Unsupervised Metric For Large-Scale Cloud Mask Evaluation
IGARSS 2020 - 2020 IEEE INTERNATIONAL GEOSCIENCE AND REMOTE SENSING SYMPOSIUM(2020)
摘要
Determining the efficacy of analytics at scale, especially when considering remote sensing imagery, provides unique challenges that the community has only recently been able to explore. The need for scaled analytics has become acute with the broad availability of geospatial platforms and the inability for classic modeling analytics to keep scale. In this paper, we discuss ongoing efforts to address this gap as it pertains to data correction. We illustrate the usage of a metric that takes into account the variation between scenes to create an unsupervised measurement of improved cloud masking performance. It is used to illustrate 46.7% relative improved performance of a deep learning based cloud mask on Sentinel-2 imagery as measured against the vendor provided cloud mask.
更多查看译文
关键词
Data correction, cloud masking, noise
AI 理解论文
溯源树
样例
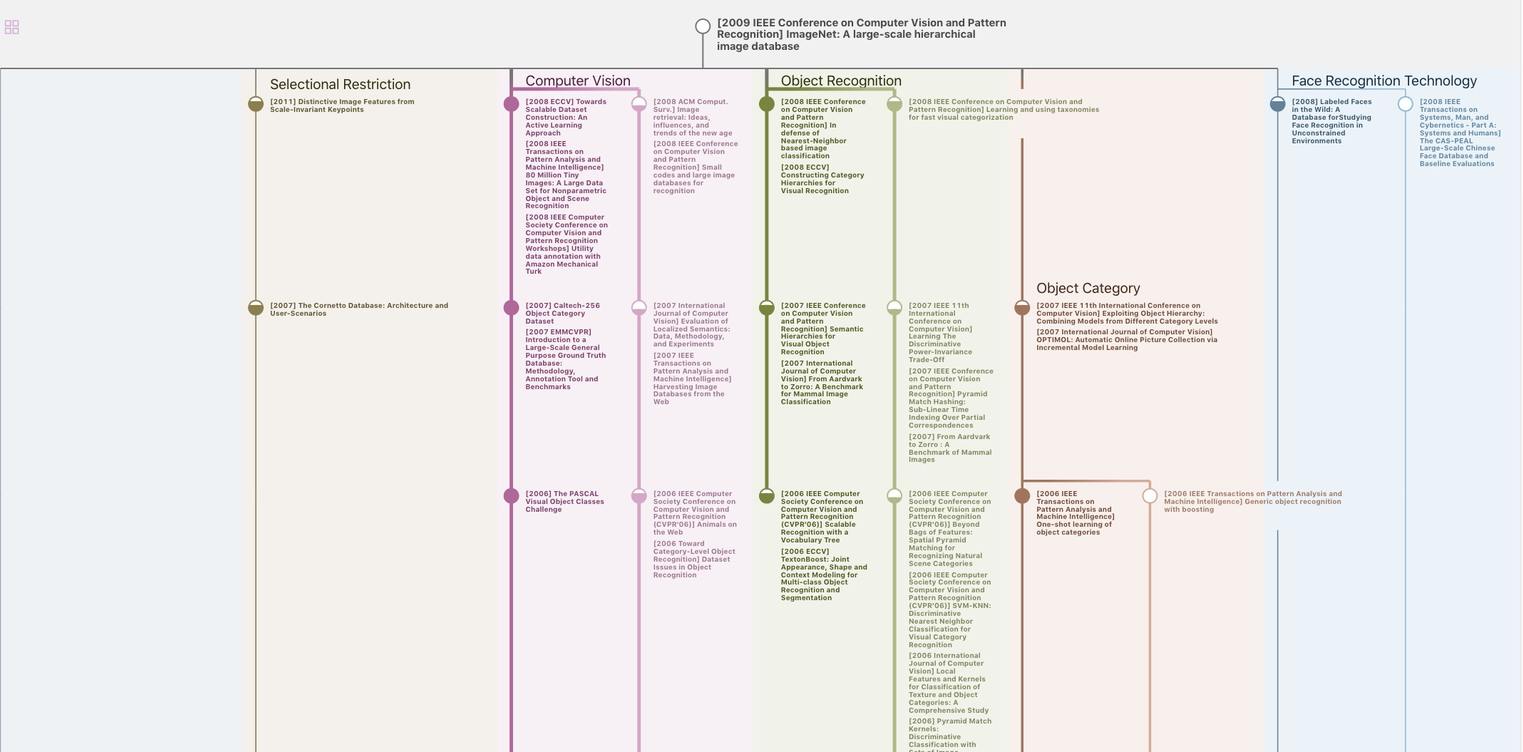
生成溯源树,研究论文发展脉络
Chat Paper
正在生成论文摘要