A Simulation-based End-to-End Learning Framework for Evidential Occupancy Grid Mapping
2021 32ND IEEE INTELLIGENT VEHICLES SYMPOSIUM (IV)(2021)
摘要
Evidential occupancy grid maps (OGMs) are a popular representation of the environment of automated vehicles. Inverse sensor models (ISMs) are used to compute OGMs from sensor data such as lidar point clouds. Geometric ISMs show a limited performance when estimating states in unobserved but inferable areas and have difficulties dealing with ambiguous input. Deep learning-based ISMs face the challenge of limited training data and they often cannot handle uncertainty quantification yet. We propose a deep learning-based framework for learning an OGM algorithm which is both capable of quantifying first- and second-order uncertainty and which does not rely on manually labeled data. Results on synthetic and on real-world data show superiority over other approaches. Source code and datasets are available at https://github.com/ika-rwth-aschen/EviLOG.
更多查看译文
关键词
evidential occupancy grid mapping,simulation-based,end-to-end
AI 理解论文
溯源树
样例
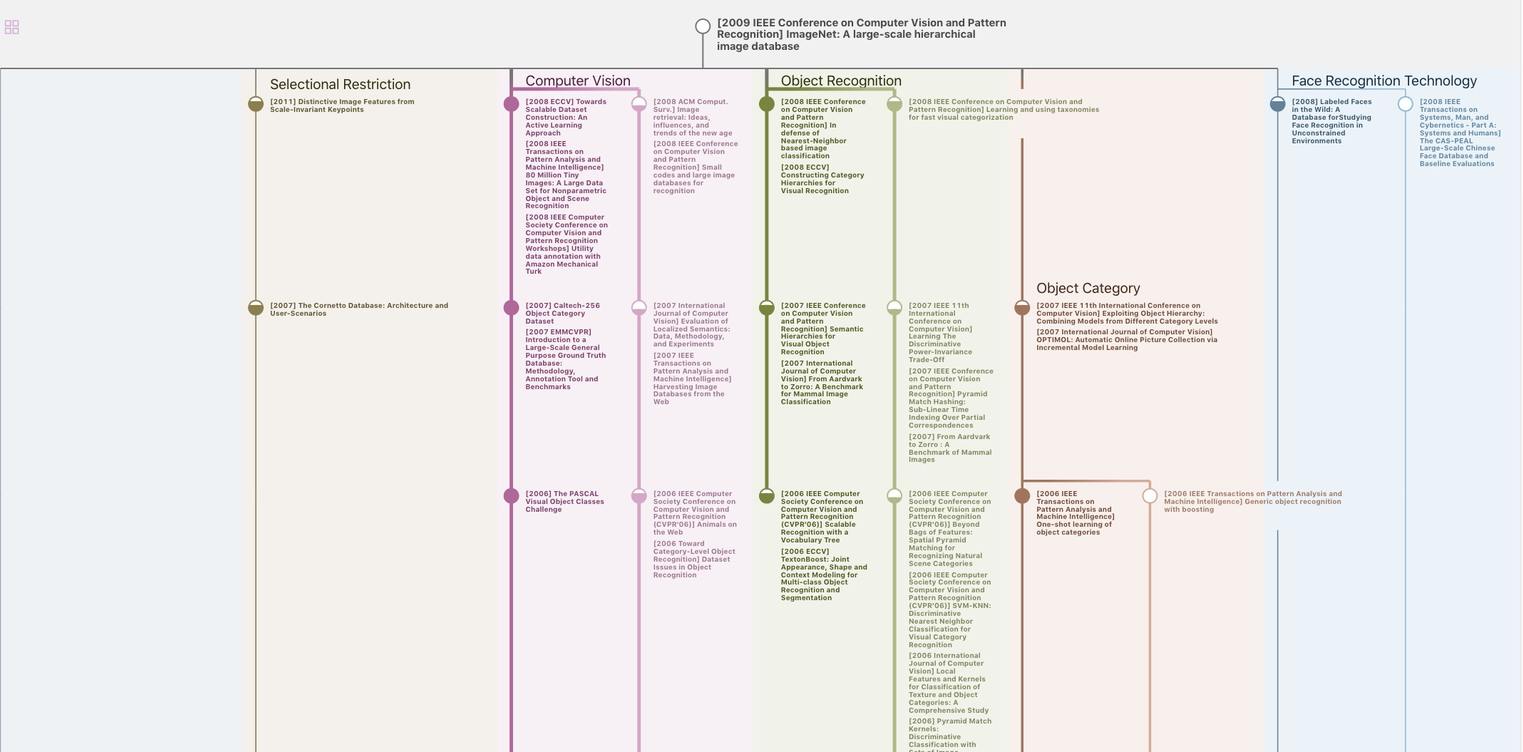
生成溯源树,研究论文发展脉络
Chat Paper
正在生成论文摘要