Spontaneous Temporal Grouping Neural Network for Long-Term Memory Modeling
IEEE transactions on cognitive and developmental systems(2022)
摘要
The capacity of long-term memory is an important issue in sequence learning, but it remains challenging for the problems of vanishing gradients or out-of-order dependencies. Inspired by human memory, in which long-term memory is broken into fragments and then can be recalled at appropriate times, we propose a neural network via spontaneous temporal grouping in this article. In the architecture, the segmented layer is used for spontaneous sequence segmentation under guidance of the reset gates which are driven to be sparse in the training process; the cascading layer is used to collect information from the temporal groups, where a filtered long short-term memory with chrono-initialization is proposed to alleviate the gradient vanishing phenomenon, and random skip connections are adopted to capture complex dependencies among the groups. Furthermore, the advantage of our neural architecture in long-term memory is demonstrated via a new measurement method. In experiments, we compare the performance with multiple models on several algorithmic or classification tasks, and both of the sequences with fixed lengths like the MNISTs and with varying lengths like the speech utterances are adopted. The results in different criteria have demonstrated the superiority of our proposed neural network.
更多查看译文
关键词
Computer architecture,Logic gates,Microprocessors,Training,Standards,Data models,Task analysis,Long-term memory,recurrent neural network,temporal dependency,temporal grouping,vanishing gradient
AI 理解论文
溯源树
样例
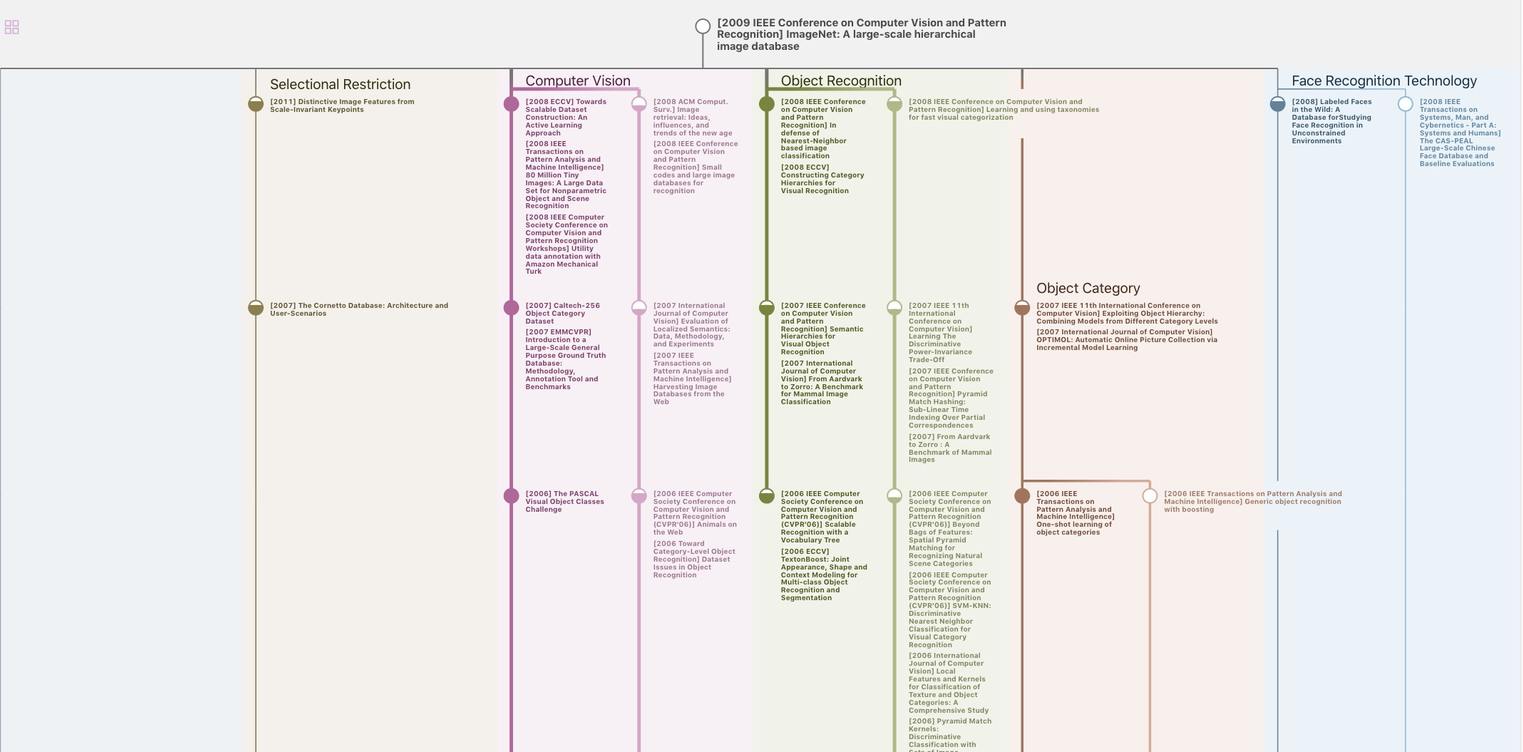
生成溯源树,研究论文发展脉络
Chat Paper
正在生成论文摘要