Unsupervised Multitemporal Building Change Detection Framework Based On Cosegmentation Using Time-Series Sar
REMOTE SENSING(2021)
摘要
Building change detection using remote sensing images is essential for various applications such as urban management and marketing planning. However, most change detection approaches can only detect the intensity or type of change. The aim of this study is to dig for more change information from time-series synthetic aperture radar (SAR) images, such as the change frequency and the change moments. This paper proposes a novel multitemporal building change detection framework that can generate change frequency map (CFM) and change moment maps (CMMs) from multitemporal SAR images. We first give definitions of CFM and CMMs. Then we generate change feature using four proposed generators. After that, a new cosegmentation method combining raw images and change feature is proposed to divide time-series images into changed and unchanged areas separately. Secondly, the proposed cosegmentation and the morphological building index (MBI) are combined to extract changed building objects. Then, the logical conjunction between the cosegmentation results and the binarized MBI is performed to recognize every moment of change. In the post-processing step, we use fragment removal to increase accuracy. Finally, we propose a novel accuracy assessment index for CFM. We call this index average change difference (ACD). Compared to the traditional multitemporal change detection methods, our method outperforms other approaches in terms of both qualitative results and quantitative indices of ACD using two TerraSAR-X datasets. The experiments show that the proposed method is effective in generating CFM and CMMs.
更多查看译文
关键词
cosegmentation, multitemporal change detection, object-based change detection (OBCD), SAR image
AI 理解论文
溯源树
样例
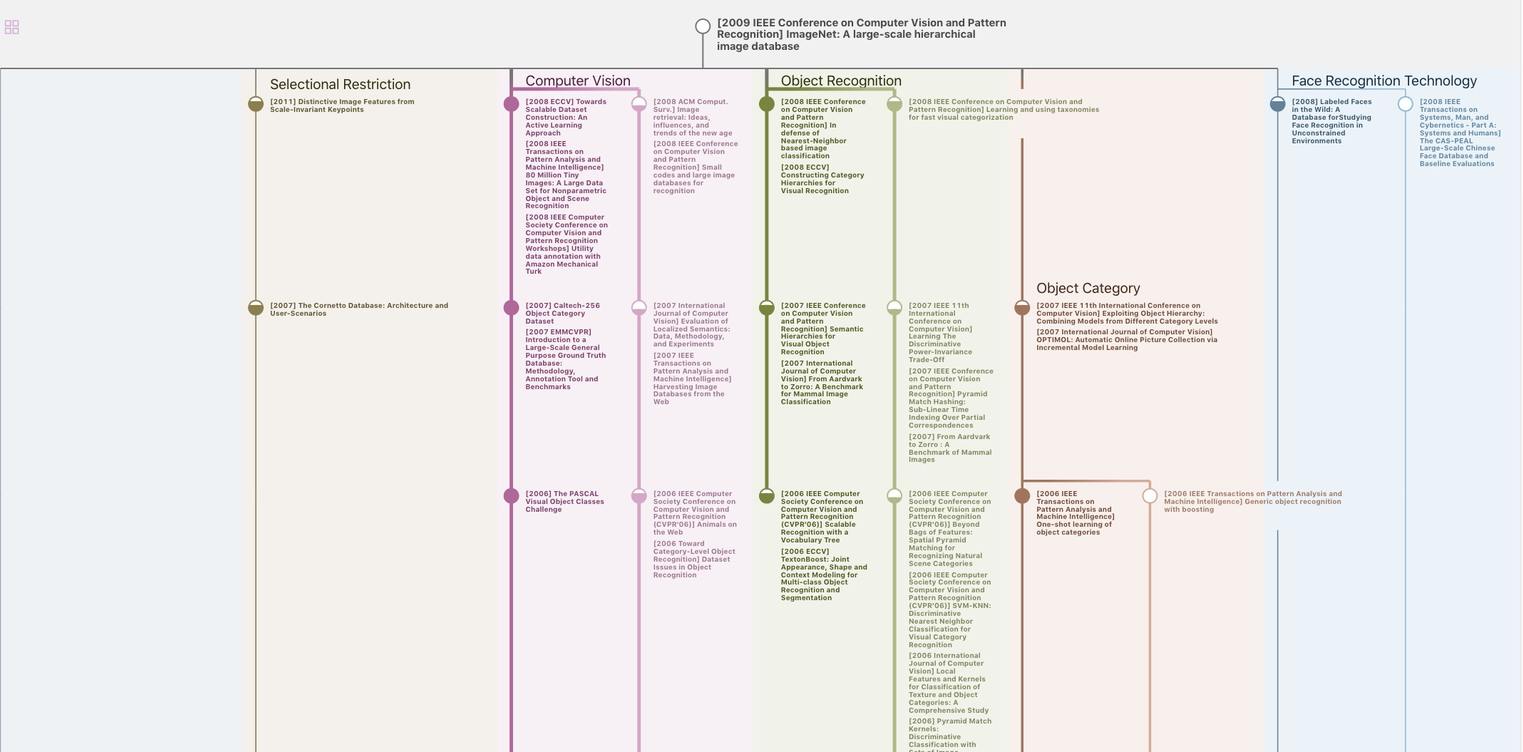
生成溯源树,研究论文发展脉络
Chat Paper
正在生成论文摘要