Simultaneous Random Number Generation and Optical Tweezers Calibration Employing a Learning Algorithm Based on the Brownian Dynamics of a Trapped Colloidal Particle
Frontiers in physics(2021)
摘要
True random number generators are in high demand for secure cryptographic algorithms. Unlike algorithmically generated pseudo-random numbers they are unclonable and non-deterministic. A particle following Brownian dynamics as a result of the stochastic Ornstein-Uhlenbeck process is a source of true randomness because the collisions with the ambient molecules are probabilistic in nature. In this paper, we trap colloidal particles in water using optical tweezers and record its confined Brownian motion in real-time. Using a segment of the initial incoming data we train our learning algorithm to measure the values of the trap stiffness and diffusion coefficient and later use those parameters to extract the "white" noise term in the Langevin equation. The random noise is temporally delta correlated, with a flat spectrum. We use these properties in an inverse problem of trap-calibration to extract trap stiffnesses, compare it with standard equipartition of energy technique, and show it to scale linearly with the power of the trapping laser. Interestingly, we get the best random number sequence for the best calibration. We test the random number sequence, which we have obtained, using standard tests of randomness and observe the randomness to improve with increasing sampling frequencies. This method can be extended to the trap-calibration for colloidal particles confined in complex fluids, or active particles in simple or complex environments so as to provide a new and accurate analytical methodology for studying Brownian motion dynamics using the newly-emerged but robust machine learning platform.
更多查看译文
关键词
true random numbers,supervised learning,optical trap calibration,autoregresison,time series analysis
AI 理解论文
溯源树
样例
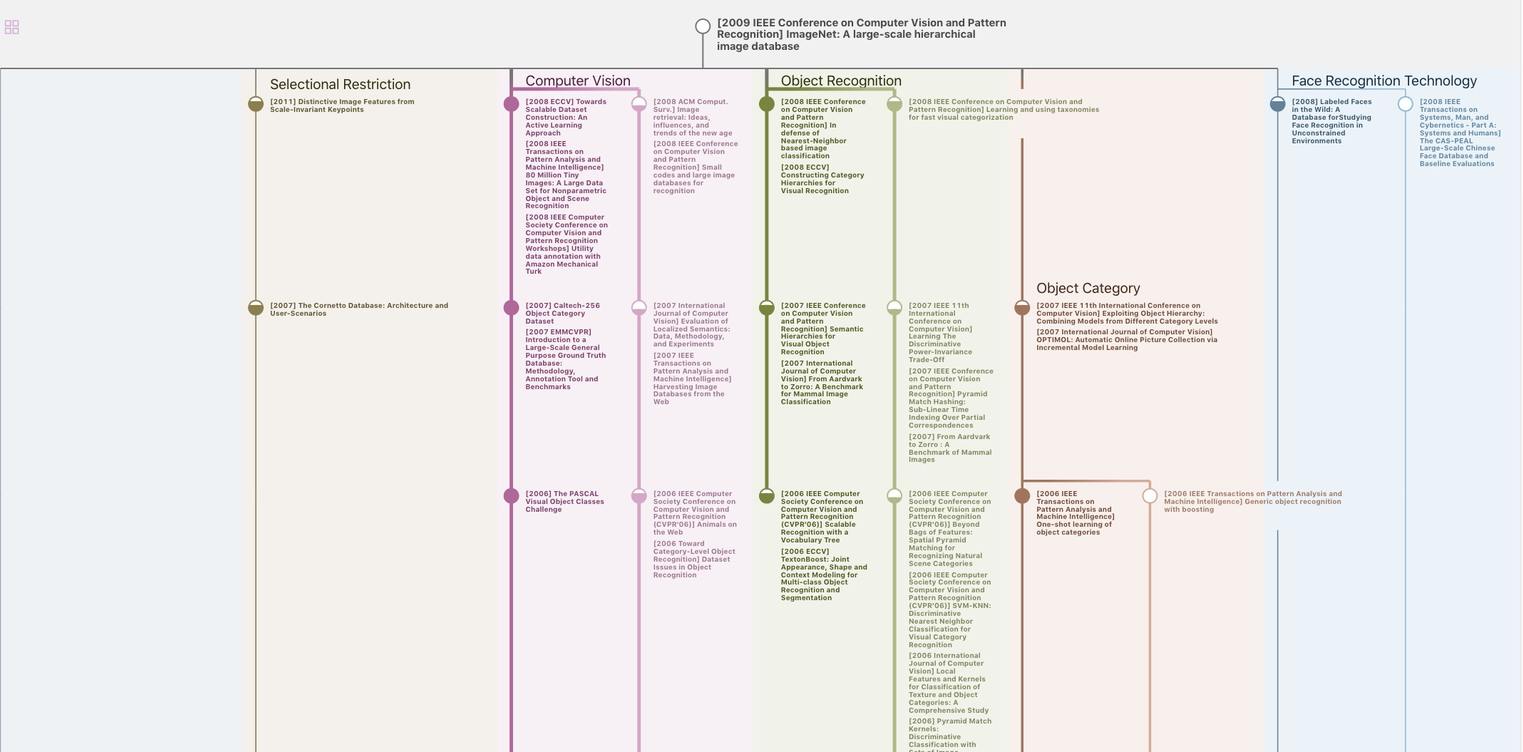
生成溯源树,研究论文发展脉络
Chat Paper
正在生成论文摘要