Unsupervised feature selection via transformed auto-encoder
Knowledge-Based Systems(2021)
摘要
As one of the fundamental research issues, feature selection plays a critical role in machine learning. By the removal of irrelevant features, it attempts to reduce computational complexities of upstream tasks, usually with computation accelerations and performance improvements. This paper proposes an auto-encoder based scheme for unsupervised feature selection. Due to the inherent consistency, this framework can solve traditional constrained feature selection problems approximately. Specifically, the proposed model takes non-negativity, orthogonality, and sparsity into account, whose internal characteristics are exploited sufficiently. It can also employ other loss functions and flexible activation functions. The former can fit a wide range of learning tasks, and the latter has the ability to play the role of regularization terms to impose regularization constraints on the model. Thereinafter, the proposed model is validated on multiple benchmark datasets, where various activation and loss functions are analyzed for finding better feature selectors. Finally, extensive experiments demonstrate the superiority of the proposed method against other compared state-of-the-arts.
更多查看译文
关键词
Machine learning,Deep learning,Feature selection,Unsupervised learning,Auto-encoder
AI 理解论文
溯源树
样例
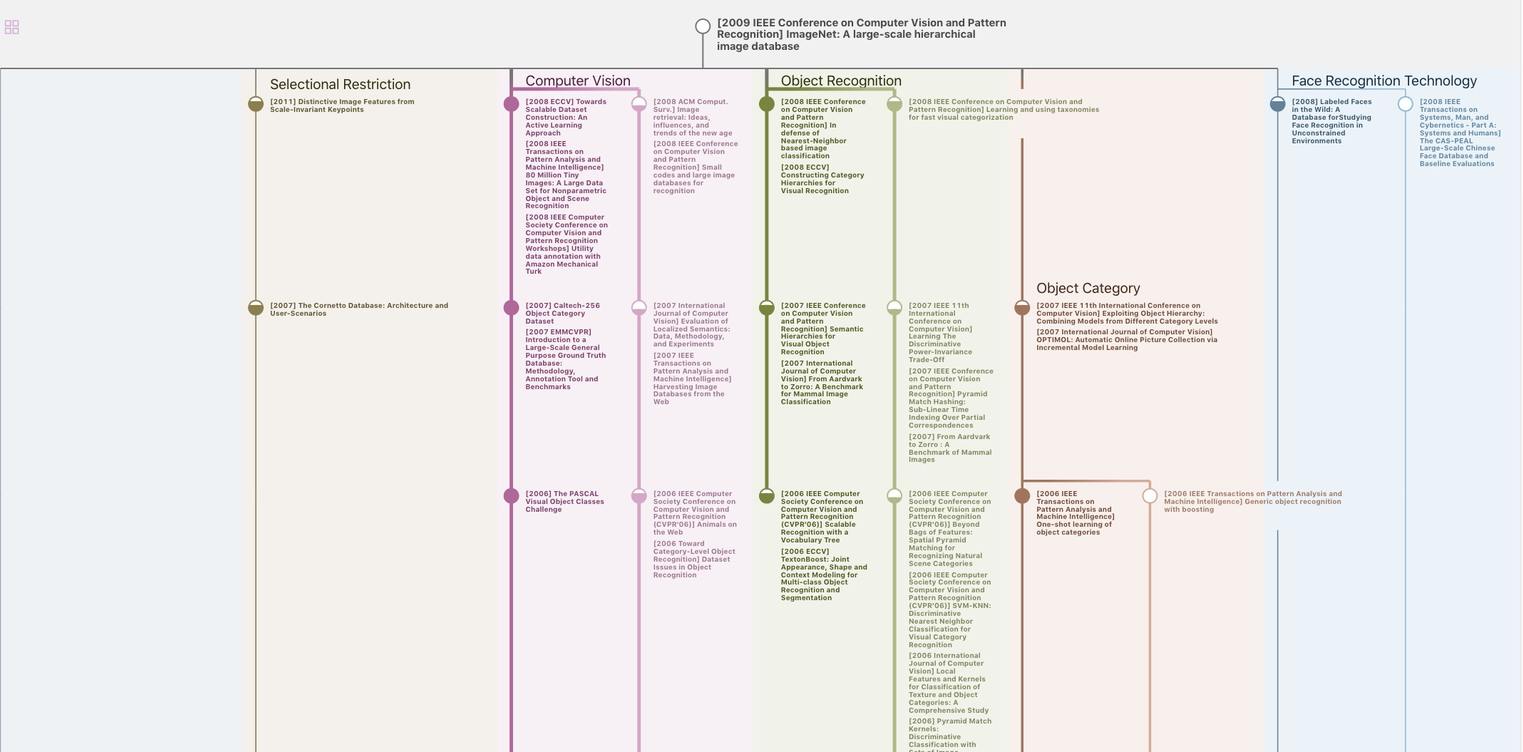
生成溯源树,研究论文发展脉络
Chat Paper
正在生成论文摘要