Power-efficient Spike Sorting Scheme Using Analog Spiking Neural Network Classifier
ACM journal on emerging technologies in computing systems(2021)
摘要
The method to map the neural signals to the neuron from which it originates is spike sorting. A low-power spike sorting system is presented for a neural implant device. The spike sorter constitutes a two-step trainer module that is shared by the signal acquisition channel associated with multiple electrodes. A low-power Spiking Neural Network (SNN) module is responsible for assigning the spike class. The two-step shared supervised on-chip training module is presented for improved training accuracy for the SNN. Post implant, the relatively power-hungry training module can be activated conditionally based on a statistics-driven retraining algorithm that allows on the fly training and adaptation. A low-power analog implementation for the SNN classifier is proposed based on resistive crossbar memory exploiting its approximate computing nature. Owing to the direct mapping of SNN functionality using physical characteristics of devices, the analog mode implementation can achieve ∼21 × lower power than its fully digital counterpart. We also incorporate the effect of device variation in the training process to suppress the impact of inevitable inaccuracies in such resistive crossbar devices on the classification accuracy. A variation-aware, digitally calibrated analog front-end is also presented, which consumes less than ∼50 nW power and interfaces with the digital training module as well as the analog SNN spike sorting module. Hence, the proposed scheme is a low-power, variation-tolerant, adaptive, digitally trained, all-analog spike sorter device, applicable to implantable and wearable multichannel brain-machine interfaces.
更多查看译文
关键词
Adaptation,neuromorphic computing,resistive crossbar network (RCN),spiking neural network (SNN),spike sorting
AI 理解论文
溯源树
样例
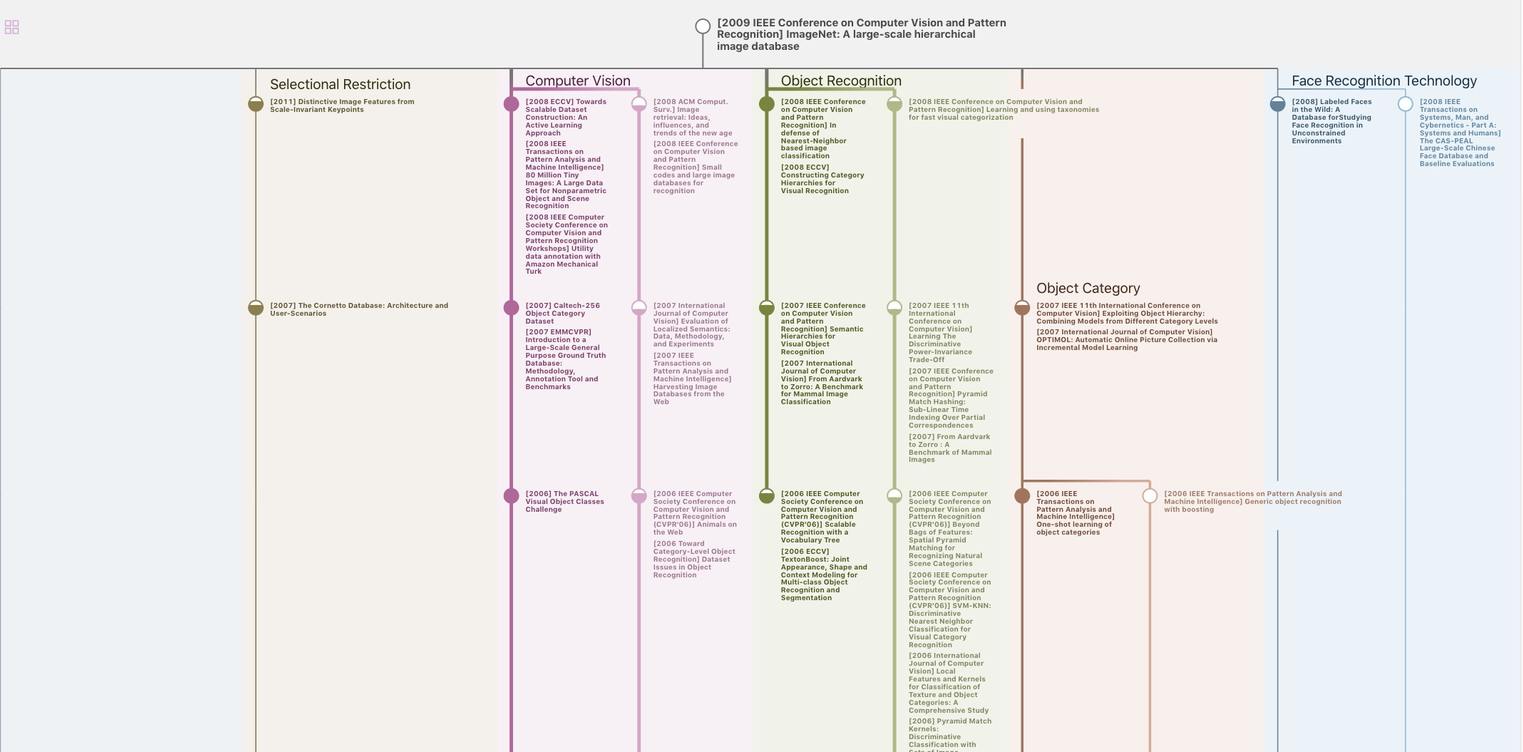
生成溯源树,研究论文发展脉络
Chat Paper
正在生成论文摘要