Parameter estimation for diffusion process from perturbed discrete observations
Communications in Statistics - Simulation and Computation(2023)
摘要
We study the parameter estimation for ergodic diffusion process X-t from perturbed observations Y-tj = X-tj + epsilon(tj), j = 1, ... , n where t(1), ... , t(n) are observation times and the noise et is a strongly mixing stationary noisy process with the density function g. We construct an estimator of the diffusion parameters based on the minimum Hellinger distance between the density of the invariant distribution of diffusion process Xt and the nonparametric deconvolution kernel estimator of this density. This article focuses on the ordinary smooth noise density class with the assumption A(1)(1 + vertical bar p vertical bar)(-kappa) <= vertical bar g(ft)(p)vertical bar <= A(2)(1 + vertical bar p vertical bar)(-kappa) (where kappa > 0 and g(ft)(p) is characteristic function of noisy random variable). This assumption is more general than the condition lim(p ->infinity) g(ft)(p) . p(kappa) = C (where C is a constant) which is used in a lot of articles. We also discuss the asymptotic normality for both the estimator of deconvolution kernel density and the estimator of diffusion parameters. Finally, we illustrate the properties of the estimator by two examples of diffusion processes.
更多查看译文
关键词
Asymptotic normality,deconvolution,estimator,strongly mixing processes
AI 理解论文
溯源树
样例
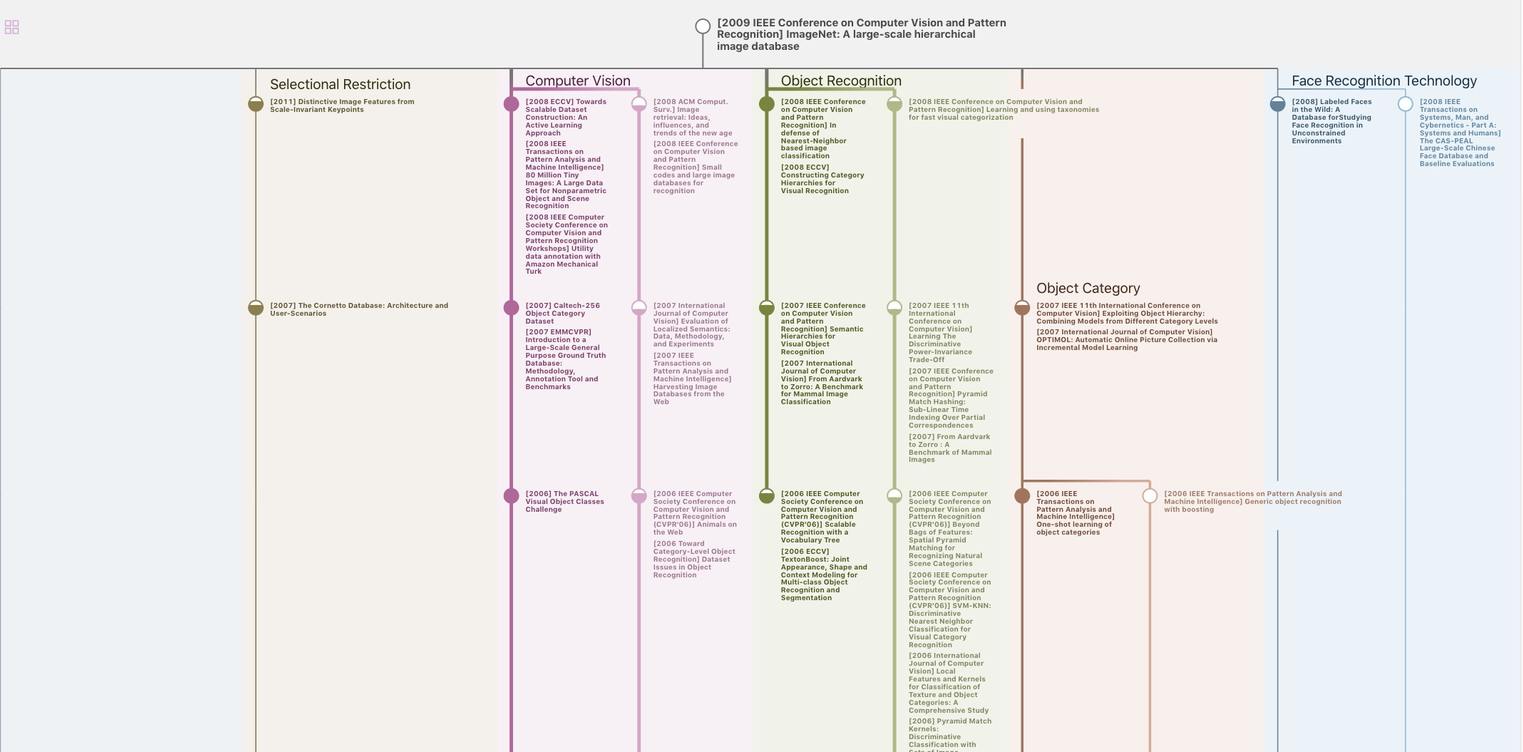
生成溯源树,研究论文发展脉络
Chat Paper
正在生成论文摘要