Offensive Security Of Keyboard Data Using Machine Learning For Password Authentication In Iot
IEEE ACCESS(2021)
摘要
In this paper, to increase the attack success rate, we analyzed the distribution of all collected keyboard data based on the distance of time and keyboard scancode data, which presents the crucial data from the previous study. To achieve this, we derived time-distance based features that have higher attack success rates than in previous studies. The proposed attack method defines 6 features, and evaluates the performance based on 18 datasets. For performance evaluation, the accuracy, precision, recall, F1-score, and AUC of Datasets (1 to 3) were compared, and two experiments showed improved overall performance by at least 10.6 % and up to 16.1 % compared to previous studies in terms of the performance evaluation for each feature, comparison of variations in maximum performance, comparison of variations in performance of each feature, and comparison of variations in overall performance. Moreover, the best accuracy, which represents the probability of password exposure, was 96.7 %, which suggests that our proposed attack method has a higher accuracy than the previous study (96.2 %). In conclusion, we demonstrated that password authentication is neutralized by stealing the user password more effectively. For future research, we will focus on improving the attack success rate with respect to accuracy and overall performance numbers, using not only machine learning, but also deep learning.
更多查看译文
关键词
Keyboards, Authentication, Servers, Password, Smart homes, Tools, Security, Offensive security, vulnerability analysis, password authentication, user authentication, machine learning
AI 理解论文
溯源树
样例
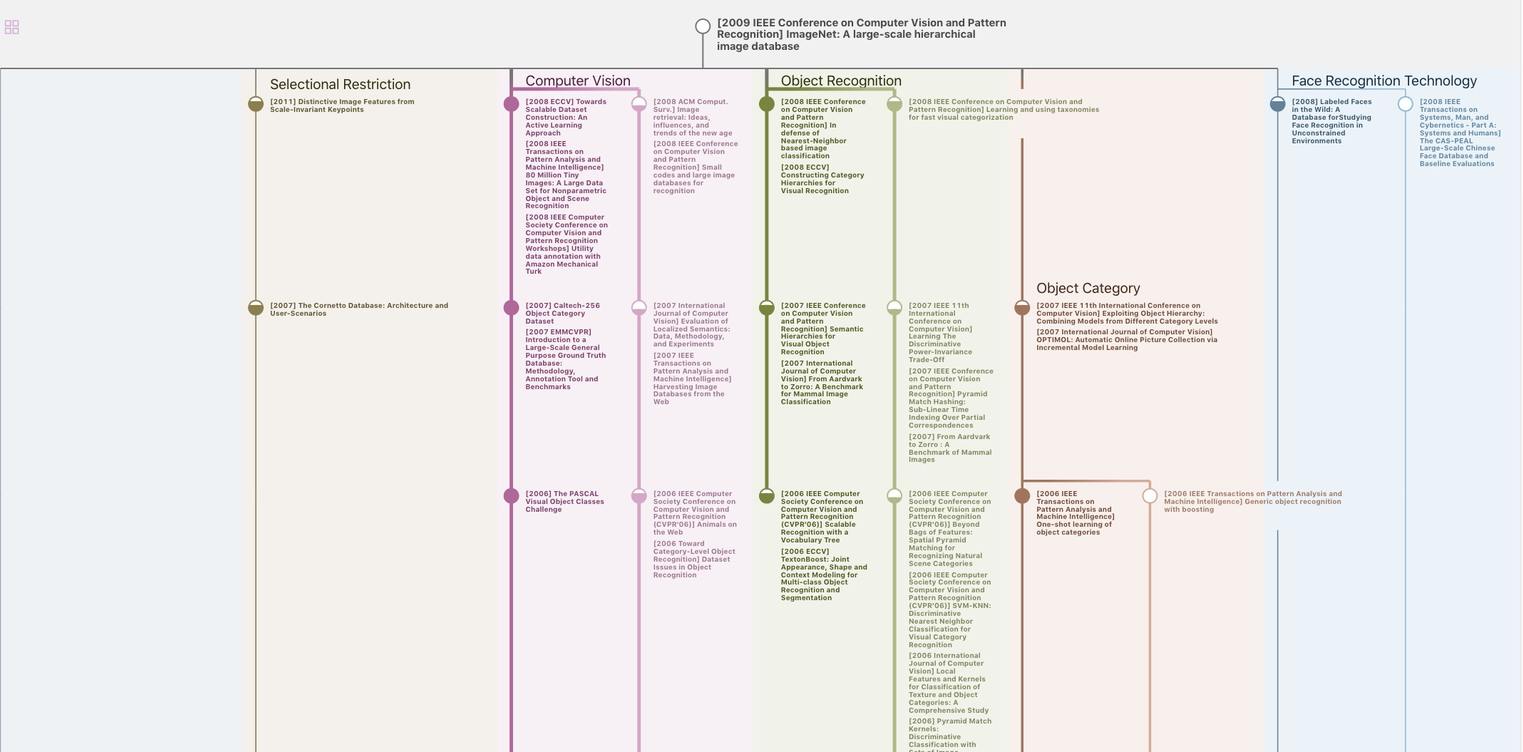
生成溯源树,研究论文发展脉络
Chat Paper
正在生成论文摘要