Scaling Up A Multispectral Resnet-50 To 128 Gpus
IGARSS 2020 - 2020 IEEE INTERNATIONAL GEOSCIENCE AND REMOTE SENSING SYMPOSIUM(2020)
摘要
Similarly to other scientific domains, Deep Learning (DL) holds great promises to fulfil the challenging needs of Remote Sensing (RS) applications. However, the increase in volume, variety and complexity of acquisitions that are carried out on a daily basis by Earth Observation (EO) missions generates new processing and storage challenges within operational processing pipelines. The aim of this work is to show that High-Performance Computing (HPC) systems can speed up the training time of Convolutional Neural Networks (CNNs). Particular attention is put on the monitoring of the classification accuracy that usually degrades when using large batch sizes. The experimental results of this work show that the training of the model scales up to a batch size of 8,000, obtaining classification performances in terms of accuracy in line with those using smaller batch sizes.
更多查看译文
关键词
Distributed deep learning, high performance computing, residual neural network, convolutional neural network, classification, sentinel-2
AI 理解论文
溯源树
样例
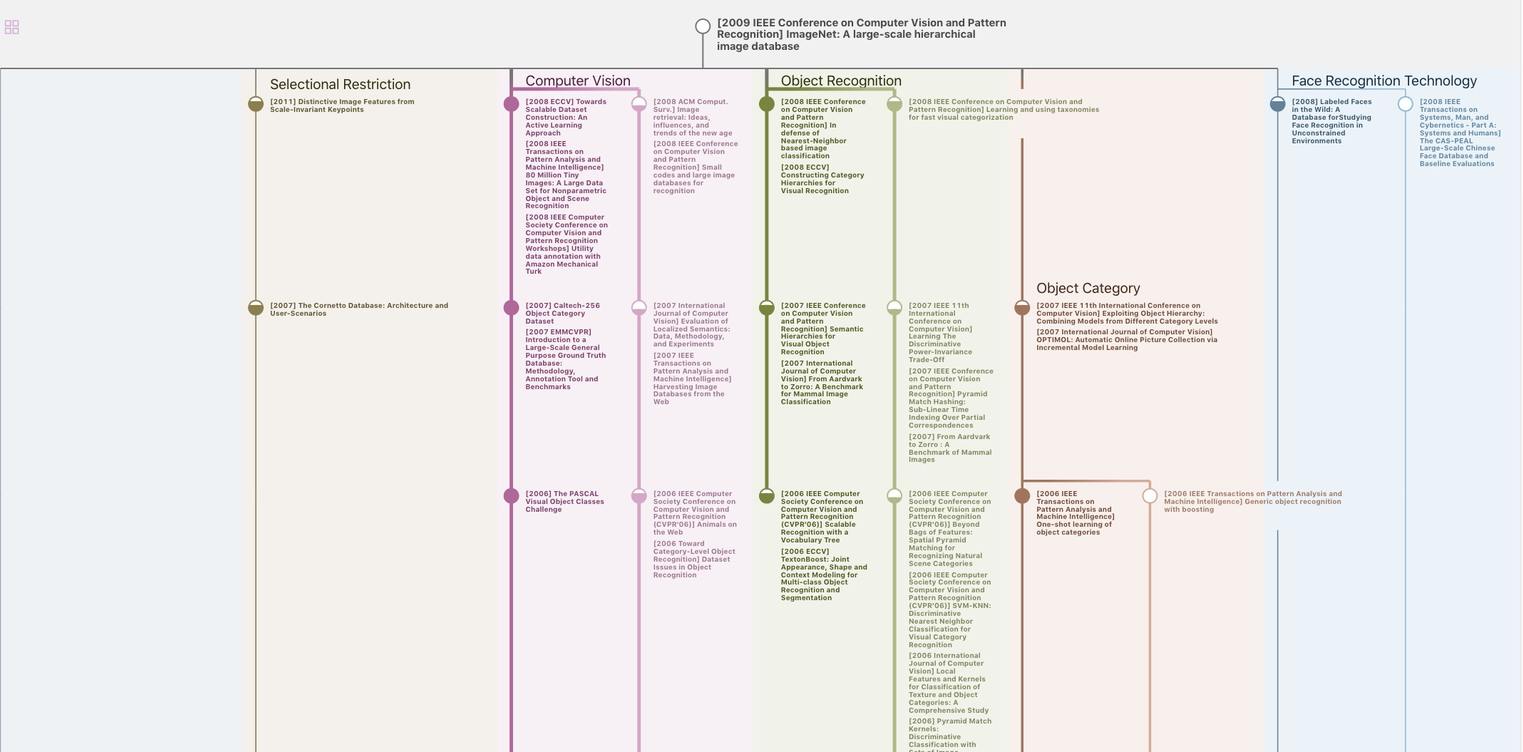
生成溯源树,研究论文发展脉络
Chat Paper
正在生成论文摘要