Detecting Cyber Attack Under Quantitative Impact of Demand Side Management
ieee/pes transmission and distribution conference and exposition(2020)
摘要
Cyber enabled Demand Side management (DSM) plays an increasing role in modern power grid operations, in particular, to improve power balance and grid stability. The cyber communication that enables DSM, however, is vulnerable to attacks that can impact significantly the distribution grid functioning. This work investigates the detection of false data injection into the DSM communication loop by adapting supervised learning models to capture the demand data and both parametric and non parametric detectors to identify intrusion in real time. Specifically, the seasonal autoregressive integrated moving average (SARIMA) time series forecast models is utilized to capture the demand data and subsequently an elasticity model is adapted for the price-demand relationship in the DSM loop. Two kinds of detection mechanisms are considered to detect a cyber attack in this interactive system, a parametric detector that models residuals from SARIMA forecasts as Gaussian and uses a generalized likelihood ratio test (GLRT) and a non parametric detector that uses a cumulative sum (CUSUM) based statistical deviations test on the raw data. Several inferences are drawn on the performance of these mechanisms as functions of user pool, DSM participation rate and attack magnitude. Notably, the non parametric detection outperforms the parametric detector for smaller user pools and a higher penetration of DSM enables better detection of cyber attacks.
更多查看译文
关键词
Demand Side Management,SARIMA,Price-demand Relationship,GLRT,Nonparametric Detector
AI 理解论文
溯源树
样例
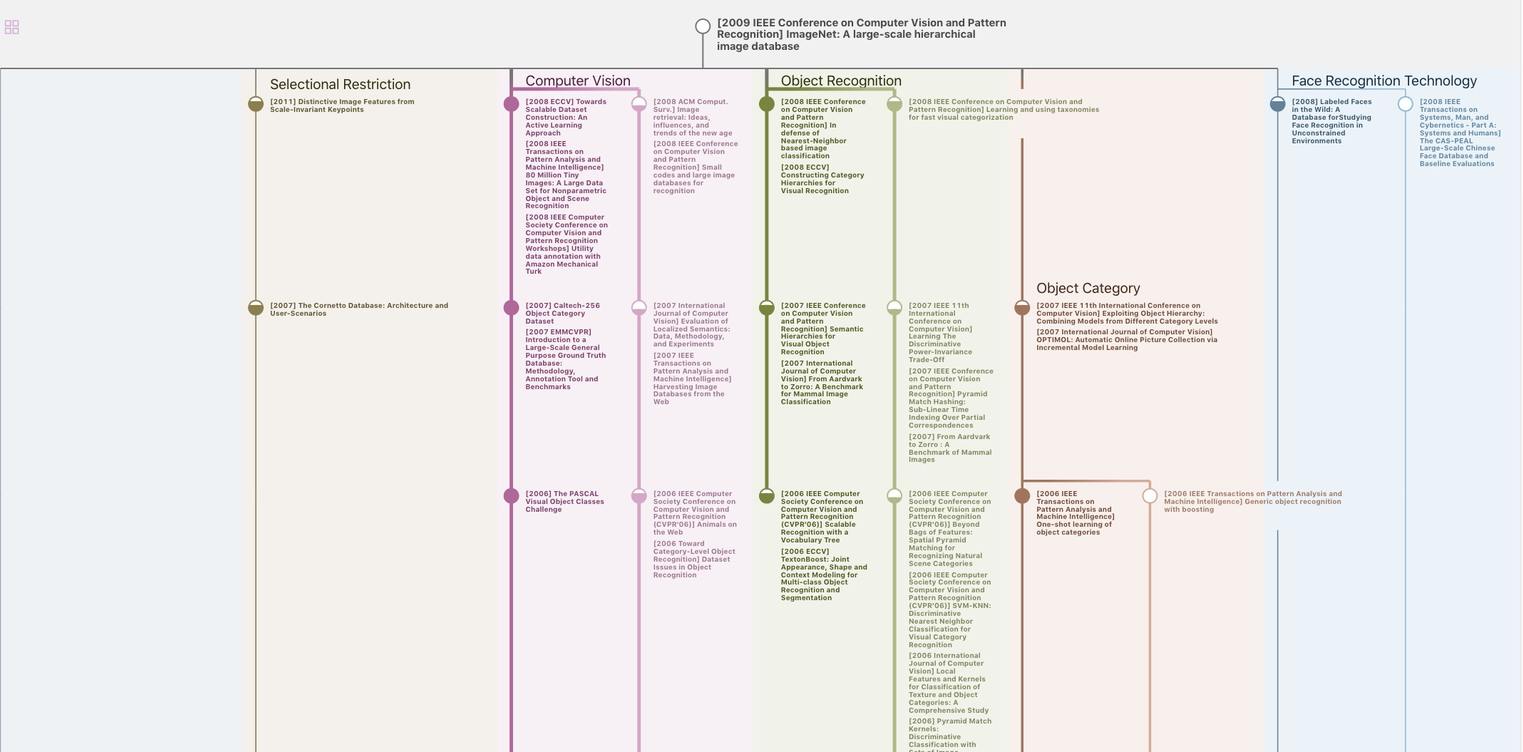
生成溯源树,研究论文发展脉络
Chat Paper
正在生成论文摘要