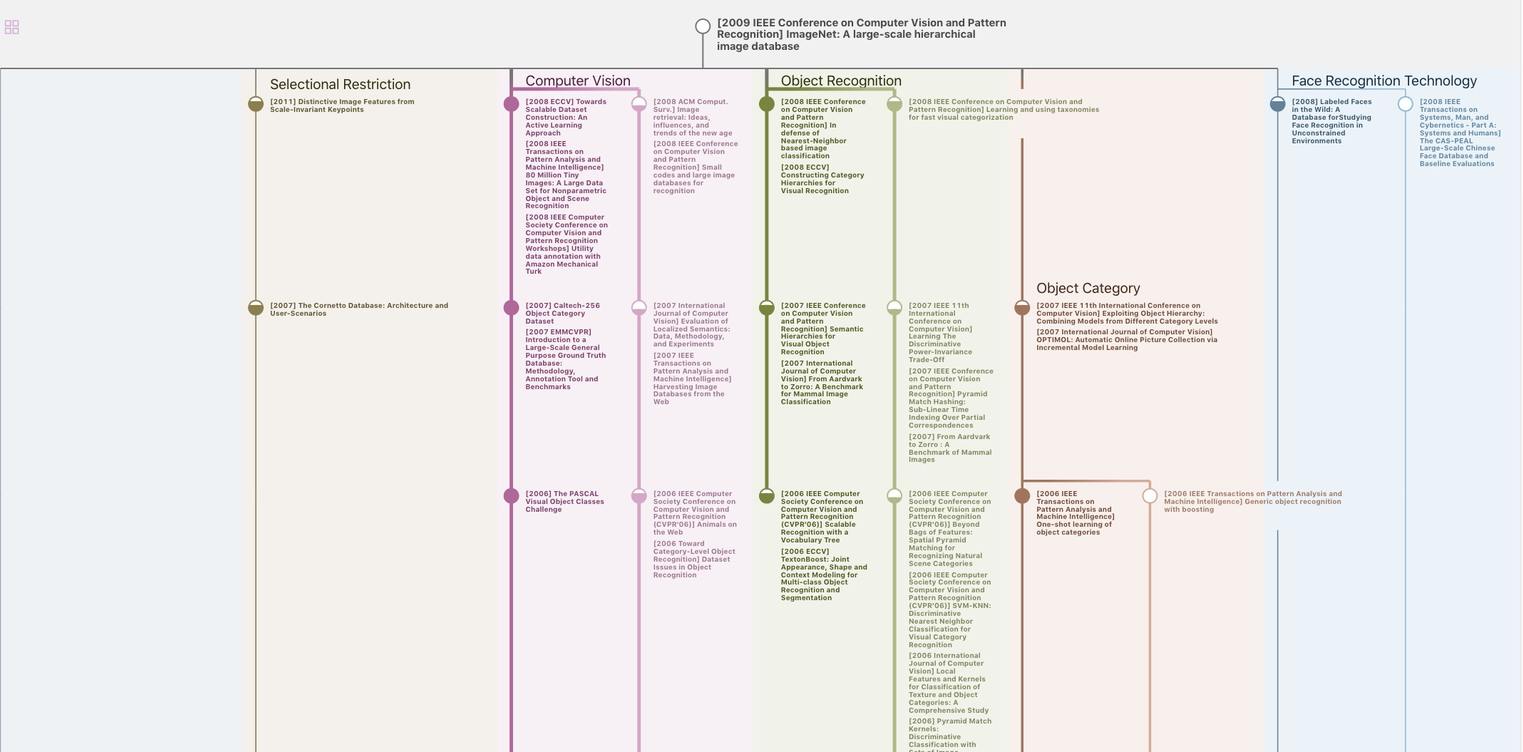
生成溯源树,研究论文发展脉络
Chat Paper
正在生成论文摘要