Improving Human Robot Collaboration Through Force/Torque Based Learning For Object Manipulation
ROBOTICS AND COMPUTER-INTEGRATED MANUFACTURING(2021)
摘要
Human-Robot Collaboration (HRC) is a term used to describe tasks in which robots and humans work together to achieve a goal. Unlike traditional industrial robots, collaborative robots need to be adaptive; able to alter their approach to better suit the situation and the needs of the human partner. As traditional programming techniques can struggle with the complexity required, an emerging approach is to learn a skill by observing human demonstration and imitating the motions; commonly known as Learning from Demonstration (LfD). In this work, we present a LfD methodology that combines an ensemble machine learning algorithm (i.e. Random Forest (RF)) with stochastic regression, using haptic information captured from human demonstration. The capabilities of the proposed method are evaluated using two collaborative tasks; co-manipulation of an object (where the human provides the guidance but the robot handles the objects weight) and collaborative assembly of simple interlocking parts. The proposed method is shown to be capable of imitation learning; interpreting human actions and producing equivalent robot motion across a diverse range of initial and final conditions. After verifying that ensemble machine learning can be utilised for real robotics problems, we propose a further extension utilising Weighted Random Forest (WRF) that attaches weights to each tree based on its performance. It is then shown that the WRF approach outperforms RF in HRC tasks.
更多查看译文
关键词
Imitation learning, Human-Robot Collaboration, Random Forests regression, Gaussian mixture regression and ensemble-learning
AI 理解论文
溯源树
样例
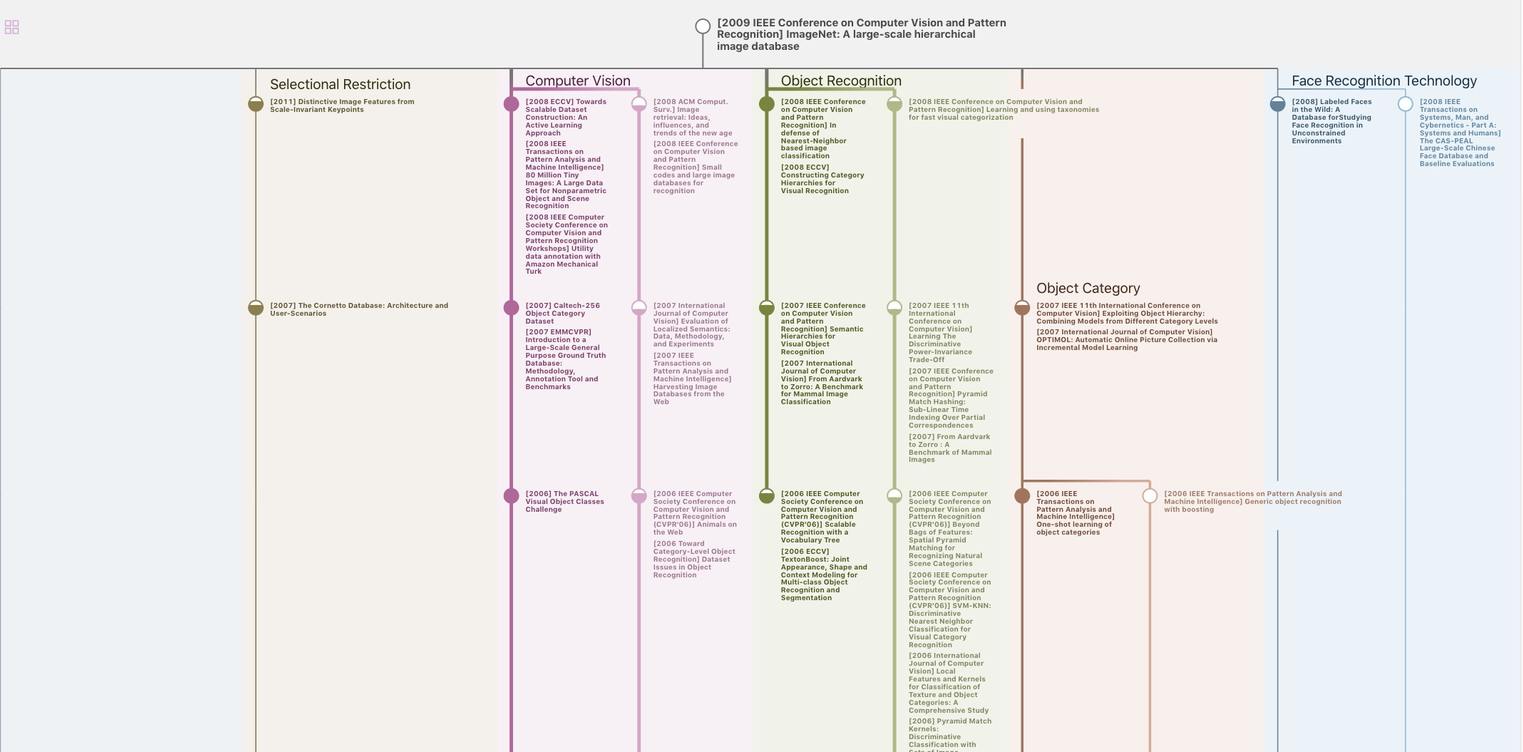
生成溯源树,研究论文发展脉络
Chat Paper
正在生成论文摘要