A Comparison Between Deep Learning Convolutional Neural Networks And Radiologists In The Differentiation Of Benign And Malignant Thyroid Nodules On Ct Images
ENDOKRYNOLOGIA POLSKA(2021)
摘要
Introduction: We designed 5 convolutional neural network (CNN) models and ensemble models to differentiate malignant and benign thyroid nodules on CT, and compared the diagnostic performance of CNN models with that of radiologists.Material and methods: We retrospectively included CT images of 880 patients with 986 thyroid nodules confirmed by surgical pathology between July 2017 and December 2019. Two radiologists retrospectively diagnosed benign and malignant thyroid nodules on CT images in a test set. Five CNNs (ResNet50, DenseNet121, DenseNet169, SE-ResNeXt50, and Xception) were trained-validated and tested using 788 and 198 thyroid nodule CT images, respectively. Then, we selected the 3 models with the best diagnostic performance on the test set for the model ensemble. We then compared the diagnostic performance of 2 radiologists with 5 CNN models and the integrated model.Results: Of the 986 thyroid nodules, 541 were malignant, and 445 were benign. The area under the curves (AUCs) for diagnosing thyroid malignancy was 0.587-0.754 for 2 radiologists. The AUCs for diagnosing thyroid malignancy for the 5 CNN models and ensemble model was 0.901-0.947. There were significant differences in AUC between the radiologists' models and the CNN models (p < 0.05). The ensemble model had the highest AUC value.Conclusions: Five CNN models and an ensemble model performed better than radiologists in distinguishing malignant thyroid nodules from benign nodules on CT. The diagnostic performance of the ensemble model improved and showed good potential.
更多查看译文
关键词
deep learning, convolutional neural network (CNN), thyroid nodule classification, computed tomography (CT)
AI 理解论文
溯源树
样例
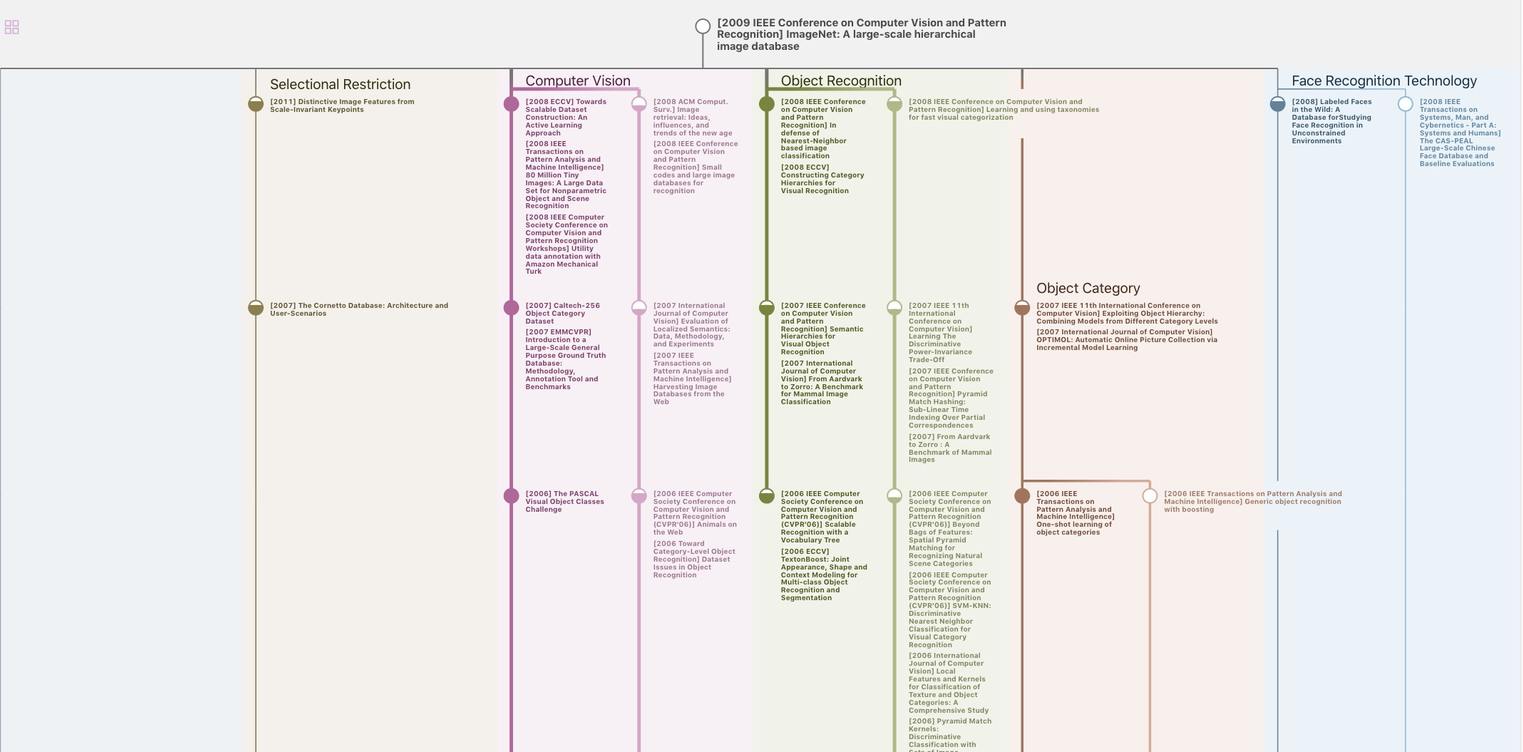
生成溯源树,研究论文发展脉络
Chat Paper
正在生成论文摘要