Object Reconstruction Based on Attentive Recurrent Network from Single and Multiple Images
NEURAL PROCESSING LETTERS(2021)
摘要
The application of traditional 3D reconstruction methods such as structure-from-motion and simultaneous localization and mapping are typically limited by illumination conditions, surface textures, and wide baseline viewpoints in the field of robotics. To solve this problem, many researchers have applied learning-based methods with convolutional neural network architectures. However, simply utilizing convolutional neural networks without taking other measures into account is computationally intensive, and the results are not satisfying. In this study, to obtain the most informative images for reconstruction, we introduce a residual block to a 2D encoder for improved feature extraction, and propose an attentive latent unit that makes it possible to select the most informative image being fed into the network rather than choosing one at random. The recurrent visual attentive network is injected into the auto-encoder network using reinforcement learning. The recurrent visual attentive network pays more attention to useful images, and the agent will quickly predict the 3D volume. This model is evaluated based on both single- and multi-view reconstructions. The experiment results show that the recurrent visual attentive network increases prediction performance in a way that is superior to other alternative methods, and our model has desirable capacity for generalization.
更多查看译文
关键词
Object reconstruction,Convolutional LSTM,Visual attention,Robotic application
AI 理解论文
溯源树
样例
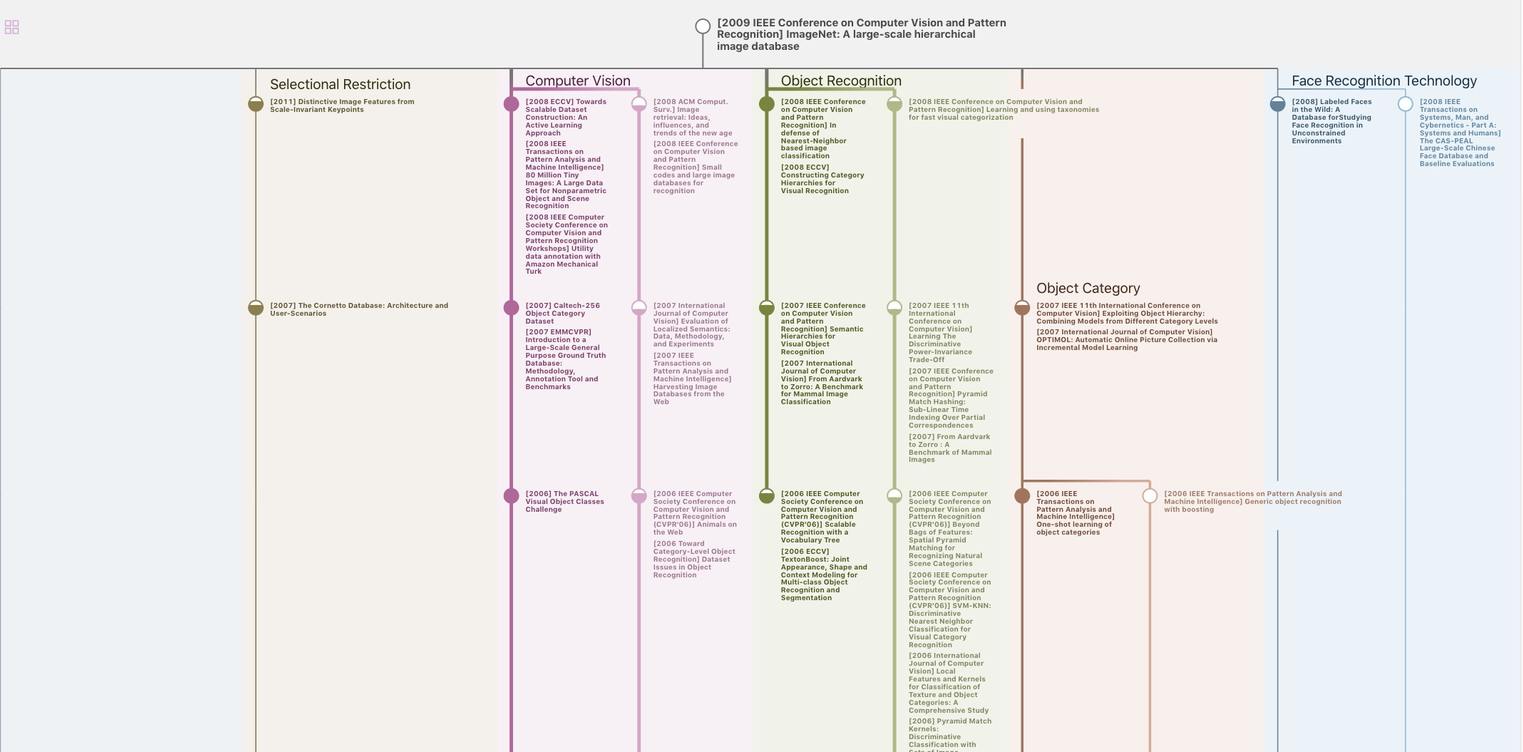
生成溯源树,研究论文发展脉络
Chat Paper
正在生成论文摘要