Self-Paced Learning with Superpixelwise Features for Hyperspectral Image Classification
IGARSS 2020 - 2020 IEEE International Geoscience and Remote Sensing Symposium(2020)
摘要
We explore self-paced boost learning (SPBL) with superpix-elwise features for hyperspectral image classification (HIC). Firstly, we conduct feature extraction using superpixelwise principal component analysis (SuperPCA), which reduces the dimensionality of hyperspectral images considering the project discrepancy in different homogeneous regions. Secondly, we perform classification by SPBL on the extracted features, where the learning just focuses on the pixels to be classified and does not make their spatial neighbours involved. SPBL embraces the power of self-paced learning on classifying from simple to complex and that of boost learning on classifying in a robust fashion. Our method is not deep learning grounded and the training does not demand high computing resources. The experimental results on two public hyperspectral image datasets demonstrate that our method is competitive with several prominent ones.
更多查看译文
关键词
Hyperspectral Images (HSIs),Hyperspectral Image Classification (HIC),Superpixelwise Principal Component Analysis (SuperPCA),Self-paced Boost Learning (SPBL)
AI 理解论文
溯源树
样例
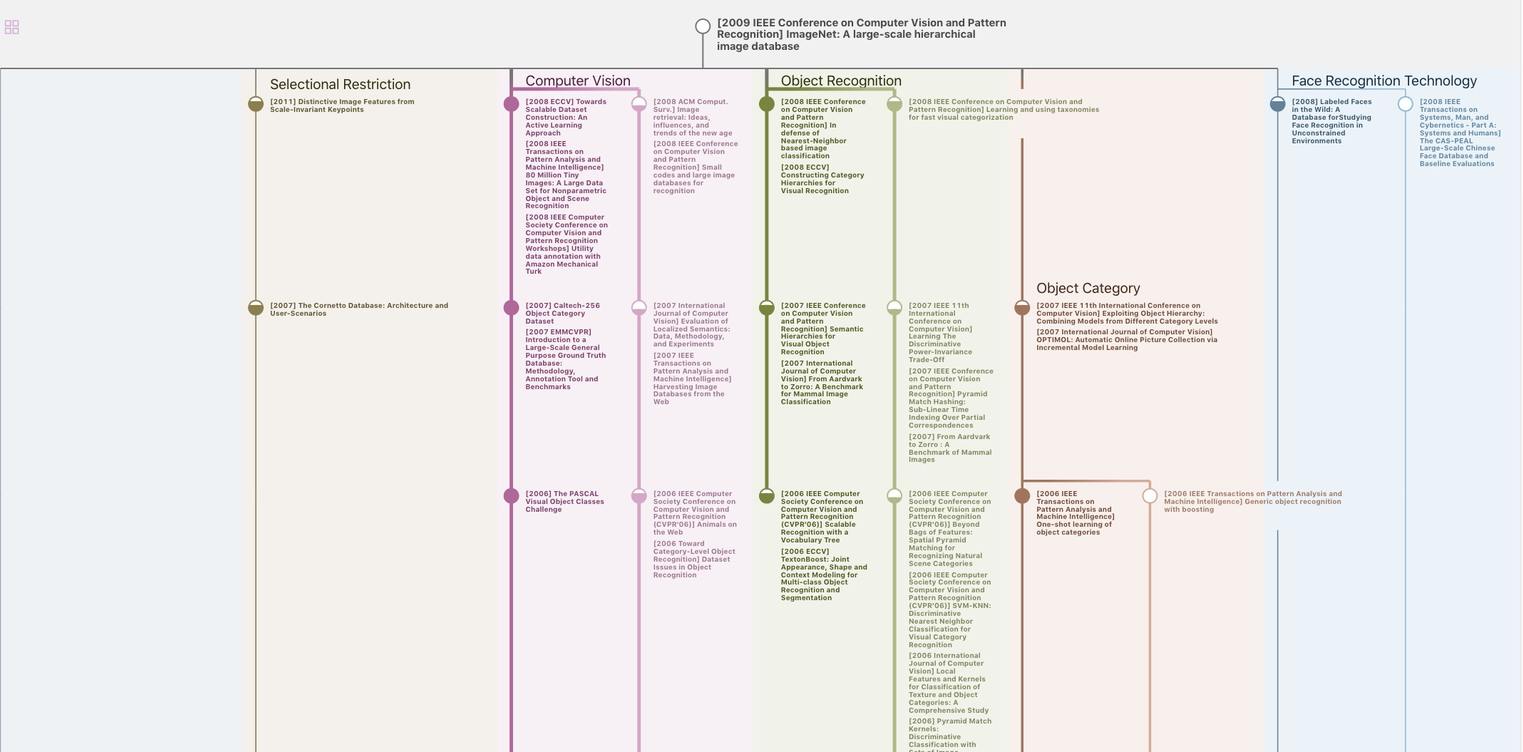
生成溯源树,研究论文发展脉络
Chat Paper
正在生成论文摘要