Binary segmentation of medical images using implicit spline representations and deep learning
Computer Aided Geometric Design(2021)
摘要
We propose a novel approach to image segmentation based on combining implicit spline representations with deep convolutional neural networks. This is done by predicting the control points of a bivariate spline function whose zero-set represents the segmentation boundary. We adapt several existing neural network architectures and design novel loss functions that are tailored towards providing implicit spline curve approximations. The method is evaluated on a congenital heart disease computed tomography medical imaging dataset. Experiments are carried out by measuring performance in various standard metrics for different networks and loss functions. We determine that splines of bidegree (1,1) with 128×128 coefficient resolution performed optimally for 512×512 resolution CT images. For our best network, we achieve an average volumetric test Dice score of close to 92%, which reaches the state of the art for this congenital heart disease dataset.
更多查看译文
关键词
Implicit spline representations,Shape modelling,Deep learning,Medical imaging,Image segmentation
AI 理解论文
溯源树
样例
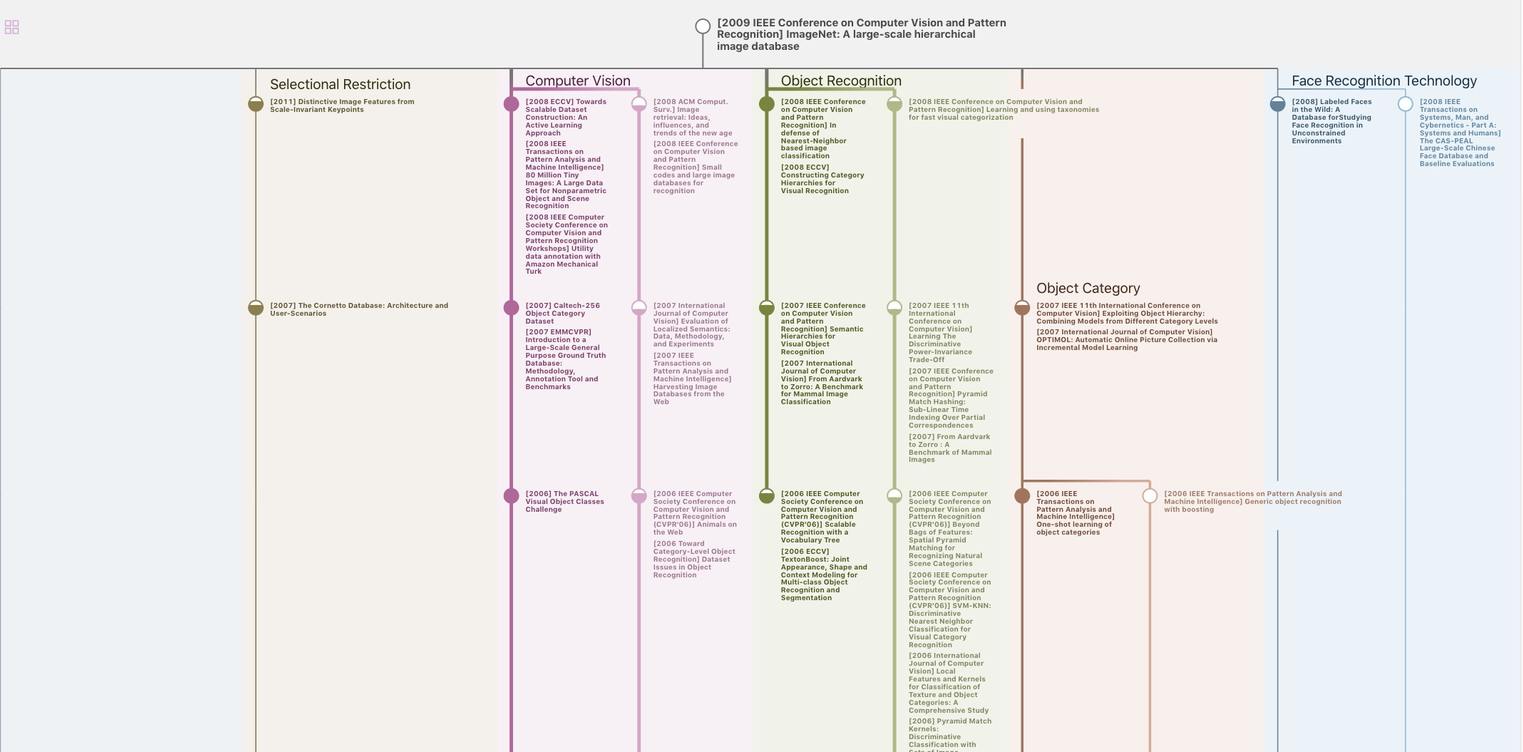
生成溯源树,研究论文发展脉络
Chat Paper
正在生成论文摘要