Metamodel-Based Reliability Analysis In Spatially Variable Soils Using Convolutional Neural Networks
JOURNAL OF GEOTECHNICAL AND GEOENVIRONMENTAL ENGINEERING(2021)
摘要
In recent years, the random field finite-element method (FEM) has been used increasingly in geotechnical engineering to carry out analyses that account for the inherent spatial variability in the physical and mechanical properties of both natural and treated soils. However, this method, which usually is performed in tandem with Monte Carlo simulation (MCS), requires significantly greater computational resources than deterministic finite-element analysis. Metamodeling is one of the techniques commonly adopted to alleviate the computational burden. This paper proposes a novel and computationally efficient metamodeling technique that involves the use of convolutional neural networks (CNNs) to perform random field finite-element analyses. CNNs, which treat random fields as images, are capable of outputting FEM predicted quantities with learned high-level features that contain information about the random variabilities in both spatial distribution and intensity. CNNs, after being trained with sufficient random field samples, could be used as a metamodel to replace the expensive random field finite-element simulations for all subsequent calculations. The validity of the proposed approach was illustrated using a synthetic excavation problem and a synthetic surface footing problem. The good agreement between the CNN outputs and the FEM predictions demonstrated the promising potential of using CNNs as a metamodel for reliability analysis in spatially variable soils. (c) 2021 American Society of Civil Engineers.
更多查看译文
关键词
Convolutional neural networks, Machine learning, Spatial variability, Metamodel, Random field, Probability of failure
AI 理解论文
溯源树
样例
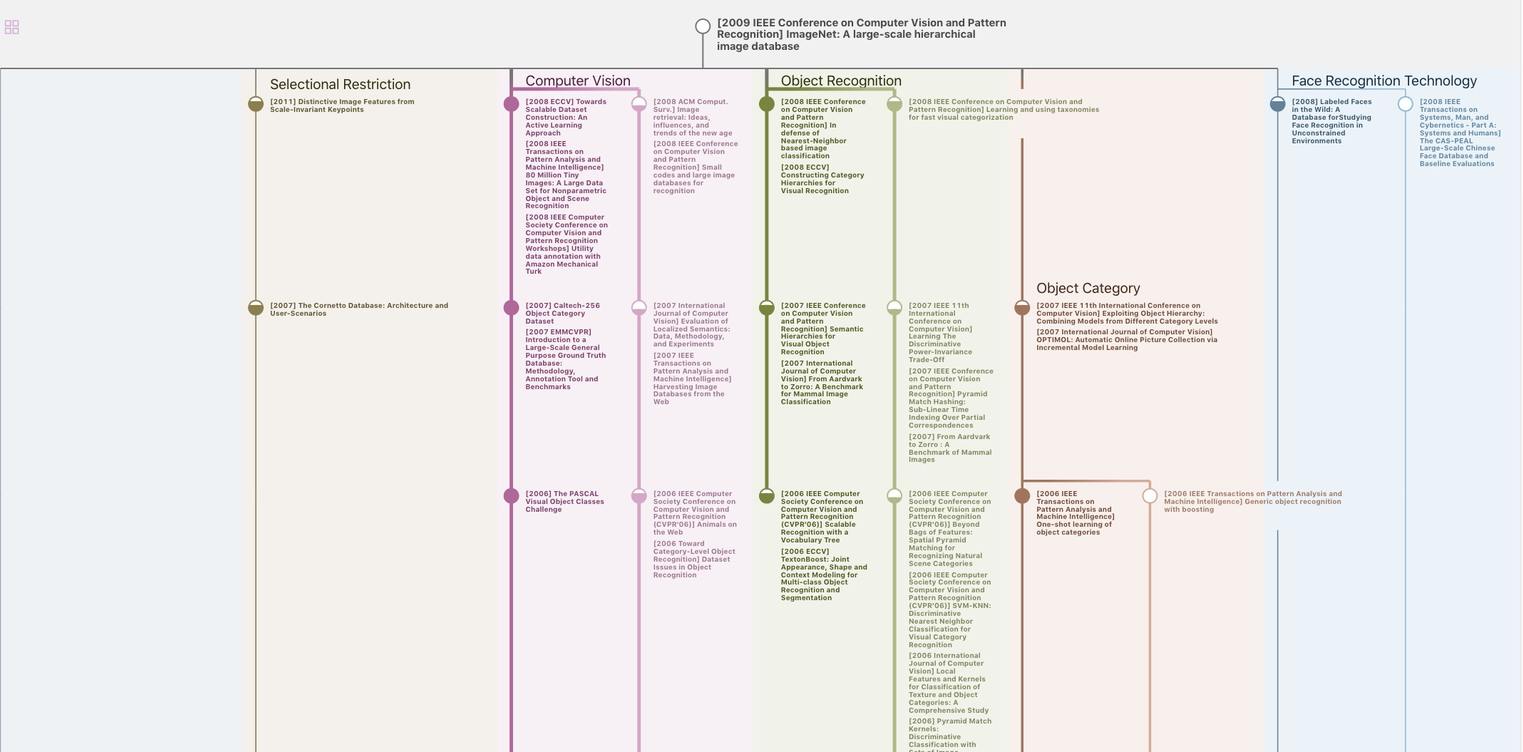
生成溯源树,研究论文发展脉络
Chat Paper
正在生成论文摘要