Quantitative Structure-Plasticity Relationship in Metallic Glass:A Machine Learning Study
Chinese Physics B/Chinese physics B(2021)
摘要
The lack of the long-range order in the atomic structure challenges the identification of the structural defects, akin to dislocations in crystals, which are responsible for predicting plastic events and mechanical failure in metallic glasses(MGs). Although vast structural indicators have been proposed to identify the structural defects, quantitatively gauging the correlations between these proposed indicators based on the undeformed configuration and the plasticity of MGs upon external loads is still lacking. Here, we systematically analyze the ability of these indicators to predict plastic events in a representative MG model using machine learning method. Moreover, we evaluate the influences of coarse graining method and medium-range order on the predictive power. We demonstrate that indicators relevant to the low-frequency vibrational modes reveal the intrinsic structural characteristics of plastic rearrangements. Our work makes an important step towards quantitative assessments of given indicators, and thereby an effective identification of the structural defects in MGs.
更多查看译文
关键词
metallic glass,structure,plasticity,machine learning
AI 理解论文
溯源树
样例
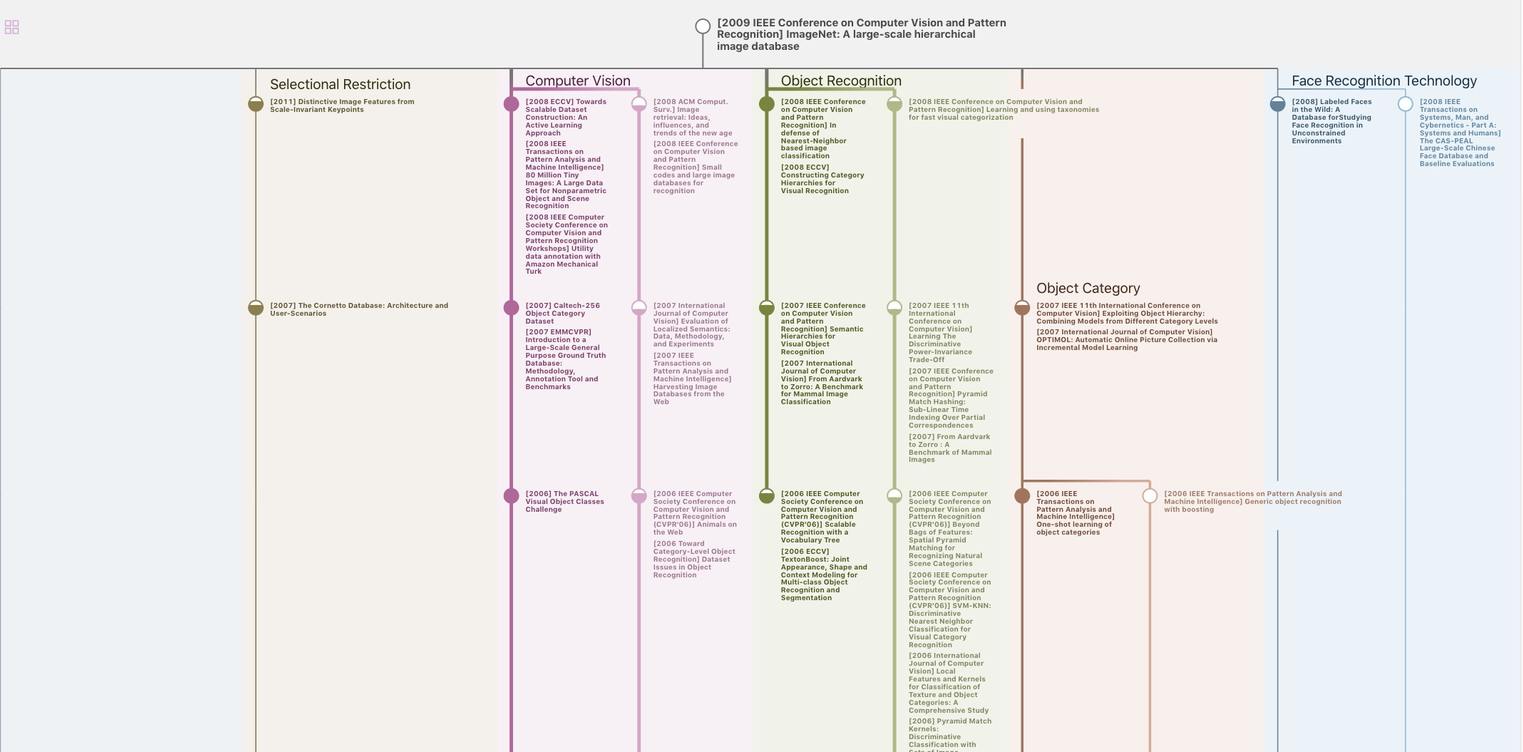
生成溯源树,研究论文发展脉络
Chat Paper
正在生成论文摘要