A Maximum-Likelihood TDOA Localization Algorithm Using Difference-of-Convex Programming
IEEE SIGNAL PROCESSING LETTERS(2021)
Abstract
A popular approach to estimate a source location using time difference of arrival (TDOA) measurements is to construct an objective function based on the maximum likelihood (ML) method. An iterative algorithm can be employed to minimize that objective function. The main challenge in this optimization process is the non-convexity of the objective function, which precludes the use of many standard convex optimization tools. Usually, approximations, such as convex relaxation, are applied, resulting in performance loss. In this work, we take advantage of difference-of-convex (DC) programming tools to develop an efficient solution to the ML TDOA localization problem. We show that, by using a simple trick, the objective function can be modified into an exact difference of two convex functions. Hence, tools from DC programming can be leveraged to carry out the optimization task, which guarantees convergence to a stationary point of the objective function. Simulation results show that, when initialized within the convex hull of the anchors, the proposed TDOA localization algorithm outperforms a number of benchmark methods, behaves as an exact ML estimator, and indeed achieves the Cramer-Rao lower bound.
MoreTranslated text
Key words
Linear programming,Location awareness,Convex functions,Maximum likelihood estimation,Programming,Position measurement,Convergence,Localization,TDOA,non-convex,optimization,CCCP,DC programming,difference of convex,convex-concave
AI Read Science
Must-Reading Tree
Example
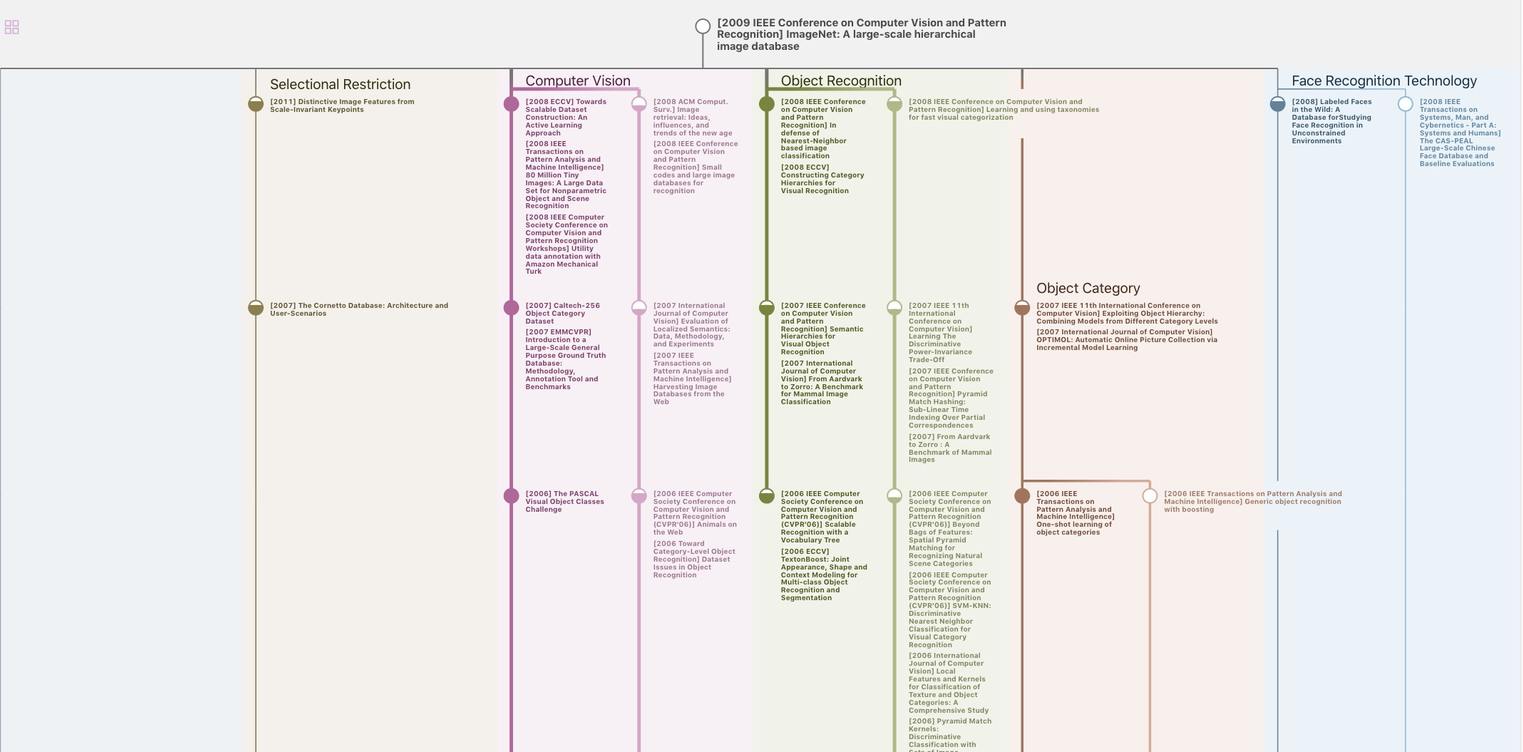
Generate MRT to find the research sequence of this paper
Chat Paper
Summary is being generated by the instructions you defined