Predicting carbon and water vapor fluxes using machine learning and novel feature ranking algorithms
SCIENCE OF THE TOTAL ENVIRONMENT(2021)
摘要
Gap-filling eddy covariance flux data using quantitative approaches has increased over the past decade. Numerousmethods have been proposed previously, including look-up table approaches, parametricmethods, processbased models, and machine learning. Particularly, the REddyProc package from the Max Planck Institute for Biogeochemistry and ONEFlux package from AmeriFlux have been widely used in many studies. However, there is no consensus regarding the optimalmodel and feature selectionmethod that could be used for predicting different flux targets (Net Ecosystem Exchange, NEE; or Evapotranspiration -ET), due to the limited systematic comparative research based on the identical site-data. Here, we compared NEE and ET gap-filling/prediction performance of the least-square-based linear model, artificial neural network, random forest (RF), and support vector machine (SVM) using data obtained from four major row-crop and forage agroecosystems located in the subtropical or the climate-transition zones in the US. Additionally, we tested the impacts of different training-testing data partitioning settings, including a 10-fold time-series sequential (10FTS), a 10-fold cross validation (CV) routine with single data point (10FCV), daily (10FCVD), weekly ( 10FCVW) and monthly (10FCVM) gap length, and a 7/14-day flanking window(FW) approach; and implemented a novel Sliced Inverse Regression-based Recursive Feature Elimination algorithm (SIRRFE). We benchmarked the model performance against REddyProc and ONEFlux-produced results. Our results indicated that accurate NEE and ET prediction models could be systematically constructed using SVM/RF and only a few top informative features. The gapfilling performance of ONEFlux is generally satisfactory (R-2 = 0.39-0.71), but results from REddyProc could be very limited or even unreliable inmany cases (R-2= 0.01-0.67). Overall, SIRRFE-refined SVMmodels yielded excellent results for predicting NEE (R-2= 0.46-0.92) and ET (R-2= 0.74-0.91). Finally, the performance of various modelswas greatly affected by the types of ecosystem, predicting targets, and training algorithms; butwas insensitive towards training-testing partitioning. Our research provided more insights into constructing novel gapfilling models and understanding the underlying drivers affecting boundary layer carbon/water fluxes on an ecosystem level. (c) 2021 Elsevier B.V. All rights reserved.
更多查看译文
关键词
Eddy covariance,Machine learning,Feature ranking,Support vector machine,Remote sensing
AI 理解论文
溯源树
样例
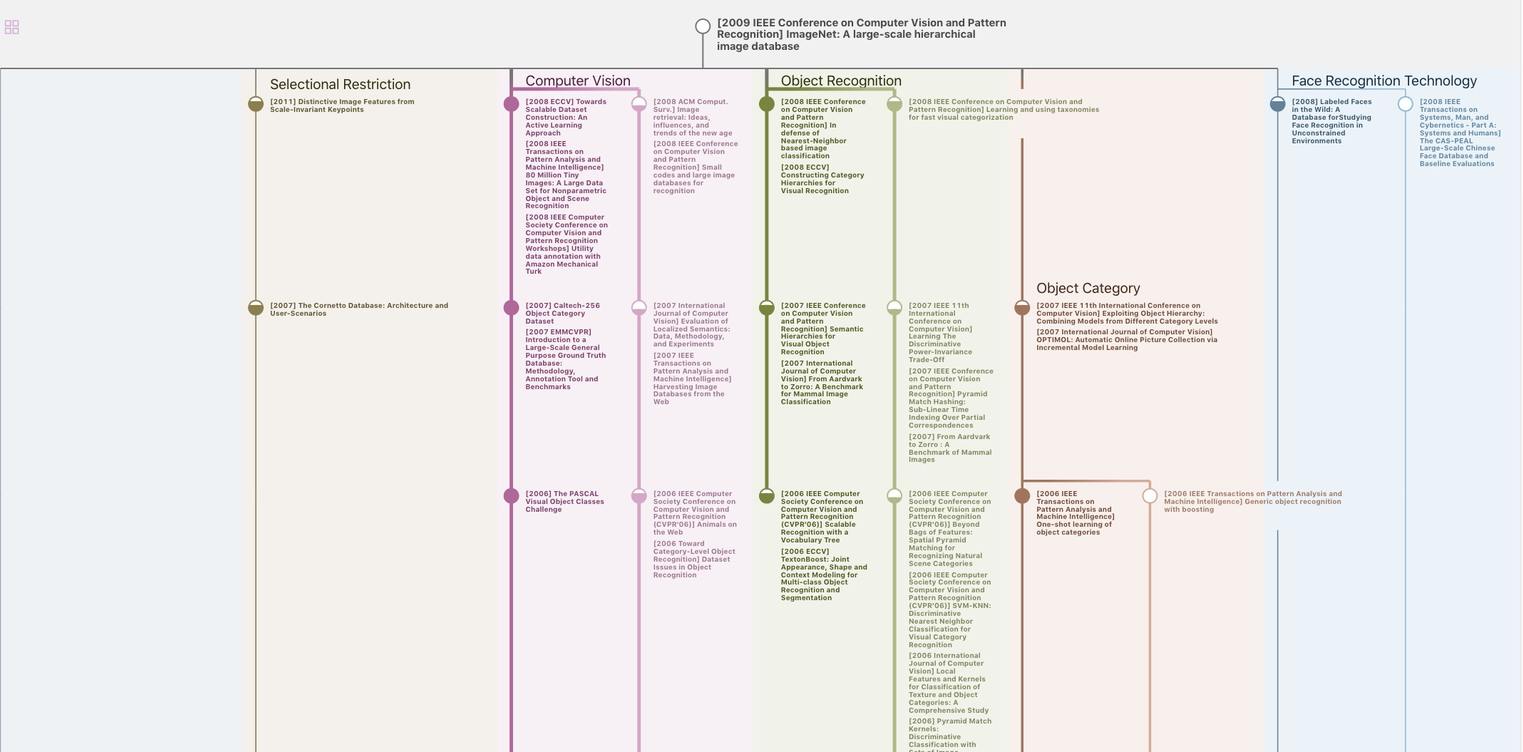
生成溯源树,研究论文发展脉络
Chat Paper
正在生成论文摘要