Space-Address Decoupled Scratchpad Memory Management For Neural Network Accelerators
CONCURRENCY AND COMPUTATION-PRACTICE & EXPERIENCE(2021)
摘要
Deep neural networks have been demonstrated to be useful in varieties of intelligent tasks, and various specialized NN accelerators have been proposed recently to improve the hardware efficiency, which are typically equipped with software-managed scratchpad memory (SPM) for high performance and energy efficiency. However, traditional SPM management techniques cause memory fragmentation for NN accelerators, and thus lead to low utilization of precious SPM. The main reason is that traditional techniques are originally designed for managingfixed-length registersrather thanvariable-length memory blocks. In this article, we propose a novel SPM management approach for NN accelerators. The basic intuition is that NN computation/memory behaviors are predictable and relatively regular compared with traditional applications, and thus most information can be determined at compile time. In addition, by exploiting the variable-length feature of SPM, we propose to divide the allocation process into two passes: thespace assignmentand theaddress assignmentpass, which are simultaneously (and implicitly) performed in traditional one-pass allocation techniques. Experimental results on the memory requests of a representative NN accelerator demonstrate that the proposed approach can significantly reduce the memory consumption by 30% at most compared with state-of-the-art SPM management techniques, and the memory usage is only 2% larger than that of the theoretical optimal allocation.
更多查看译文
关键词
deep neural network, memory management, scratchpad memory
AI 理解论文
溯源树
样例
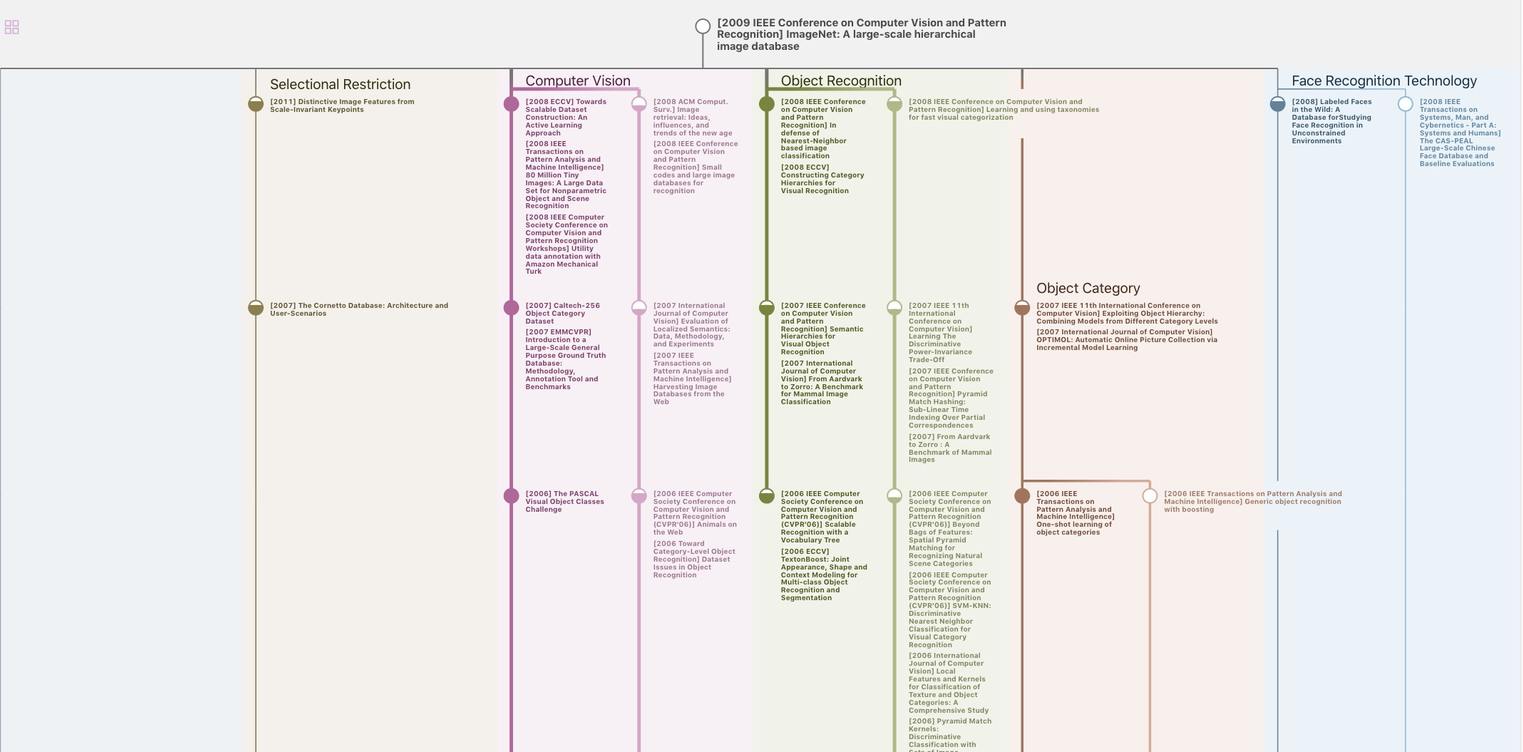
生成溯源树,研究论文发展脉络
Chat Paper
正在生成论文摘要