Learning Catalyst Design Based On Bias-Free Data Set For Oxidative Coupling Of Methane
ACS CATALYSIS(2021)
摘要
Combinatorial catalyst design is hardly generalizable, and the empirical aspect of the research has biased the literature data toward accidentally found combinations. Here, 300 quaternary solid catalysts are randomly sampled from a materials space consisting of 36,540 catalysts, and their performance in the oxidative coupling of methane is evaluated by a high-throughput screening instrument. The obtained bias-free data set is analyzed to withdraw catalyst design guidelines. Even with random sampling, 51 catalysts out of the 300 provide a C-2 yield sufficiently superior to the noncatalytic free radical process. Data analysis suggests the significance of choosing synergistic combinations, and such combinations could be generalized based on the group in the periodic table. Decision tree classification is successfully implemented to facilitate efficient sampling of quaternary catalysts toward a better C-2 yield.
更多查看译文
关键词
bias free data set, high-throughput screening, catalyst informatics, oxidative coupling of methane, machine learning
AI 理解论文
溯源树
样例
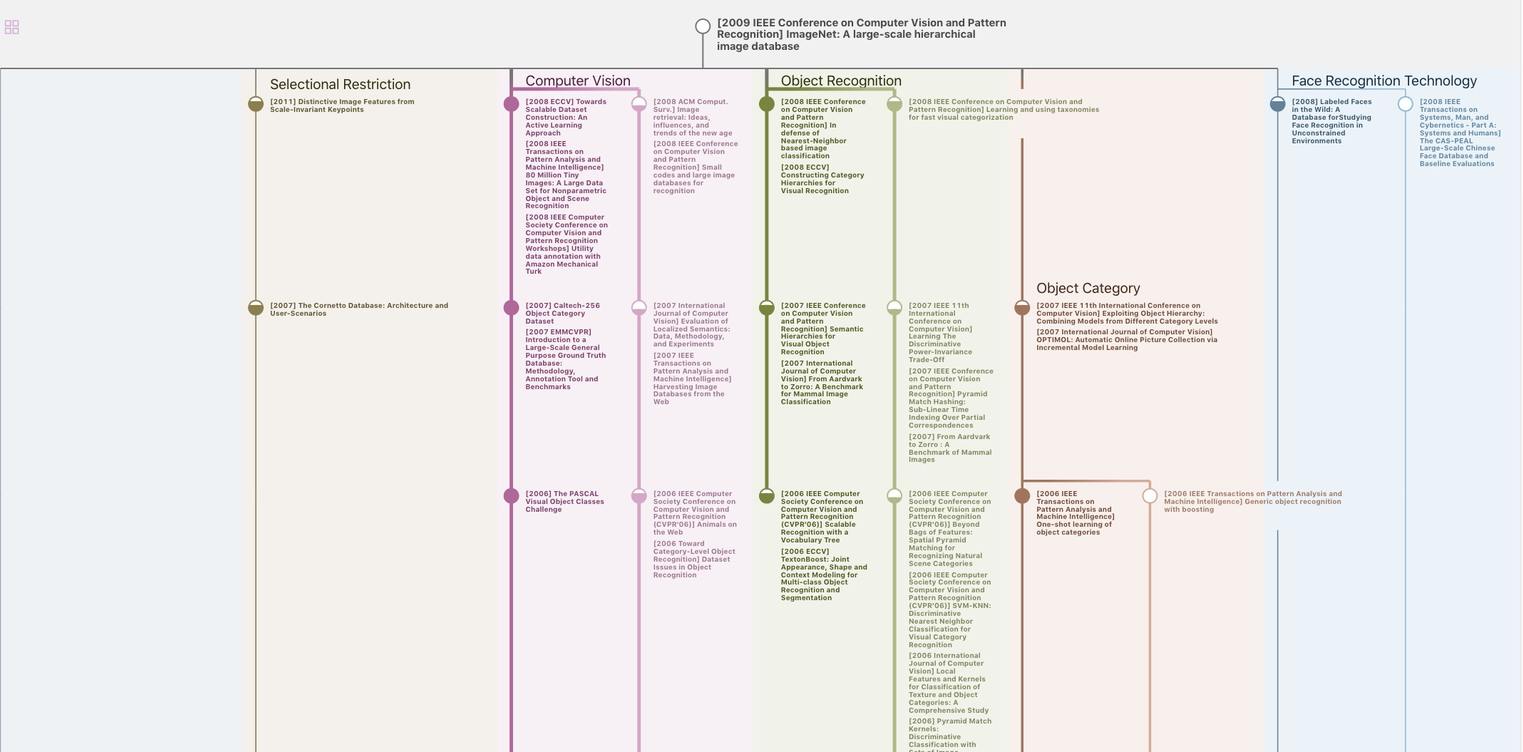
生成溯源树,研究论文发展脉络
Chat Paper
正在生成论文摘要