Deep Learning For Automatic Recognition Of Oil Production Related Objects Based On High-Resolution Remote Sensing Imagery
IGARSS 2020 - 2020 IEEE INTERNATIONAL GEOSCIENCE AND REMOTE SENSING SYMPOSIUM(2020)
摘要
Effectively monitoring the location and land use of oil production facilities and production emissions in the oil region is of great significance to the HSE management of oilfields. In the study, we construct a location-based Petroleum Remote Sensing dataset ( PetroRS dataset ) , which consist of 10 thousand labelled high-resolution images in two classes of oil production-related objects. After two distinct foul's of data augmentation, the dataset is enlarged 9 times. We applied Faster R-CNN, a deep learning method, to the PetroRS dataset to set up a preliminary result as the baseline. On this basis, we use the model to train the augmented dataset and improve the model by optimized anchor based on scale and aspect-ratio statistics. The results show: (1) Faster R-CNN model could detect two classes of oil production-related object automatically and simultaneously with the accuracy of 76% and 32%, respectively; (2) The model training with augmented dataset gives better result, more than 5% accuracy increments, compared to the baseline; (3) The improved model with optimized anchor returns a better result, more than 10% accuracy increments, compared to the baseline. We believe deep learning could provide a new practical and applicable idea in terms of applying remote sensing technology in the petroleum industry.
更多查看译文
关键词
deep learning, well site, mud pits, object detection, PetroRS Dataset, Faster R-CNN
AI 理解论文
溯源树
样例
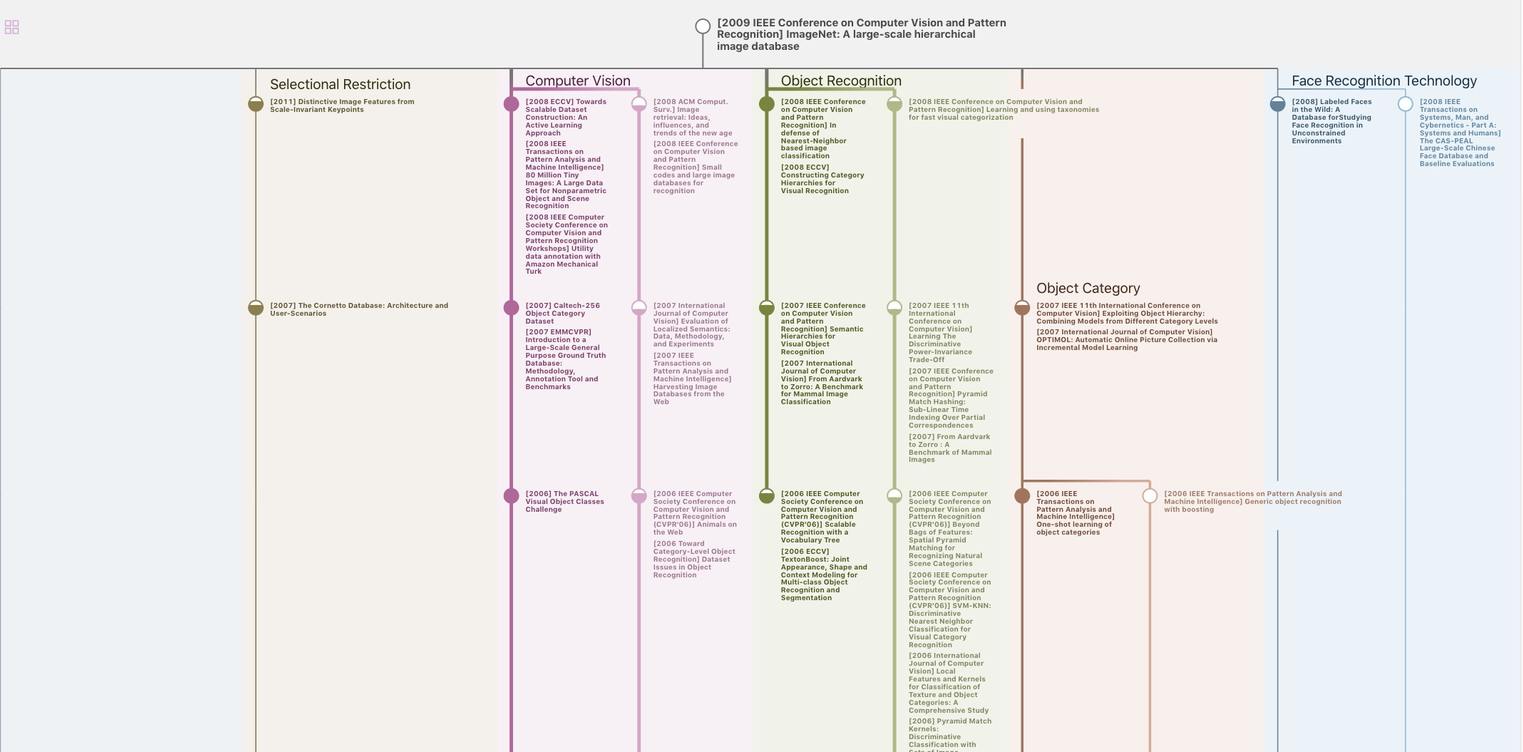
生成溯源树,研究论文发展脉络
Chat Paper
正在生成论文摘要