A Transfer Learning Method For Meteorological Visibility Estimation Based On Feature Fusion Method
APPLIED SCIENCES-BASEL(2021)
摘要
Featured ApplicationThe method in this paper aims to select effective subregions, reduce the amount of calculation and maintain the accuracy of visibility estimation.Meteorological visibility is an important meteorological observation indicator to measure the weather transparency which is important for the transport safety. It is a challenging problem to estimate the visibilities accurately from the image characteristics. This paper proposes a transfer learning method for the meteorological visibility estimation based on image feature fusion. Different from the existing methods, the proposed method estimates the visibility based on the data processing and features' extraction in the selected subregions of the whole image and therefore it had less computation load and higher efficiency. All the database images were gray-averaged firstly for the selection of effective subregions and features extraction. Effective subregions are extracted for static landmark objects which can provide useful information for visibility estimation. Four different feature extraction methods (Densest, ResNet50, Vgg16, and Vgg19) were used for the feature extraction of the subregions. The features extracted by the neural network were then imported into the proposed support vector regression (SVR) regression model, which derives the estimated visibilities of the subregions. Finally, based on the weight fusion of the visibility estimates from the subregion models, an overall comprehensive visibility was estimated for the whole image. Experimental results show that the visibility estimation accuracy is more than 90%. This method can estimate the visibility of the image, with high robustness and effectiveness.
更多查看译文
关键词
visibility evaluation, feature extraction, gray average, feature fusion, transfer learning
AI 理解论文
溯源树
样例
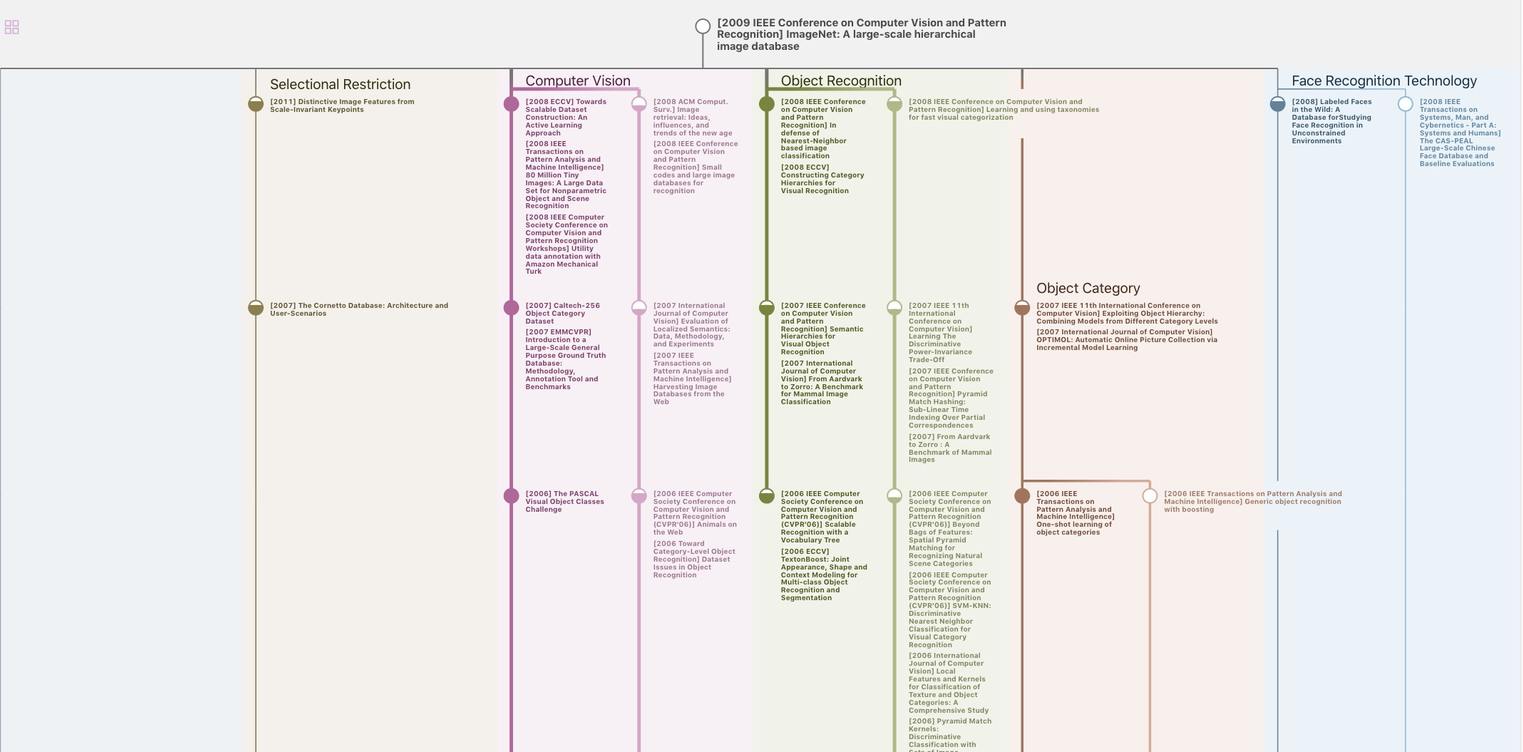
生成溯源树,研究论文发展脉络
Chat Paper
正在生成论文摘要