Machine learning for rediscovering revolutionary ideas of the past
ADAPTIVE BEHAVIOR(2022)
摘要
The explosion of online knowledge has made knowledge, paradoxically, difficult to find. A web or journal search might retrieve thousands of articles, ranked in a manner that is biased by, for example, popularity or eigenvalue centrality rather than by informed relevance to the complex query. With hundreds of thousands of articles published each year, the dense, tangled thicket of knowledge grows even more entwined. Although natural language processing and new methods of generating knowledge graphs can extract increasingly high-level interpretations from research articles, the results are inevitably biased toward recent, popular, and/or prestigious sources. This is a result of the inherent nature of human social-learning processes. To preserve and even rediscover lost scientific ideas, we employ the theory that scientific progress is punctuated by means of inspired, revolutionary ideas at the origin of new paradigms. Using a brief case example, we suggest how phylogenetic inference might be used to rediscover potentially useful lost discoveries, as a way in which machines could help drive revolutionary science.
更多查看译文
关键词
Artificial intelligence, machine learning, phylogenetic analysis, scientific revolution, social learning
AI 理解论文
溯源树
样例
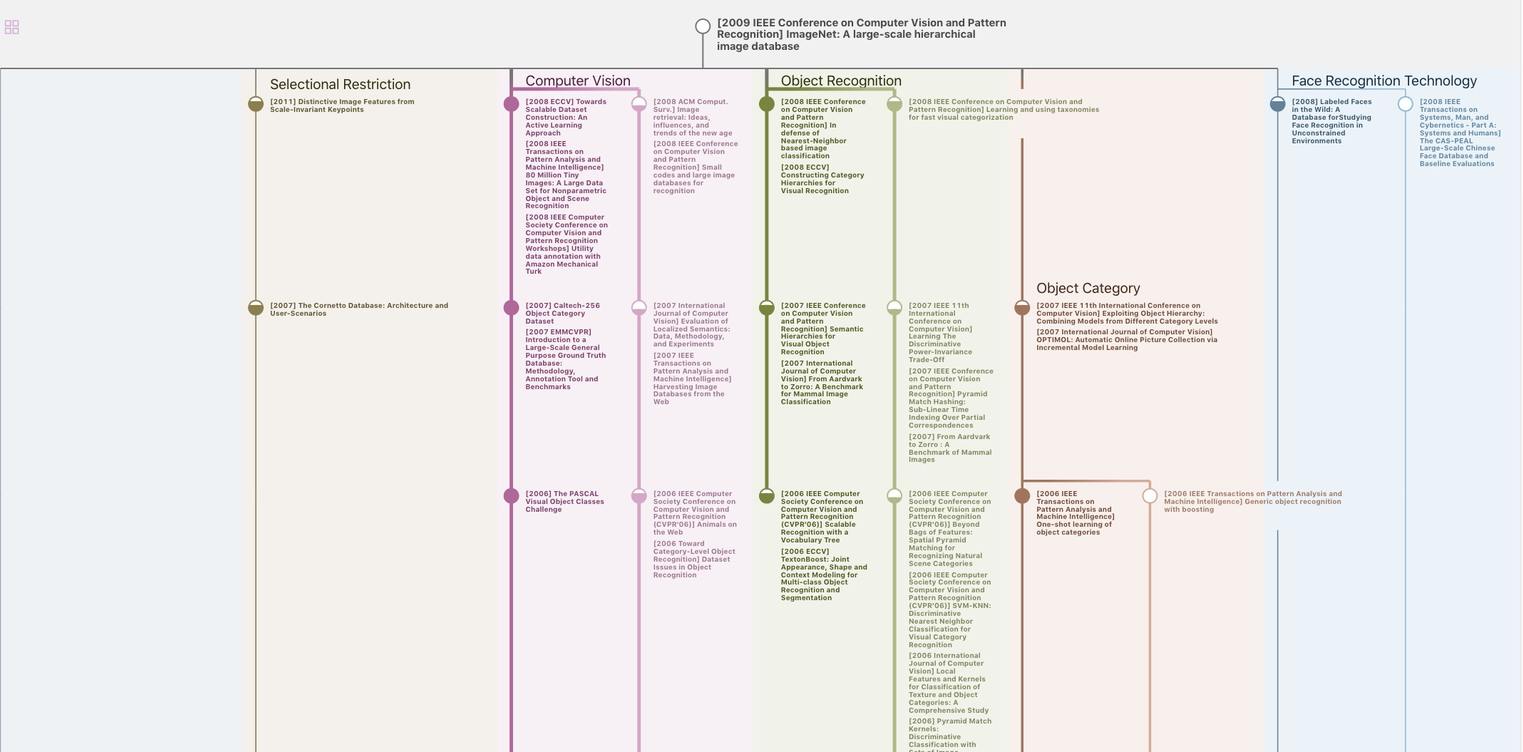
生成溯源树,研究论文发展脉络
Chat Paper
正在生成论文摘要