Model Latent Views With Multi-Center Metric Learning for Vehicle Re-Identification
IEEE Transactions on Intelligent Transportation Systems(2021)
摘要
Multi-view vehicle re-identification (Re-ID) aims to retrieve all images of a target vehicle from a large gallery where the vehicles are captured from non-overlapping cameras. However, the drastic variation in vehicle appearance under different viewpoints greatly affects the performance of the multi-view vehicle Re-ID model, so the key issue in multi-view vehicle Re-ID is learning an effective feature representation that is robust to both dramatic intra-class variability and small inter-class variability. To achieve this goal, we have proposed a multi-center metric learning framework for multi-view vehicle Re-ID. In our approach, we model latent views from vehicle visual appearance directly without any extra labels except ID. Firstly, we introduce several latent view clusters for a vehicle to model latent multi-view information and each view cluster has a learnable center. Then multi-view vehicle matching task can be transformed into two subproblems, cross-view matching and cross-target matching. Finally, an intra-class ranking loss with cross-view center constraint and a cross-class ranking loss with cross-vehicle center constraint are proposed to address the two subproblems, respectively. Extensive experimental evaluations on three widely used benchmarks show the superiority of the proposed framework in contrast to a series of existing state-of-the-arts.
更多查看译文
关键词
Multi-view modeling,multi-center learning,deep metric learning,multi-view vehicle re-identification
AI 理解论文
溯源树
样例
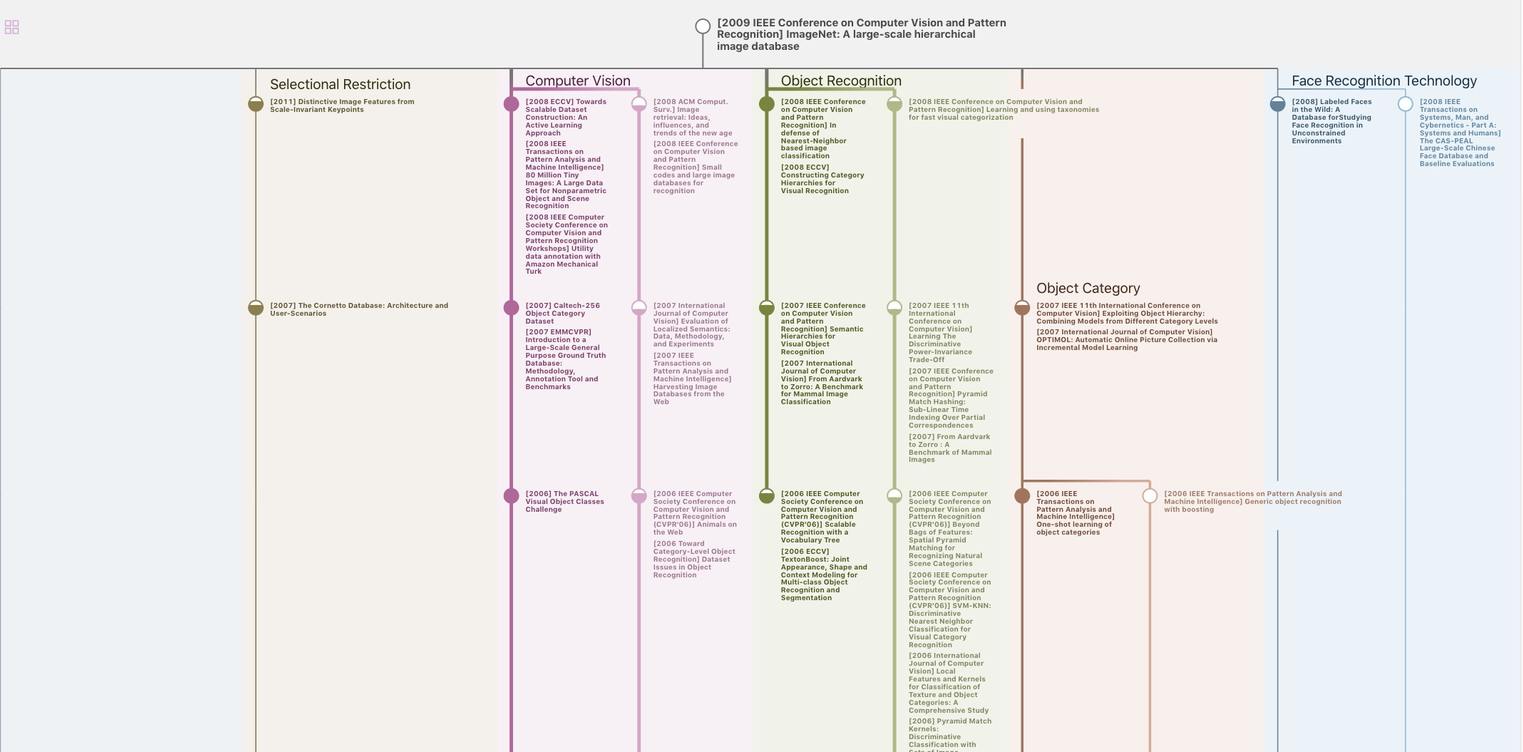
生成溯源树,研究论文发展脉络
Chat Paper
正在生成论文摘要