Speech Enhancement Using Multi-Stage Self-Attentive Temporal Convolutional Networks
IEEE-ACM TRANSACTIONS ON AUDIO SPEECH AND LANGUAGE PROCESSING(2021)
摘要
Multi-stage learning is an effective technique for invoking multiple deep-learning modules sequentially. This paper applies multi-stage learning to speech enhancement by using a multi-stage structure, where each stage comprises a self-attention (SA) block followed by stacks of temporal convolutional network (TCN) blocks with doubling dilation factors. Each stage generates a prediction that is refined in a subsequent stage. A feature fusion block is inserted at the input of later stages to re-inject original information. The resulting multi-stage speech enhancement system, multi-stage SA-TCN, is compared with state-of-the-art deep-learning speech enhancement methods using the LibriSpeech and VCTK datasets. The multi-stage SA-TCN system's hyperparameters are fine-tuned, and the impact of the SA block, the feature fusion block, and the number of stages are determined. The use of a multi-stage SA-TCN system as a front-end for automatic speech recognition systems is also investigated. It is shown that the multi-stage SA-TCN systems perform well relative to other state-of-the-art systems in terms of speech enhancement and speech recognition scores.
更多查看译文
关键词
Speech enhancement, Convolution, Speech recognition, Task analysis, Noise measurement, Spectrogram, Recurrent neural networks, Speech enhancement, speech recognition, neural networks, self-attention, temporal convolutional networks, multi-stage architectures
AI 理解论文
溯源树
样例
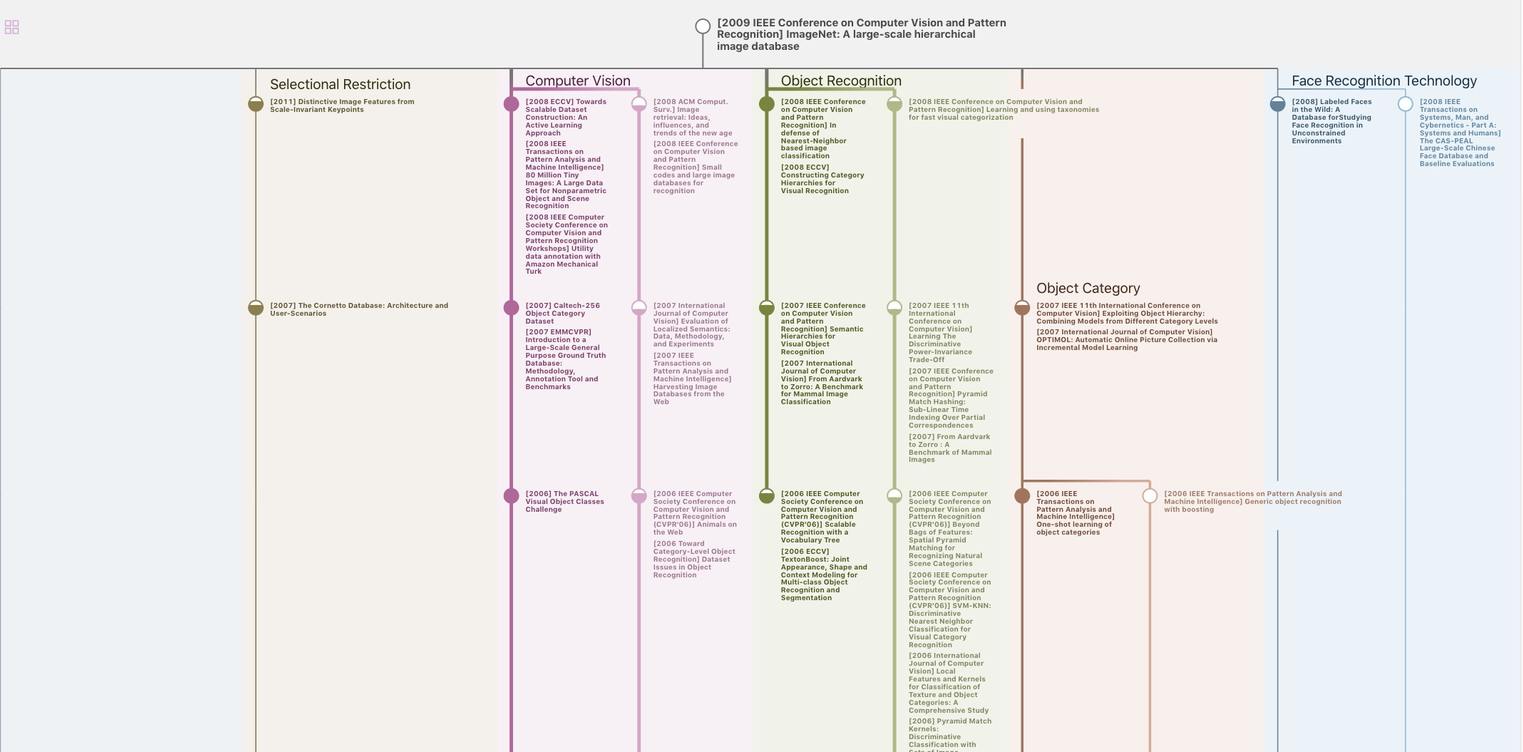
生成溯源树,研究论文发展脉络
Chat Paper
正在生成论文摘要