Multilabel Classification Model for Type Recognition of Single-Phase-to-Ground Fault Based on KNN-Bayesian Method
IEEE Transactions on Industry Applications(2021)
摘要
In nonsolidly earthed distribution networks, single-phase-to-ground faults (SPGFs) significantly threaten the safety of people and equipment. Although selection and location techniques for the existing fault lines have made remarkable contributions in reducing the damage due to SPGFs, a certain amount of power loss still exists in the SPGF owing to its low efficiency in detection and maintenance. Multiple-dimensional classification of the SPGF May help reveal the nature of the fault from different perspectives; therefore, in this article, a multilabel classification model for recognizing the types of SPGF is proposed. In the proposed model, the SPGF is classified considering five dimensions, namely time-domain continuity, time-domain stability, volt-ampere characteristics of transition impedance, transition impedance size, and fault point medium. Subsequently, the corresponding features are determined. In addition, a multilabel classification model of the SPGF is constructed with an 8-D feature space and a 14-label fault-type space. Finally, a k-nearest neighbors Bayesian method is designed to solve the multilabel classification problem. The feasibility and advantages of the proposed model and methods are verified using field data and through comparison with the KNN method.
更多查看译文
关键词
K-Nearest Neighbors-Bayesian,multidimens-ional features,multilabel classification,single-phase-to-ground fault (SPGF),type recognition
AI 理解论文
溯源树
样例
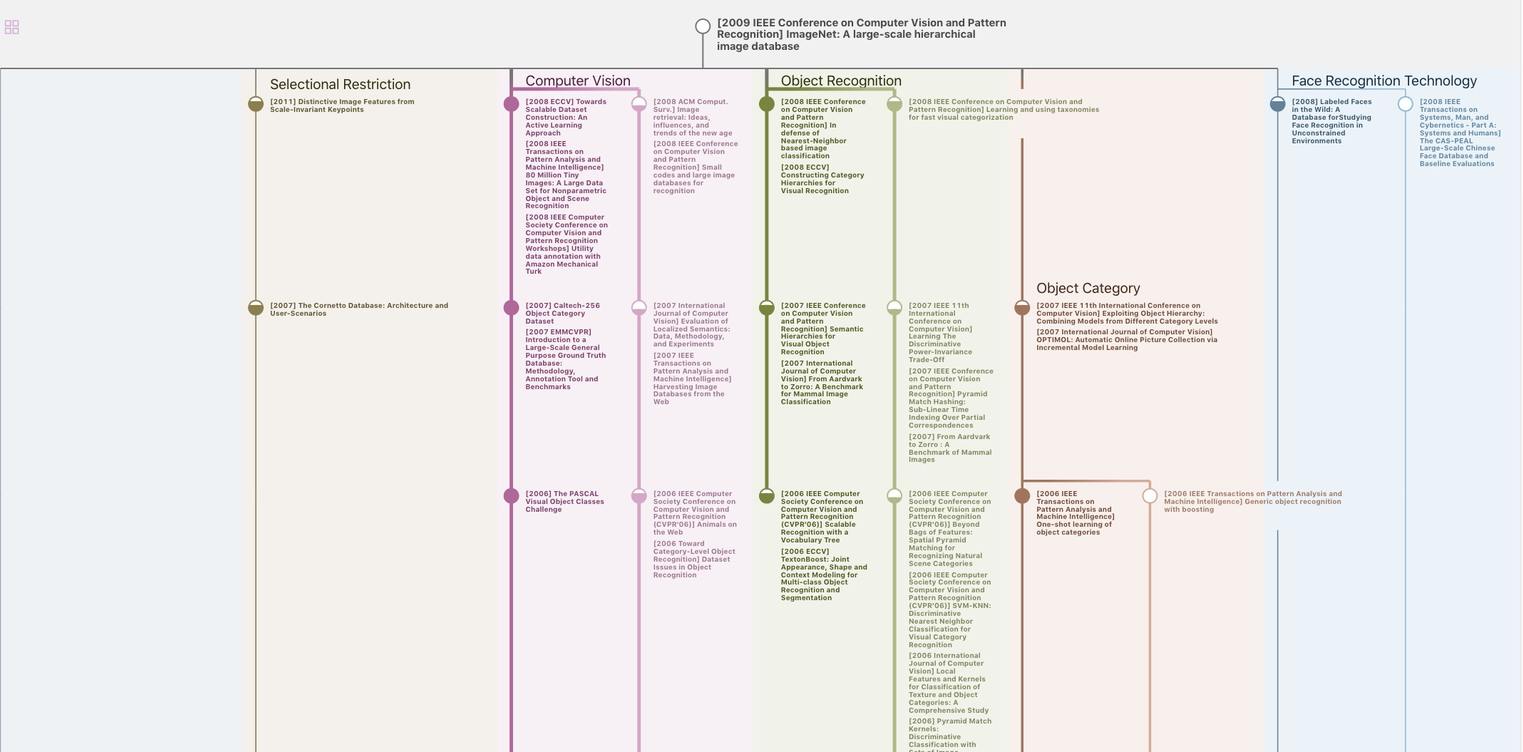
生成溯源树,研究论文发展脉络
Chat Paper
正在生成论文摘要