Automated Crack Severity Level Detection And Classification For Ballastless Track Slab Using Deep Convolutional Neural Network
AUTOMATION IN CONSTRUCTION(2021)
摘要
The classification and treatment of cracks with different severity levels based on the width measurement is a critical consideration in maintenance of ballastless track slab. Existing deep learning methods cannot directly quantify cracks, which must rely on image processing technologies to post-process the initial results by deep learning, inevitably leading to multiple steps and low efficiency. This paper proposes a novel quantitative classification method for cracks with different severity levels based on deep convolutional neural networks, using orthogonal projection method to preprocess training data and define the severity level, which is validated and evaluated from four aspects: network structures, crack data, classification methods, and environmental conditions. Results show that the Inception-ResNet-v2 network can classify crack images into three severity levels without pixel segmentation or post-processing, achieving the accuracy, precision, recall and F1 score all exceeding 93%, with good robustness and adaptability to noise and light intensity.
更多查看译文
关键词
Deep convolutional neural network, Automated crack classification, Ballastless track slab, Image processing technology, Severity level quantification
AI 理解论文
溯源树
样例
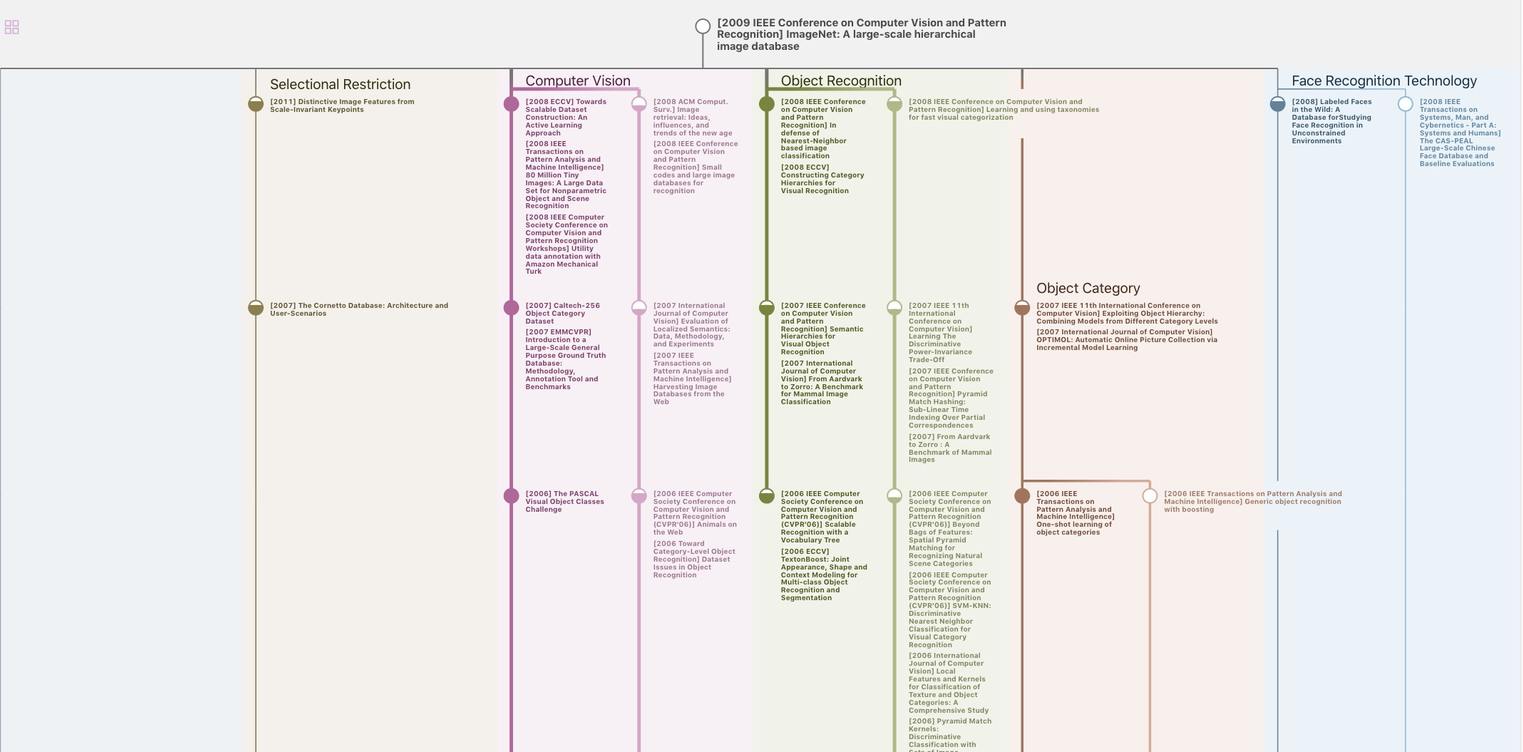
生成溯源树,研究论文发展脉络
Chat Paper
正在生成论文摘要