Evolving Attention with Residual Convolutions
INTERNATIONAL CONFERENCE ON MACHINE LEARNING, VOL 139(2021)
摘要
Transformer is a ubiquitous model for natural language processing and has attracted wide attentions in computer vision. The attention maps are indispensable for a transformer model to encode the dependencies among input tokens. However, they are learned without explicit interactions in each layer and sometimes fail to capture reasonable patterns. In this paper, we propose a novel and generic mechanism based on evolving attention try improve the performance of transformers. On one hand, the attention maps in different layers share common knowledge, thus the ones in preceding layers can instruct the learning of attention in succeeding layers through residual connections. On the other hand, low-level and high-level attentions vary in the levels of abstraction, so we adopt additional convolutional layers to capture the evolutionary process of attention maps. The proposed evolving attention mechanism achieves significant performance improvement over various state-of-the-art models for multiple tasks, including image classification, natural language understanding and machine translation.
更多查看译文
AI 理解论文
溯源树
样例
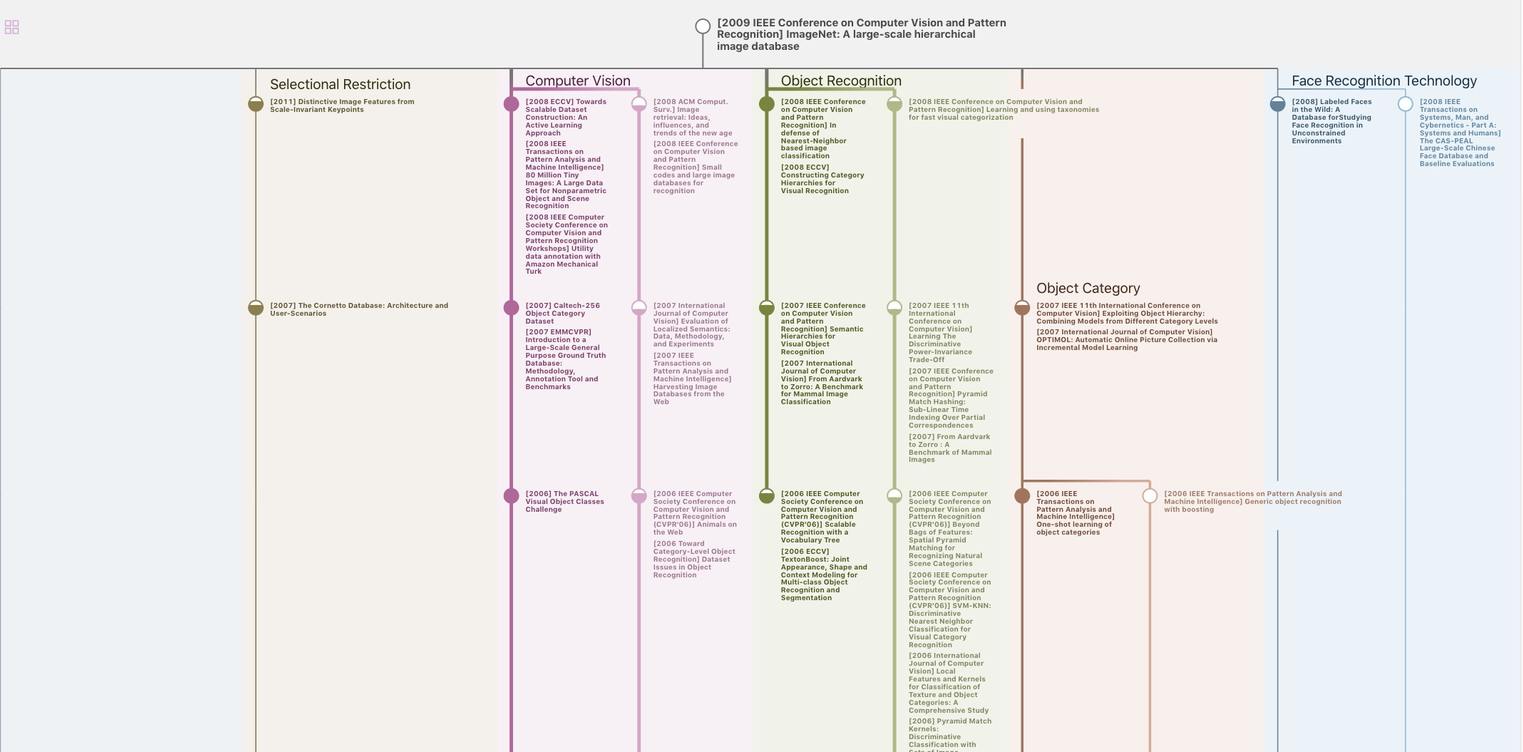
生成溯源树,研究论文发展脉络
Chat Paper
正在生成论文摘要